Chlorophyll-α forecasting using LSTM, bidirectional LSTM and GRU networks in El Mar Menor (Spain)
LOGIC JOURNAL OF THE IGPL(2024)
Abstract
The objective of this research is to develop accurate forecasting models for chlorophyll-alpha concentrations at various depths in El Mar Menor, Spain. Chlorophyll-alpha plays a crucial role in assessing eutrophication in this vulnerable ecosystem. To achieve this objective, various deep learning forecasting techniques, including long short-term memory, bidirectional long short-term memory and gated recurrent uni networks, were utilized. The models were designed to forecast the chlorophyll-alpha levels with a 2-week prediction horizon. To enhance the models' accuracy, a sliding window method combined with a blocked cross-validation procedure for time series was also applied to these techniques. Two input strategies were also tested in this approach: using only chlorophyll-alpha time series and incorporating exogenous variables. The proposed approach significantly improved the accuracy of the predictive models, no matter the forecasting technique employed. Results were remarkable, with $\overline{\sigma}$ values reaching approximately 0.90 for the 0.5-m depth level and 0.80 for deeper levels. The proposed forecasting models and methodologies have great potential for predicting eutrophication episodes and acting as decision-making tools for environmental agencies. Accurate prediction of eutrophication episodes through these models could allow for proactive measures to be implemented, resulting in improved environmental management and the preservation of the ecosystem.
MoreTranslated text
Key words
chlorophyll,machine learning,deep learning,LSTM,Mar Menor,eutrophication,GRU
AI Read Science
Must-Reading Tree
Example
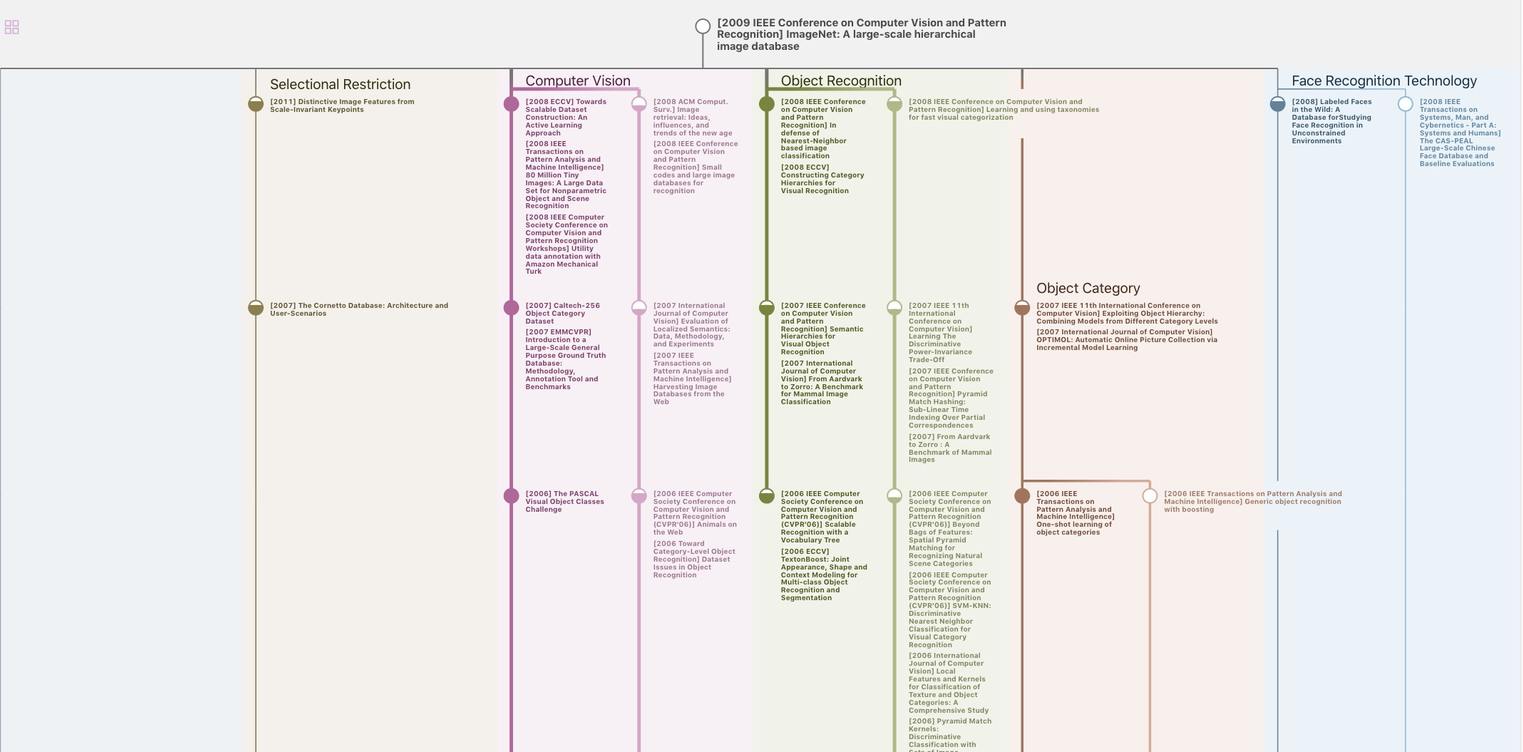
Generate MRT to find the research sequence of this paper
Chat Paper
Summary is being generated by the instructions you defined