Development of clinical tools to estimate the breathing effort during high-flow oxygen therapy: A multicenter cohort study
Pulmonology(2024)
摘要
Introduction and objectives
Quantifying breathing effort in non-intubated patients is important but difficult. We aimed to develop two models to estimate it in patients treated with high-flow oxygen therapy.
Patients and Methods
We analyzed the data of 260 patients from previous studies who received high-flow oxygen therapy. Their breathing effort was measured as the maximal deflection of esophageal pressure (ΔPes). We developed a multivariable linear regression model to estimate ΔPes (in cmH2O) and a multivariable logistic regression model to predict the risk of ΔPes being >10 cmH2O. Candidate predictors included age, sex, diagnosis of the coronavirus disease 2019 (COVID-19), respiratory rate, heart rate, mean arterial pressure, the results of arterial blood gas analysis, including base excess concentration (BEa) and the ratio of arterial tension to the inspiratory fraction of oxygen (PaO2:FiO2), and the product term between COVID-19 and PaO2:FiO2.
Results
We found that ΔPes can be estimated from the presence or absence of COVID-19, BEa, respiratory rate, PaO2:FiO2, and the product term between COVID-19 and PaO2:FiO2. The adjusted R2 was 0.39. The risk of ΔPes being >10 cmH2O can be predicted from BEa, respiratory rate, and PaO2:FiO2. The area under the receiver operating characteristic curve was 0.79 (0.73–0.85). We called these two models BREF, where BREF stands for BReathing EFfort and the three common predictors: BEa (B), respiratory rate (RE), and PaO2:FiO2 (F).
Conclusions
We developed two models to estimate the breathing effort of patients on high-flow oxygen therapy. Our initial findings are promising and suggest that these models merit further evaluation.
更多查看译文
关键词
Esophageal pressure,Breathing effort,High-flow oxygen therapy,Acute respiratory failure,Prediction model,BREF
AI 理解论文
溯源树
样例
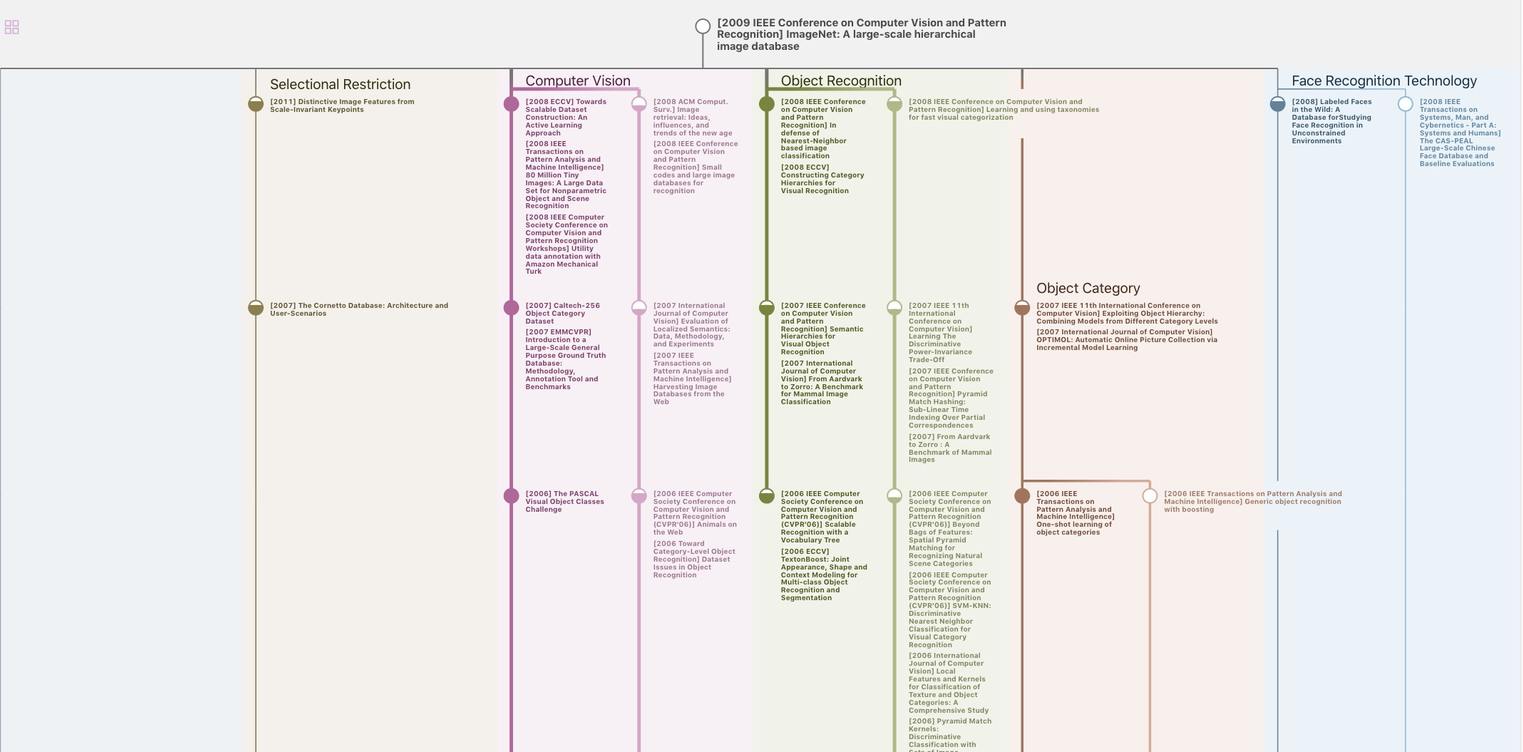
生成溯源树,研究论文发展脉络
Chat Paper
正在生成论文摘要