Machine learning and radiomics analysis by computed tomography in colorectal liver metastases patients for RAS mutational status prediction
La radiologia medica(2024)
摘要
To assess the efficacy of machine learning and radiomics analysis by computed tomography (CT) in presurgical setting, to predict RAS mutational status in colorectal liver metastases. Patient selection in a retrospective study was carried out from January 2018 to May 2021 considering the following inclusion criteria: patients subjected to surgical resection for liver metastases; proven pathological liver metastases; patients subjected to enhanced CT examination in the presurgical setting with a good quality of images; and RAS assessment as standard reference. A total of 851 radiomics features were extracted using the PyRadiomics Python package from the Slicer 3D image computing platform after slice-by-slice segmentation on CT portal phase by two expert radiologists of each individual liver metastasis performed first independently by the individual reader and then in consensus. Balancing technique was performed, and inter- and intraclass correlation coefficients were calculated to assess the between-observer and within-observer reproducibility of features. Receiver operating characteristics (ROC) analysis with the calculation of area under the ROC curve (AUC), sensitivity (SENS), specificity (SPEC), positive predictive value (PPV), negative predictive value (NPV) and accuracy (ACC) were assessed for each parameter. Linear and non-logistic regression model (LRM and NLRM) and different machine learning-based classifiers were considered. Moreover, features selection was performed before and after a normalized procedure using two different methods (3-sigma and z-score). Seventy-seven liver metastases in 28 patients with a mean age of 60 years (range 40–80 years) were analyzed. The best predictors, at univariate analysis for both normalized procedures, were original_shape_Maximum2DDiameter and wavelet_HLL_glcm_InverseVariance that reached an accuracy of 80
更多查看译文
关键词
Radiomic analysis,Machine learning,Liver metastases,Computed tomography,RAS mutational status
AI 理解论文
溯源树
样例
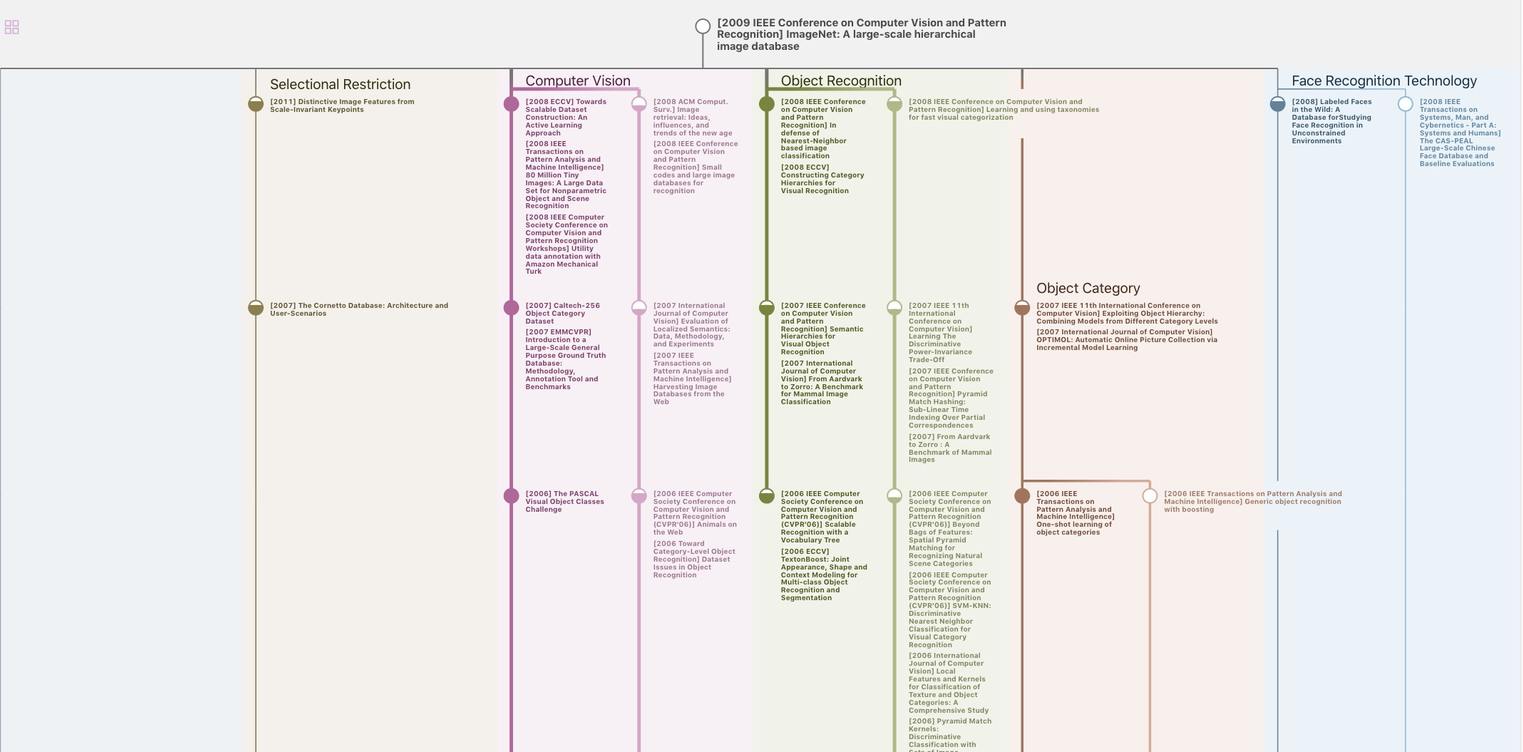
生成溯源树,研究论文发展脉络
Chat Paper
正在生成论文摘要