Serum and Urine Metabolites and Kidney Function.
Journal of the American Society of Nephrology(2024)
Abstract
Background: Metabolites represent a read-out of cellular processes underlying states of health and disease. Methods: We evaluated cross-sectional and longitudinal associations between 1255 serum and 1398 urine known and unknown (denoted with "X" in name) metabolites (Metabolon HD4, 721 detected in both biofluids) and kidney function in 1612 participants of the Atherosclerosis Risk in Communities (ARIC) Study. All analyses were adjusted for clinical and demographic covariates, including for baseline eGFR and UACR in longitudinal analyses. Results:At visit 5 of the ARIC study, the mean age of participants was 76 years (SD 6), 56% were women, mean eGFR was 62 ml/min/1.73m2 (SD 20), and median urine albumin-to-creatinine level (UACR) was 13 mg/g (IQR 25). In cross-sectional analysis, 675 serum and 542 urine metabolites were associated with eGFR (Bonferroni-corrected p < 4.0E-5 for serum analyses and p < 3.6E-5 for urine analyses), including 248 metabolites shared across biofluids. Fewer metabolites (75 serum and 91 urine metabolites, including 7 shared across biofluids) were cross-sectionally associated with albuminuria. Guanidinosuccinate, N2,N2-dimethylguanosine, hydroxy-N6,N6,N6-trimethyllysine, X-13844, and X-25422 were significantly associated with both eGFR and albuminuria. Over a mean follow-up of 6.6 years, serum mannose (HR 2.3 [1.6,3.2], p = 2.7E-5) and urine X-12117 (HR 1.7 [1.3,2.2], p = 1.9E-5) were risk factors for UACR doubling, whereas urine sebacate (HR 0.86 [0.80,0.92], p = 1.9E-5) was inversely associated. Compared to clinical characteristics alone, including the top 5 endogenous metabolites in serum and urine associated with longitudinal outcomes improved the outcome prediction (AUCs for eGFR decline: clinical model = 0.79, clinical + metabolites model = 0.87, p = 8.1E-6; for UACR doubling: clinical model = 0.66, clinical + metabolites model = 0.73, p = 2.9E-5). Conclusions: Metabolomic profiling in different biofluids provided distinct and potentially complementary insights into the biology and prognosis of kidney diseases.
MoreTranslated text
AI Read Science
Must-Reading Tree
Example
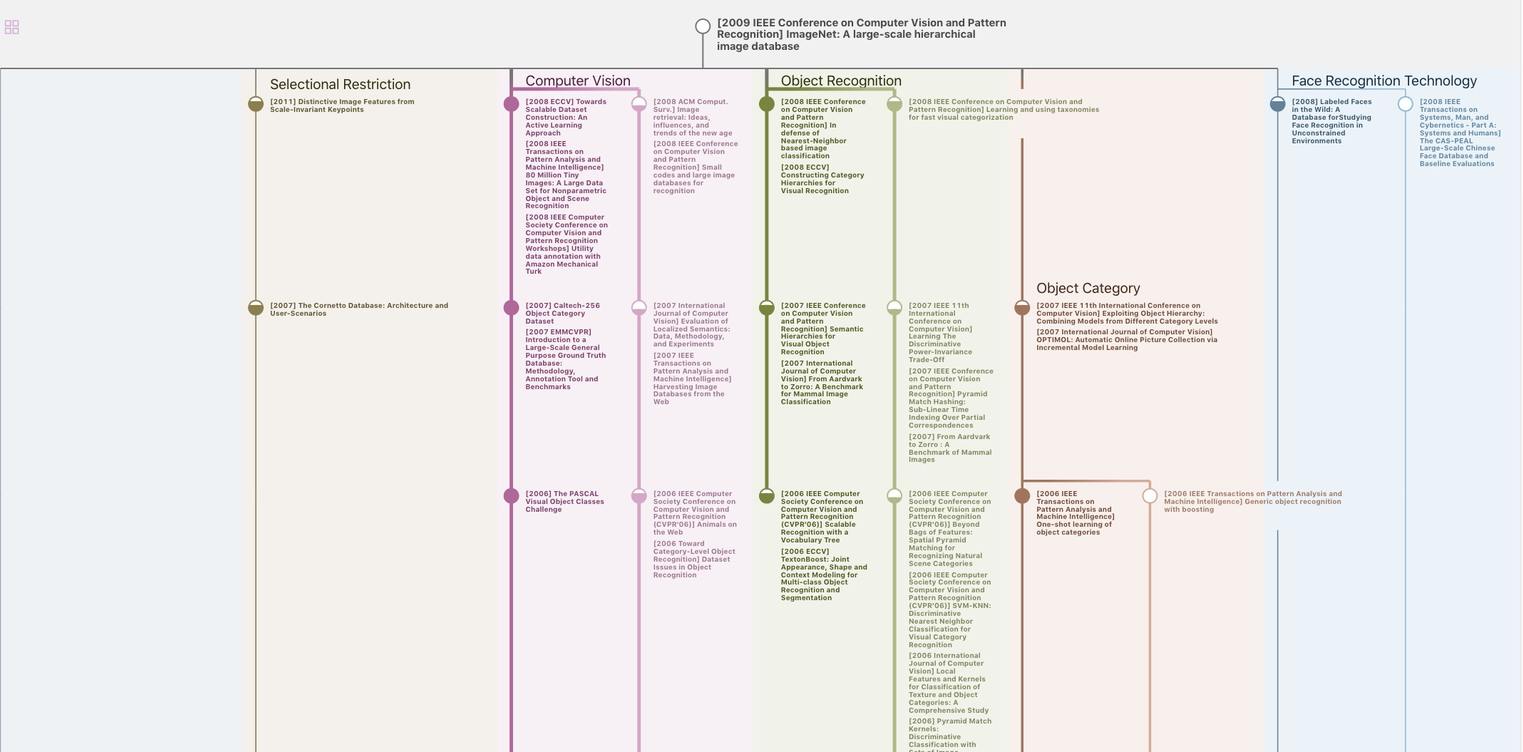
Generate MRT to find the research sequence of this paper
Chat Paper
Summary is being generated by the instructions you defined