MASiNet: Network Intrusion Detection for IoT Security Based on Meta-Learning Framework
IEEE Internet of Things Journal(2024)
Abstract
The rapid proliferation of Internet of Things (IoT) devices has led to an increased need for robust and efficient intrusion detection systems capable of identifying and mitigating novel threats. Traditional methods often struggle with the scarcity of labeled anomaly data, which is highly consequential, particularly in the context of IoT. In this study, we propose a novel few-shot learning approach by leveraging a Multi-Stage Attention Siamese Network (MASiNet) for network traffic intrusion detection based on meta-learning framework. Unlike traditional methods, the proposed MASiNet model is capable of detecting intrusions with minimal labeled samples, addressing the challenge of scarce anomaly data. The model is trained using various attack samples and evaluates unknown samples by comparing similarities with a small set of known attack types. A well-structured cost function design, incorporating two specific losses, is introduced to optimize the effectiveness of the training process. Tested on the NSL_KDD and UNSW-NB15 datasets in a simulated few-shot learning environment, the MASiNet model demonstrates superior performance in terms of accuracy, precision, False Alarm Rate (FAR), outperforming existing methods. Furthermore, we have validated our approach through real-world evaluations. The proposed method provides an effective solution for intrusion detection in the context of few-shot learning, offering a proficient solution that aligns with the dynamic nature of IoT networks.
MoreTranslated text
Key words
IoT security,Intrusion detection,Meta-learning framework,Few-shot learning
AI Read Science
Must-Reading Tree
Example
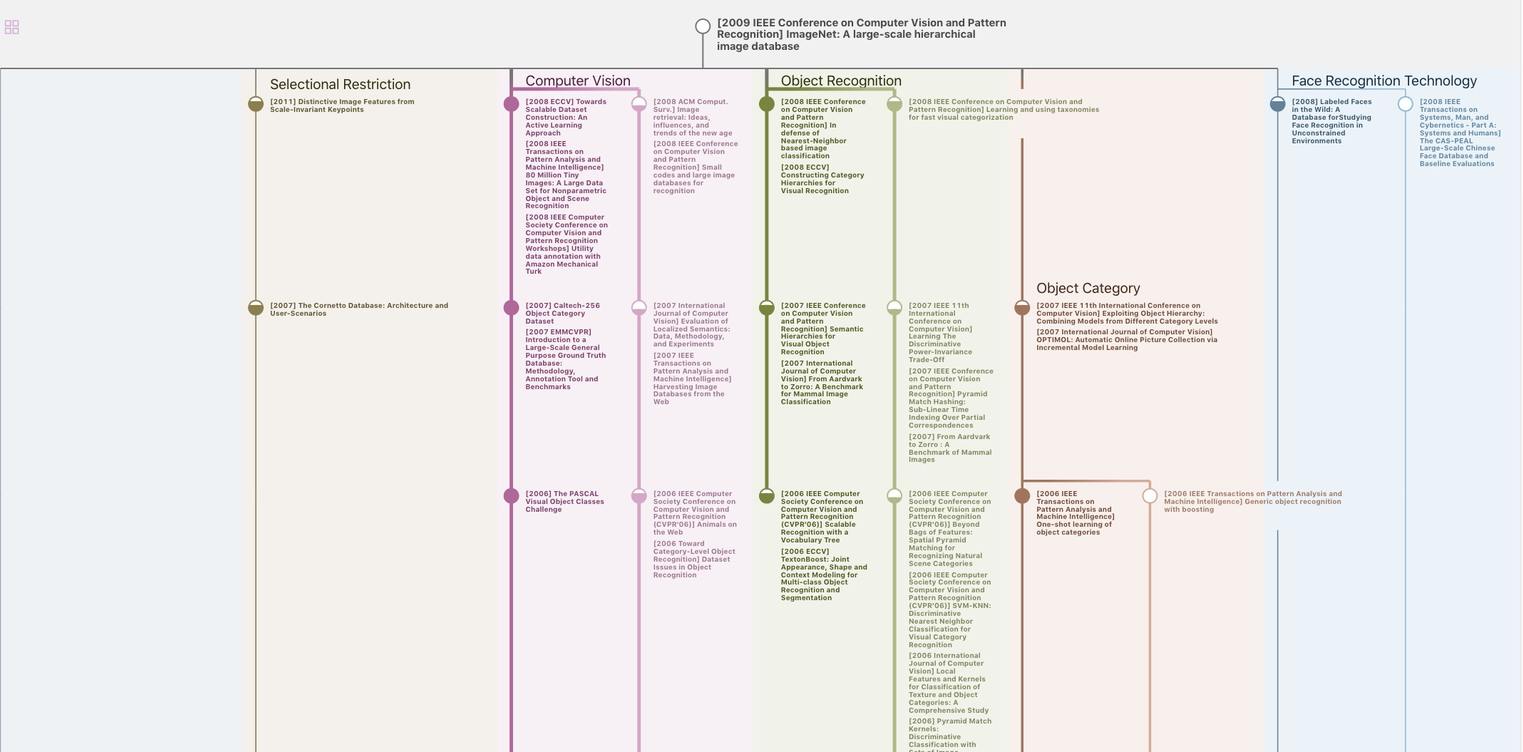
Generate MRT to find the research sequence of this paper
Chat Paper
Summary is being generated by the instructions you defined