Coupling of weather generator and crop model for enhanced low-temperature stress sample augmentation in Henan Province, PR China
Computers and Electronics in Agriculture(2024)
摘要
This study proposes a method for generating virtual samples using a weather generator and crop model to address the scarcity of low-temperature stress samples in winter wheat. Firstly, simulated meteorological data is generated using the weather generator, and then virtual meteorological yield and leaf area index (LAI) from jointing to heading stages are calculated using the crop model. These virtual samples integrate location information from weather stations, low-temperature statistics derived from simulated meteorological data, LAI data, and corresponding simulated meteorological yields. The impact of low-temperature stress on these virtual samples is assessed based on computed statistical data. Validation using real data from Henan Province confirms the similarity between generated low-temperature virtual samples and a low-temperature stress test set. The effectiveness of the created low-temperature virtual samples is evaluated under various low-temperature stress conditions using the random forest method, indicating that increasing the number of low-temperature virtual samples in the training set improves the accuracy of yield prediction models. Specifically, the increase in R2 value and the decrease in RMSE and MAE values validate the efficiency of generating virtual samples. Notably, virtual samples generated by consecutive frost days (CFD) perform excellently under different low-temperature stress and temperature conditions. When all virtual samples in the training set are low-temperature samples, using virtual samples generated based on CFD significantly improves prediction accuracy compared to the case without low-temperature virtual samples. Furthermore, training yield prediction algorithms using generated low-temperature virtual samples can reduce the overestimation of yields from more weather stations, thus enhancing prediction accuracy and reliability. In conclusion, this study effectively addresses the scarcity of low-temperature stress samples in winter wheat, reducing yield overestimation in certain areas. The choice of generation strategy for producing appropriate low-temperature virtual samples should be based on the settings of the training set and accuracy requirements.
更多查看译文
关键词
Weather generator,Crop model,Low-temperature virtual samples,Random forest
AI 理解论文
溯源树
样例
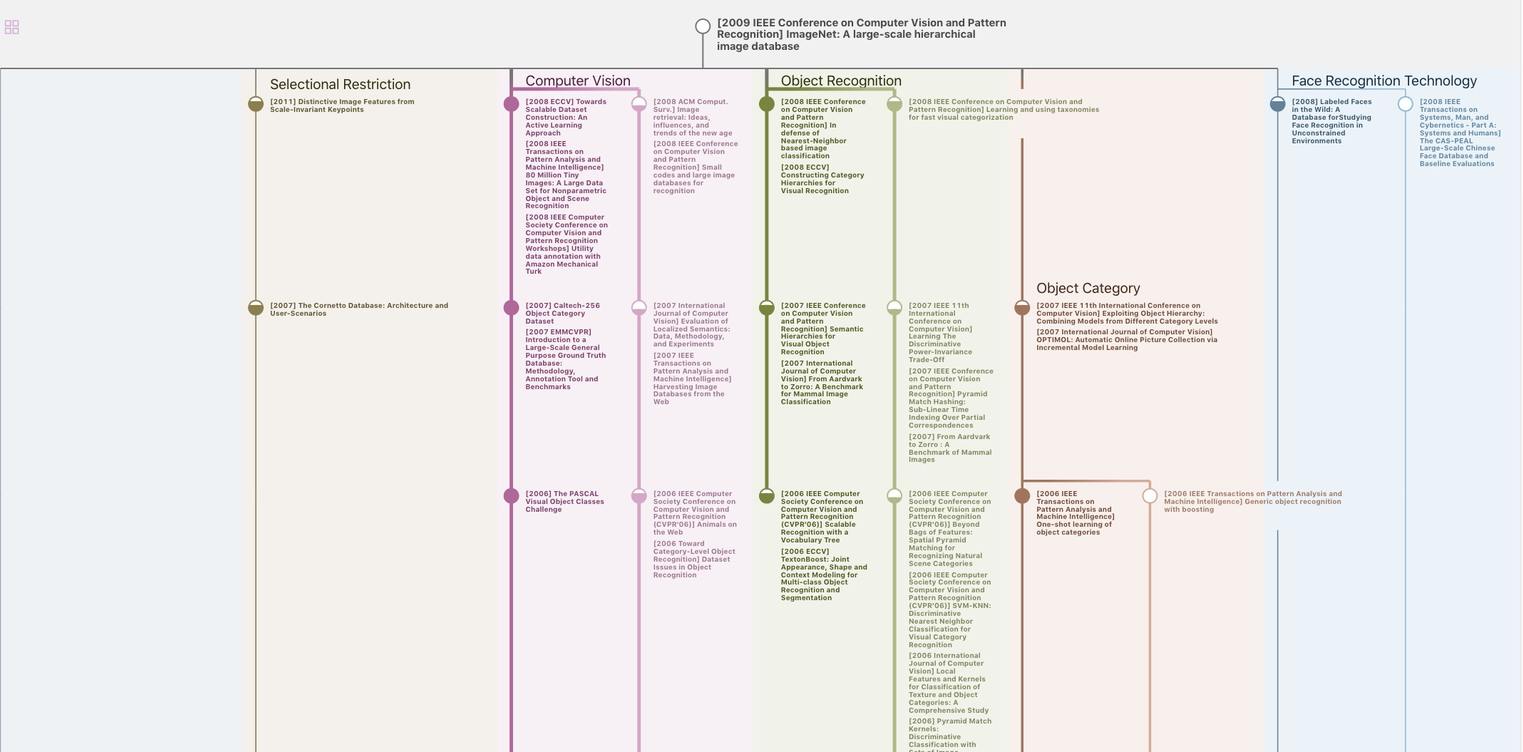
生成溯源树,研究论文发展脉络
Chat Paper
正在生成论文摘要