Site-specific fertilizer recommendation using data driven machine learning enhanced wheat productivity and resource use efficiency
Field Crops Research(2024)
Abstract
Context
Fertilizer use efficiency and profitability are very low due to blanket fertilizer recommendations in sub-Saharan Africa. It is crucial to establish tailored recommendations that account for local conditions. Countries like Ethiopia are moving towards adopting site-specific fertilizer recommendations (SSFR) that are developed using machine learning (ML) and designed to enhance yields, profitability, and environmental benefits.
Objective
The objective of this study was to evaluate the performance of ML generated SSFR for wheat in improving resource use efficiency, yields, and profitability compared to local (LBFR) and national (NBFR) blanket fertilizer recommendations.
Methods
ML was used to develop SSFR for wheat in Ethiopia. Farmer replicated on-farm validation trials were conducted across 277 sites using a randomized complete block design. Data on farm management history, yields, and prices for fertilizer and grain were gathered using Open Data Kit (ODK) tools. Key performance metrics, including site-specific yield gains or losses, profitability, and resource use efficiencies were computed for each site. Data analysis and the presentation of results were conducted using R software packages.
Results
The study indicated variability in resource use efficiency, yields, and profits within and across the testing districts. Performance of SSFR was superior in 75% and 72%, lower in 14% and 21%, and comparable in 10% and 7% of the testing sites compared to NBFR and LBFR, respectively. SSFR led to average grain yield increase of 16% and 25% over NBFR and LBFR, respectively. P and S use efficiency were low with SSFR compared to the blanket recommendations. SSFR increased nitrogen use efficiency by 30% and water use efficiency by 0.58 kg and 0.83 kg of grain per ha per mm of water over NBFR and LBFR, respectively. Furthermore, SSFR yielded profit gain of USD580 per hectare per season over LBFR and USD412 over NBFR.
Conclusions
SSFR using ML was effective at enhancing wheat productivity, profitability, and resource use efficiency. The yield loss at a few locations and reductions in P and S use efficiency with the SSFR underscore the importance of improving the predictive ability of the ML algorithm by incorporating a broader array of variables and data from diverse wheat farming contexts.
Significance
This study underlines the innovative use of data-driven ML approach to optimize fertilizer use in developing countries. The findings support the pilot expansion of SSFR under diverse conditions to optimize fertilizer efficiency and increase crop productivity and profitability for smallholder farmers.
MoreTranslated text
Key words
Fertilizer,Machine learning,Profit,Site-specific,Wheat
AI Read Science
Must-Reading Tree
Example
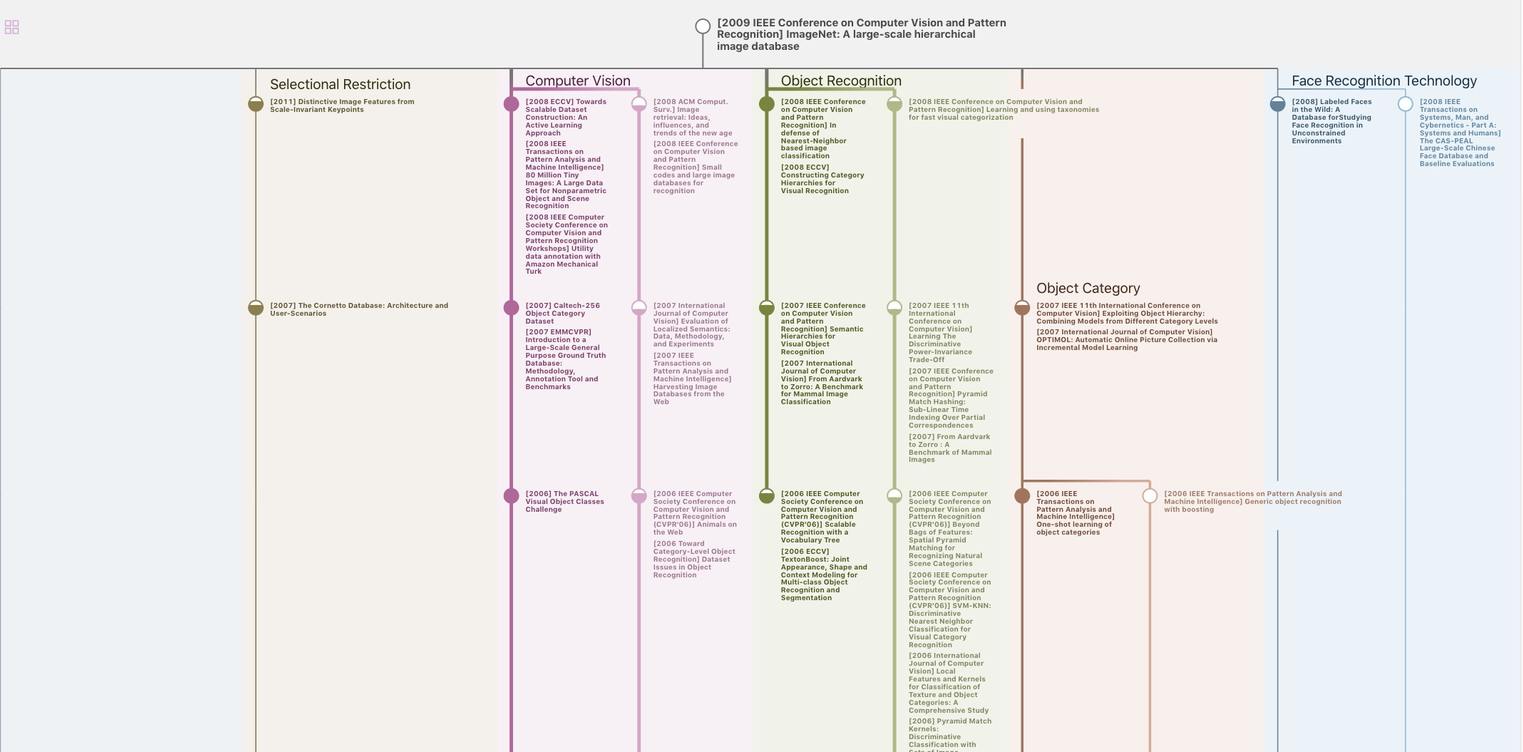
Generate MRT to find the research sequence of this paper
Chat Paper
Summary is being generated by the instructions you defined