Abstract P464: Performance of an Automated Sleep Scoring Approach for Actigraphy Data in Children and Adolescents
Circulation(2024)
Abstract
Background: Actigraphy has advanced the measurement of sleep for cardiometabolic research in childhood. However, actigraphy methods lack standardization, limiting rigor and reproducibility. GGIR, an open-source R package, facilitates raw accelerometer data processing to generate sleep data in a standardized manner. This tool has been validated and applied to adult data, but less is known about the suitability of this tool for generating sleep estimates among children. We aimed to (1) determine the validity of multiple sleep scoring settings available within GGIR in children, and (2) evaluate how this tool automatically generates sleep data in community-based pediatric studies. Method: The validation study included 30 children (14 female, 8-16y) referred for in-lab overnight polysomnography at Children’s Hospital of Philadelphia. Participants wore a GENEActiv during polysomnography that captured data at 50 Hz, which were aligned into 30-second epochs against the sleep stages from polysomnography scored by a sleep medicine physician. Balanced accuracy was the primary validation metric, which is the average of sensitivity and specificity. For community-based testing, three pediatric cohorts were used: Study 1 (Philadelphia; 12-16y; GENEActiv, N=251), Study 2 (Mexico City, 9-18y; ActiGraph GT3X-BT; N=543), and Study 3 (Houston; 5-8y; ActiGraph GT3X+, N=226). We processed raw accelerometry data with the Cole-Kripke (CK), Sadeh (S), and Van Hees (VH) algorithm settings. Result: For in-lab validation, 271.5 hours of polysomnography data were collected with 80% of the epochs scored as sleep; sleep duration averaged 7.3 hours (SD = 1.3). In rank order, average balanced accuracies were 0.81 (SD = 0.08) for GGIR-CK, 0.77 (SD = 0.09) for GGIR-VH, and 0.68 (SD = 0.11) for GGIR-S. The GGIR-S algorithm had the poorest detection of night awakenings (specificity: 0.38 [SD = 0.21]). For community-based data, without manually altering the data, the average sleep duration was highest when using the Sadeh algorithm in each study (8.3 [SD = 1.6], 8.0 [SD = 1.9], and 5.2 [SD = 4.4] hours for studies 1-3) and lower when using the GGIR-CK algorithm (7.1 [SD = 1.4], 6.9 [SD = 1.7], 4.3 [SD = 3.7] hours for studies 1-3). Approximately 2% of the data were affected by obvious errors (e.g., negative wake after sleep onset), with almost all such errors detected with the Sadeh algorithm. Conclusion: In a controlled setting, GGIR-CK had the optimal balanced accuracy performance for estimating sleep duration in children. In keeping with the lab-based finding of poorer detection of night awakenings, sleep duration was highest in the community samples when using GGIR-S. When applied to community-based data the automated GGIR approach generated sleep data that included errors, necessitating additional data cleaning. Incorporating standardized data cleaning steps within GGIR would improve rigor and reproducibility.
MoreTranslated text
AI Read Science
Must-Reading Tree
Example
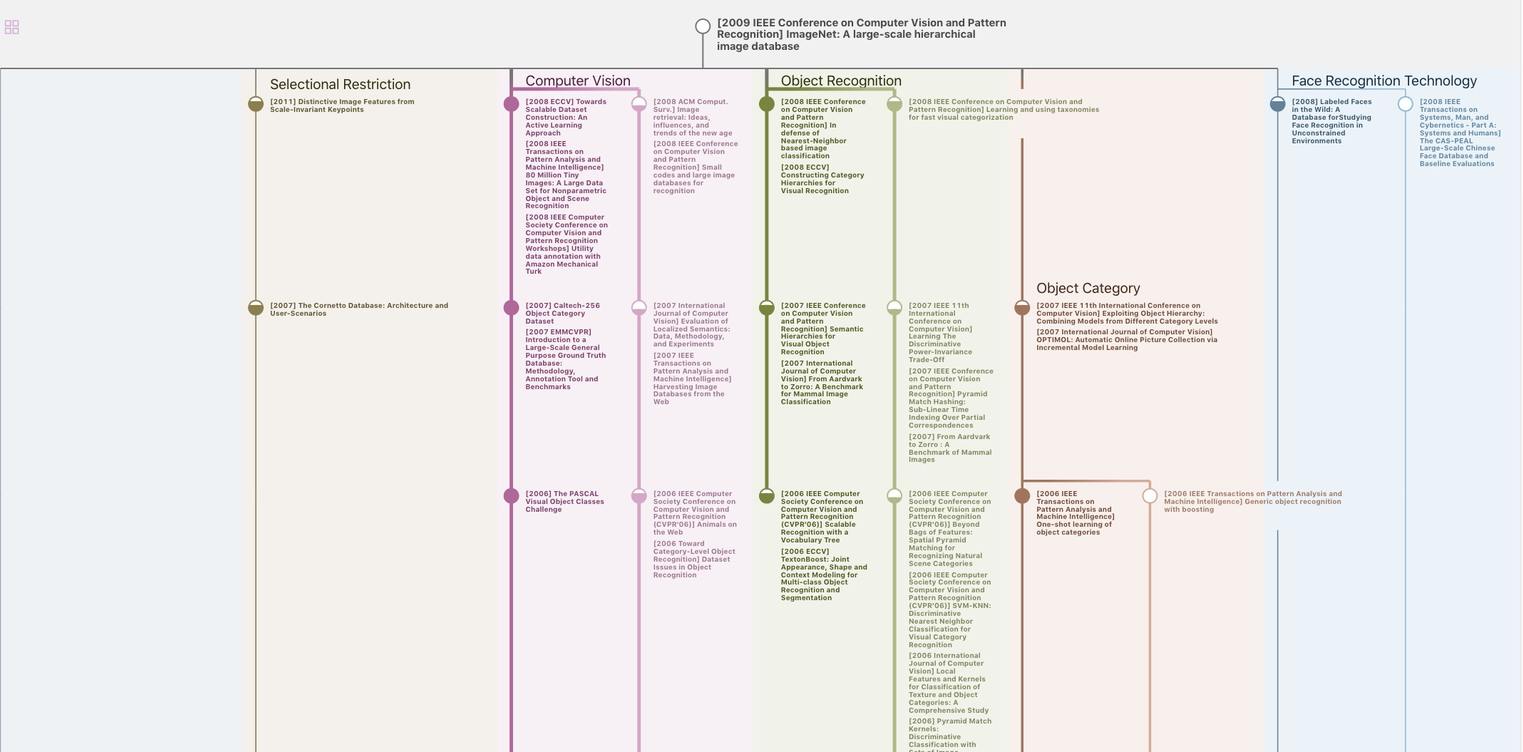
Generate MRT to find the research sequence of this paper
Chat Paper
Summary is being generated by the instructions you defined