PMA-Net: Progressive multi-stage adaptive feature learning for two-view correspondence
Knowledge-Based Systems(2024)
Abstract
Establishing high-quality correspondences is a fundamental step for many computer vision tasks. Leveraging the local consistency of correct correspondences (i.e., inliers) has been a prevalent approach to distinguish them from incorrect correspondences (i.e., outliers). However, the random distribution of a significant proportion of outliers complicates the local neighborhood construction of inliers, making it inevitably contain many outliers, which compromises the reliability of the consistency information. In this paper, we propose a Progressive Multi-Stage Adaptive Feature Learning Network (PMA-Net) to solve this problem, which progressively and adaptively learns over multiple stages to remove random outliers embedded in the initial consistency. Specifically, we propose an Adaptive Dynamic Graph Construction (ADGC) module to construct a global topological graph with a high inlier ratio by calculating the affinity between the corresponding neighbors. Additionally, we also design a Feature Mapping Processing (FMP) block and Multi-Stage Prediction (MSP) block to improve the accuracy of subsequent local neighborhood information aggregation, as well as information loss due to channel dimension compression in the prediction phase. Experimental results in camera pose estimation, remote sensing image registration, and homography estimation demonstrate that the proposed PMA-Net performs better than other state-of-the-art methods on various public datasets. Code: https://github.com/XiaojieLi11/PMA-Net
MoreTranslated text
Key words
Feature matching,Outlier removal,Pose estimation,Local neighbor,Two-view correspondence
AI Read Science
Must-Reading Tree
Example
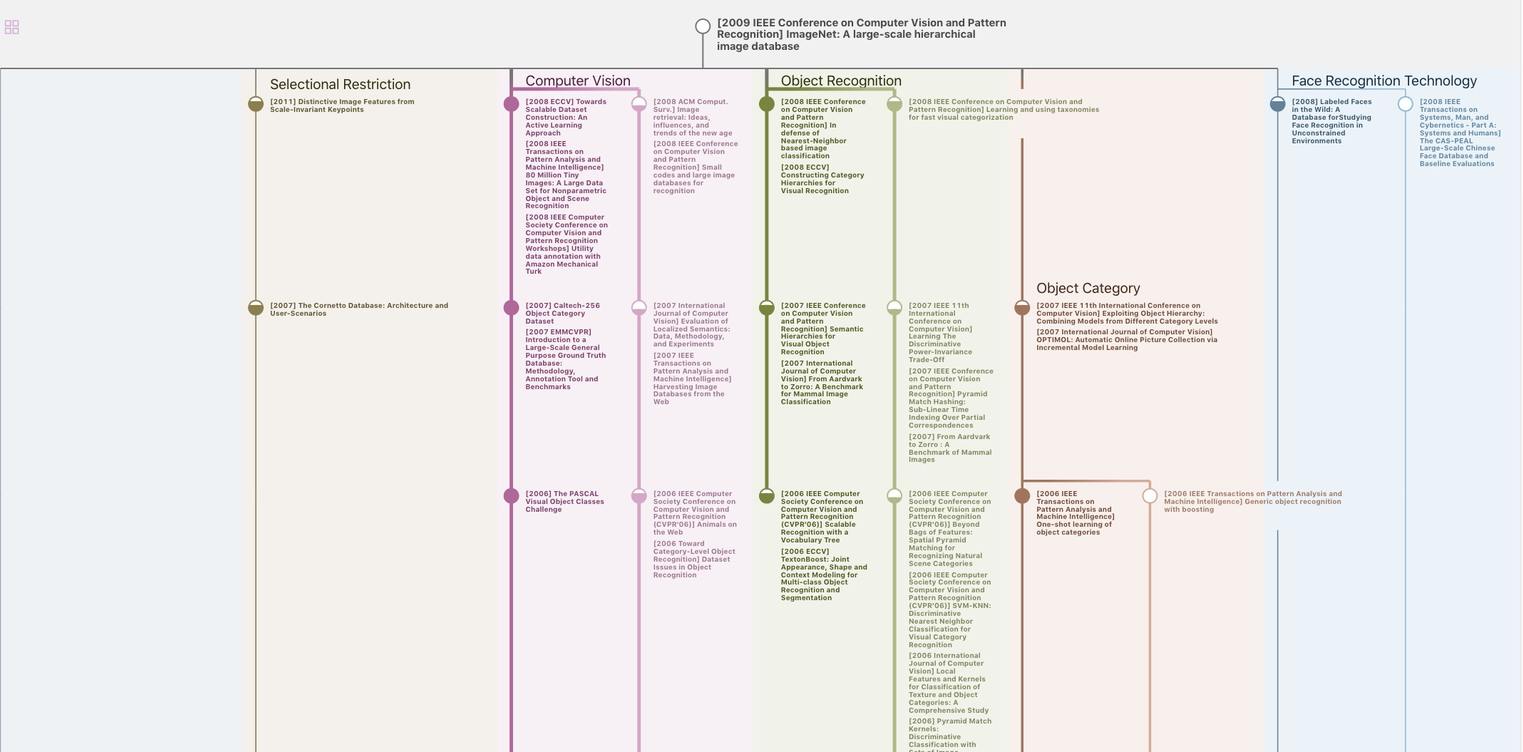
Generate MRT to find the research sequence of this paper
Chat Paper
Summary is being generated by the instructions you defined