Coordinate-Free and Low-Order Scaling Machine Learning Model for Atomic Partial Charge Prediction for Any Size of Molecules.
Journal of chemical information and modeling(2024)
摘要
The atomic partial charge is of great importance in many fields, such as chemistry and drug-target recognition. However, conventional quantum-based computing of atomic charges is relatively slow, limiting further applications of atomic charge analysis. With the help of machine learning methods, various kinds of models appear to speed up atomic charge calculations. However, there are still some concerning problems. Some models based on geometric coordinates require high-accuracy geometry optimization as a preprocess, while other models have a limitation on the size of input molecules that narrow the applications of the model. Here, we propose a machine learning atomic charge model based on a message-passing featurizer. This preprocessing featurizer can quickly extract atomic environment information from a molecule according to the connectivity inside the molecule. The resulting descriptor can be used with a neural network to quickly predict the atomic partial charge. The model is able to automatically adapt to any size of molecule while remaining efficient and achieves a root-mean-square error in the Hirshfeld charge prediction of 0.018e, with an overall time complexity of O(n2). Thus, this model could enlarge the range of applications of atomic partial charge to more fields and cases.
更多查看译文
AI 理解论文
溯源树
样例
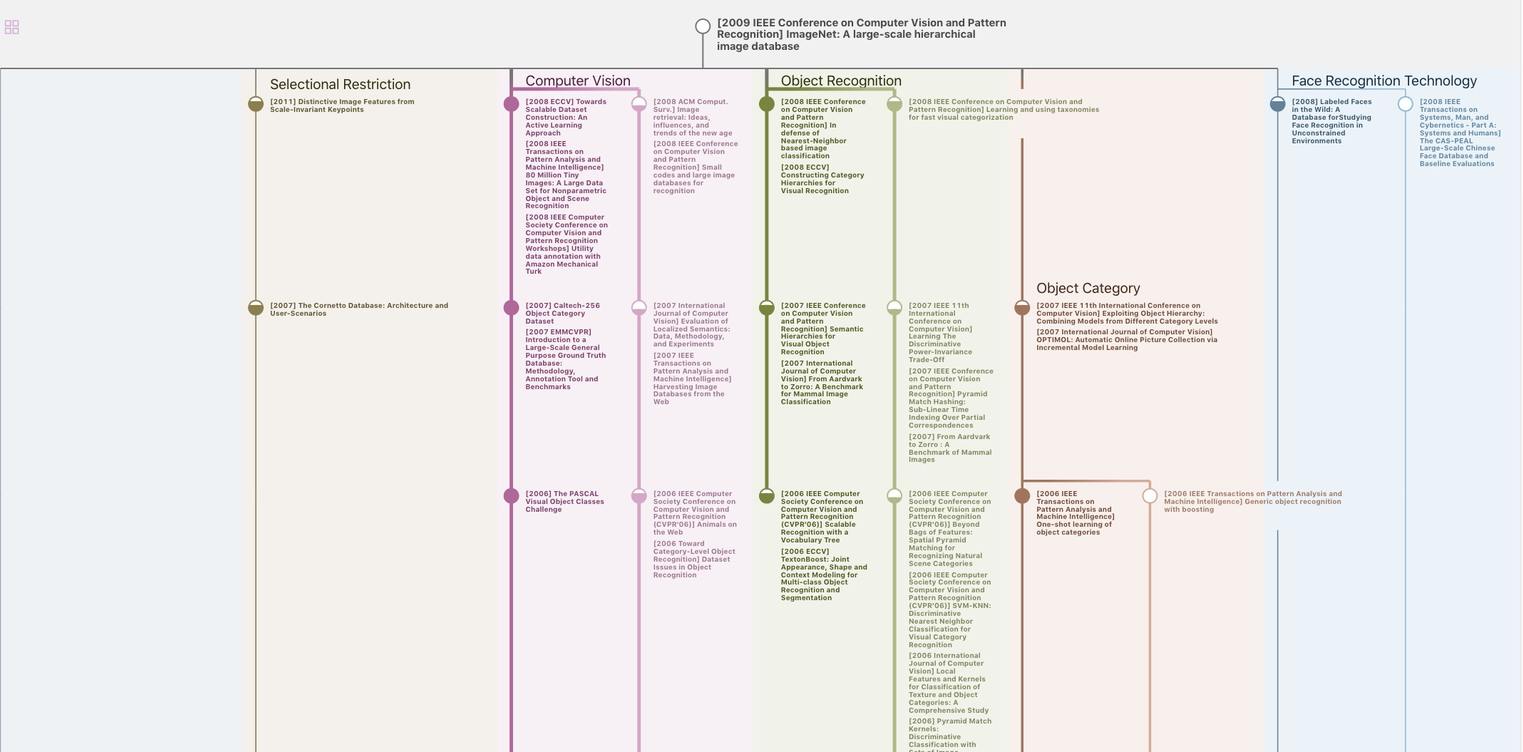
生成溯源树,研究论文发展脉络
Chat Paper
正在生成论文摘要