Learning Accurate Network Dynamics for Enhanced Adaptive Video Streaming
IEEE TRANSACTIONS ON BROADCASTING(2024)
摘要
The adaptive bitrate (ABR) algorithm plays a crucial role in ensuring satisfactory quality of experience (QoE) in video streaming applications. Most existing approaches, either rule-based or learning-driven, tend to conduct ABR decisions based on limited network statistics, e.g., mean/standard deviation of recent throughput measurements. However, all of them lack a good understanding of network dynamics given the varying network conditions from time to time, leading to compromised performance, especially when the network condition changes significantly. In this paper, we propose a framework named ANT that aims to enhance adaptive video streaming by accurately learning network dynamics. ANT represents and detects specific network conditions by characterizing the entire spectrum of network fluctuations. It further trains multiple dedicated ABR models for each condition using deep reinforcement learning. During inference, a dynamic switching mechanism is devised to activate the appropriate ABR model based on real-time network condition sensing, enabling ANT to automatically adjust its control policies to different network conditions. Extensive experimental results demonstrate that our proposed ANT achieves a significant improvement in user QoE of 20.8%-41.2% in the video-on-demand scenario and 67.4%-134.5% in the live-streaming scenario compared to state-of-the-art methods, across a wide range of network conditions.
更多查看译文
关键词
Heuristic algorithms,Throughput,Streaming media,Prediction algorithms,Quality of experience,Bit rate,Adaptive systems,Network dynamics learning,video on demand,live streaming,adaptive bitrate,reinforcement learning,quality of experience
AI 理解论文
溯源树
样例
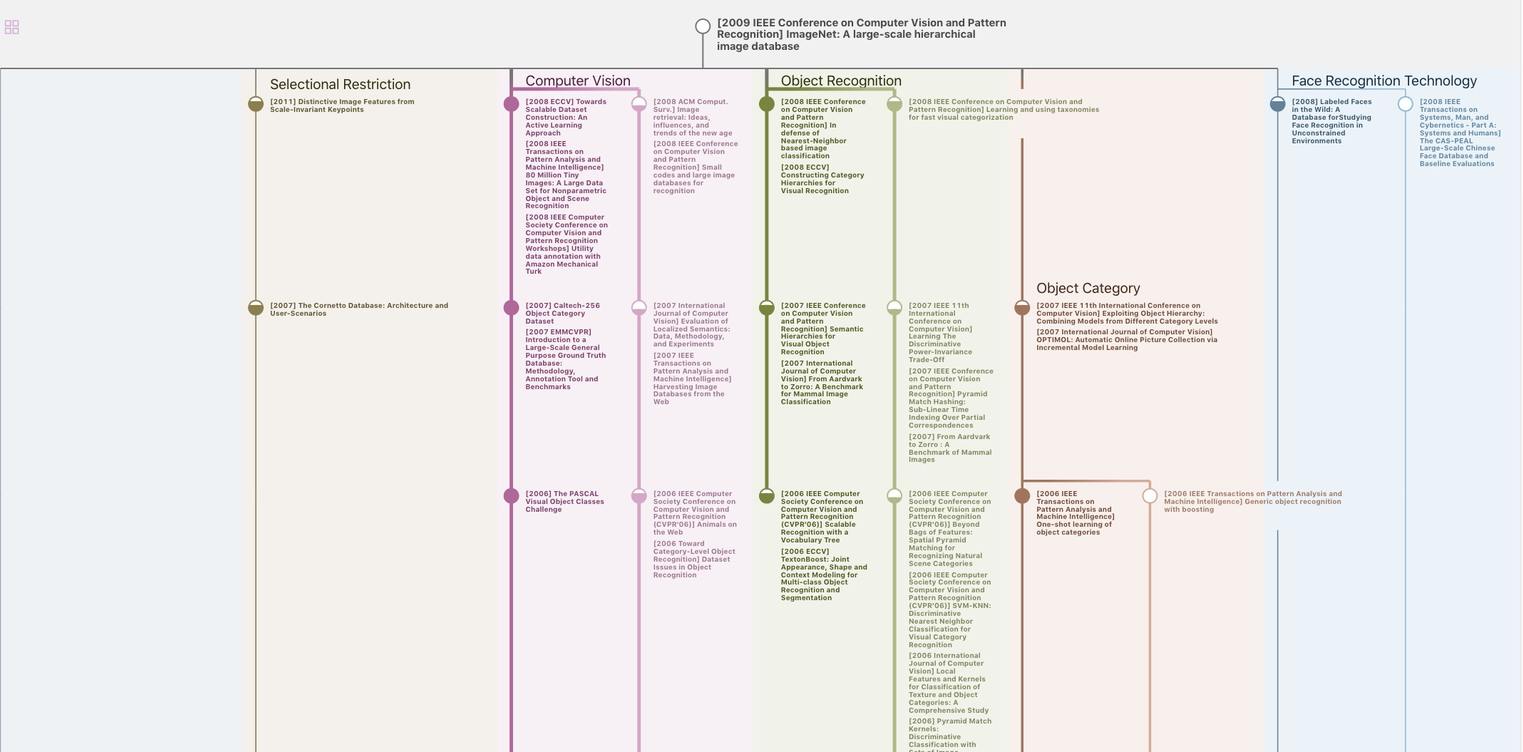
生成溯源树,研究论文发展脉络
Chat Paper
正在生成论文摘要