Accelerating the Screening of Modified MA2Z4 Catalysts for Hydrogen Evolution Reaction by Deep Learning‐Based Local Geometric Analysis
ENERGY & ENVIRONMENTAL MATERIALS(2024)
摘要
Machine learning (ML) integrated with density functional theory (DFT) calculations have recently been used to accelerate the design and discovery of single‐atom catalysts (SACs) by establishing deep structure–activity relationships. The traditional ML models are always difficult to identify the structural differences among the single‐atom systems with different modification methods, leading to the limitation of the potential application range. Aiming to the structural properties of several typical two‐dimensional MA2Z4‐based single‐atom systems (bare MA2Z4 and metal single‐atom doped/supported MA2Z4), an improved crystal graph convolutional neural network (CGCNN) classification model was employed, instead of the traditional machine learning regression model, to address the challenge of incompatibility in the studied systems. The CGCNN model was optimized using crystal graph representation in which the geometric configuration was divided into active layer, surface layer, and bulk layer (ASB‐GCNN). Through ML and DFT calculations, five potential single‐atom hydrogen evolution reaction (HER) catalysts were screened from chemical space of 600 MA2Z4‐based materials, especially V1/HfSn2N4(S) with high stability and activity (ΔGH* is 0.06 eV). Further projected density of states (pDOS) analysis in combination with the wave function analysis of the SAC‐H bond revealed that the SAC‐dz2 orbital coincided with the H‐s orbital around the energy level of −2.50 eV, and orbital analysis confirmed the formation of σ bonds. This study provides an efficient multistep screening design framework of metal single‐atom catalyst for HER systems with similar two‐dimensional supports but different geometric configurations.
更多查看译文
AI 理解论文
溯源树
样例
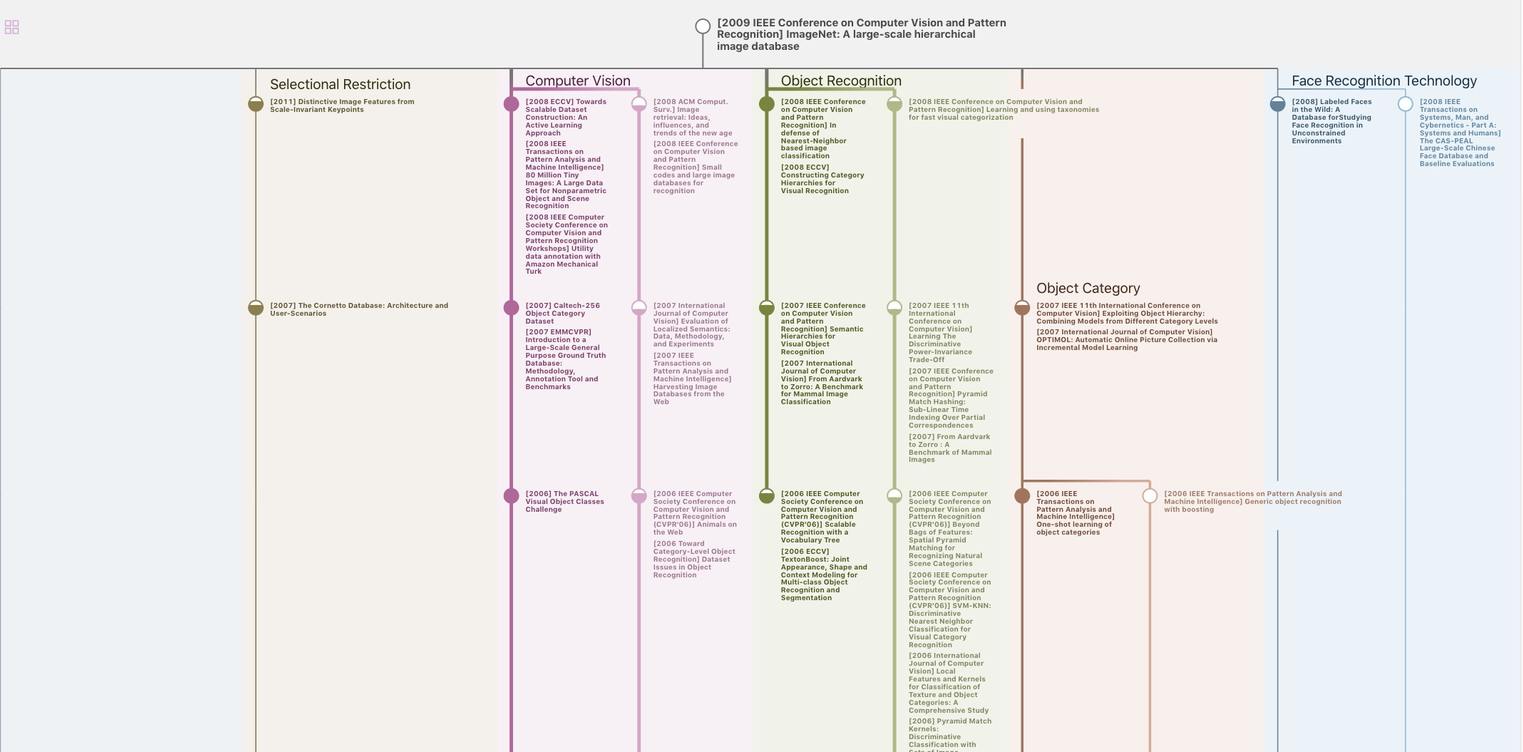
生成溯源树,研究论文发展脉络
Chat Paper
正在生成论文摘要