Smart scheduling of hanging workshop via digital twin and deep reinforcement learning
Flexible Services and Manufacturing Journal(2024)
摘要
The complexity of job-shop environments poses significant challenges for effective scheduling in the manufacturing industry. Flexible job-shop scheduling, in particular, involves coordinating multiple jobs with varying requirements across multiple machines, while considering various constraints and objectives. Traditional scheduling approaches often struggle to handle the dynamic nature inherent in such environments. To address the above challenges, this paper presents a digital twin (DT)-based management framework for hanging workshops, aiming to enhance flexible job-shop scheduling in manufacturing environments. The framework integrates real-time monitoring, condition monitoring, and smart scheduling capabilities to improve overall performance and efficiency. To implement the framework, a smart scheduling methodology based on a graph neural network and deep reinforcement learning (DRL) is devised. Markov Decision Process (MDP) captures the dynamic nature and uncertainties of the hanging workshop, while the graph embedding network technique represents operations, machines, and their relationships in a low-dimensional space. Decision-making is enhanced through training with the Proximal Policy Optimization (PPO) algorithm. Extensive Experiments are conducted to evaluate the framework’s effectiveness. The findings demonstrate the ability of the framework to improve job-shop scheduling performance.
更多查看译文
关键词
Hanging workshop,Flexible job shop problem,Deep reinforcement learning,Graph embedding,Digital twin
AI 理解论文
溯源树
样例
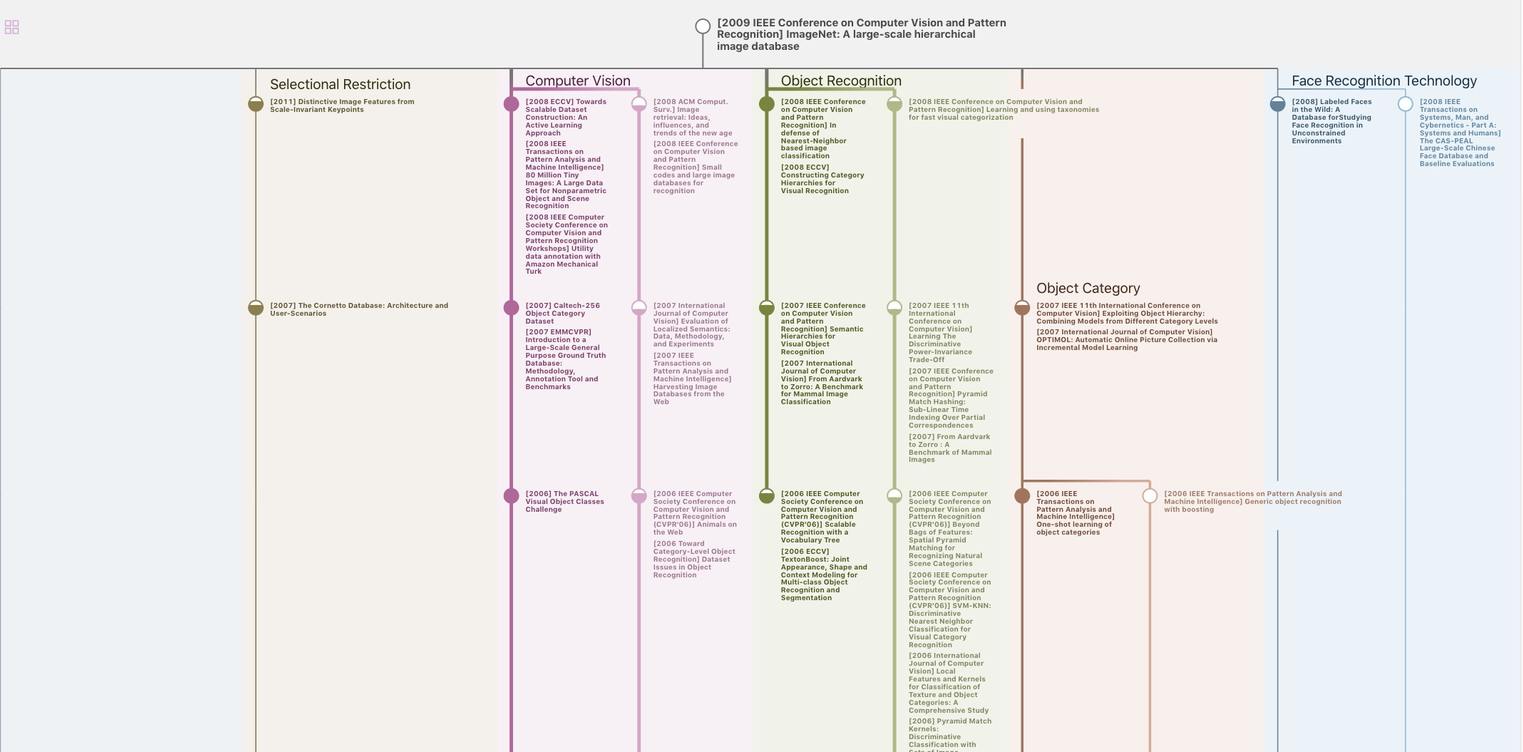
生成溯源树,研究论文发展脉络
Chat Paper
正在生成论文摘要