Learning Cross Dimension Scene Representation for Interactive Navigation Agents in Obstacle-Cluttered Environments
IEEE ROBOTICS AND AUTOMATION LETTERS(2024)
Abstract
Embodied visual navigation has witnessed significant advancements. However, most studies commonly assume that environments are static and contain at least one collision-free path. In human environments, agents frequently encounter challenges when navigating through scenes with disarranged objects. In this letter, we explore the interactive navigation problem, wherein agents possess the ability to physically interact with and modify the environment, such as moving obstacles aside, to improve their efficiency in reaching the target. To this end, we propose a novel cross dimension scene representation module under the framework of reinforcement learning (RL) that provides joint 2D and 3D scene representation for interactive agents. We first leverage 2D and 3D observation encoders to extract informative features from observations. Subsequently, a joint representation network is proposed to lift the dimension of 2D feature maps to 3D and align them with 3D observation, enabling us to fuse information from different dimensions. This allows us to simultaneously harness the advantages of 2D and 3D observations, thereby yielding a more informative representation for interactive RL agents in addressing challenges arising from physical interactions. We validate our proposed approach in the iGibson environment, and experimental results demonstrate a significant improvement over baseline methods.
MoreTranslated text
Key words
Navigation,Three-dimensional displays,Visualization,Task analysis,Robots,Semantics,Feature extraction,Vision-based navigation,deep learning in robotics and automation,mobile manipulation
AI Read Science
Must-Reading Tree
Example
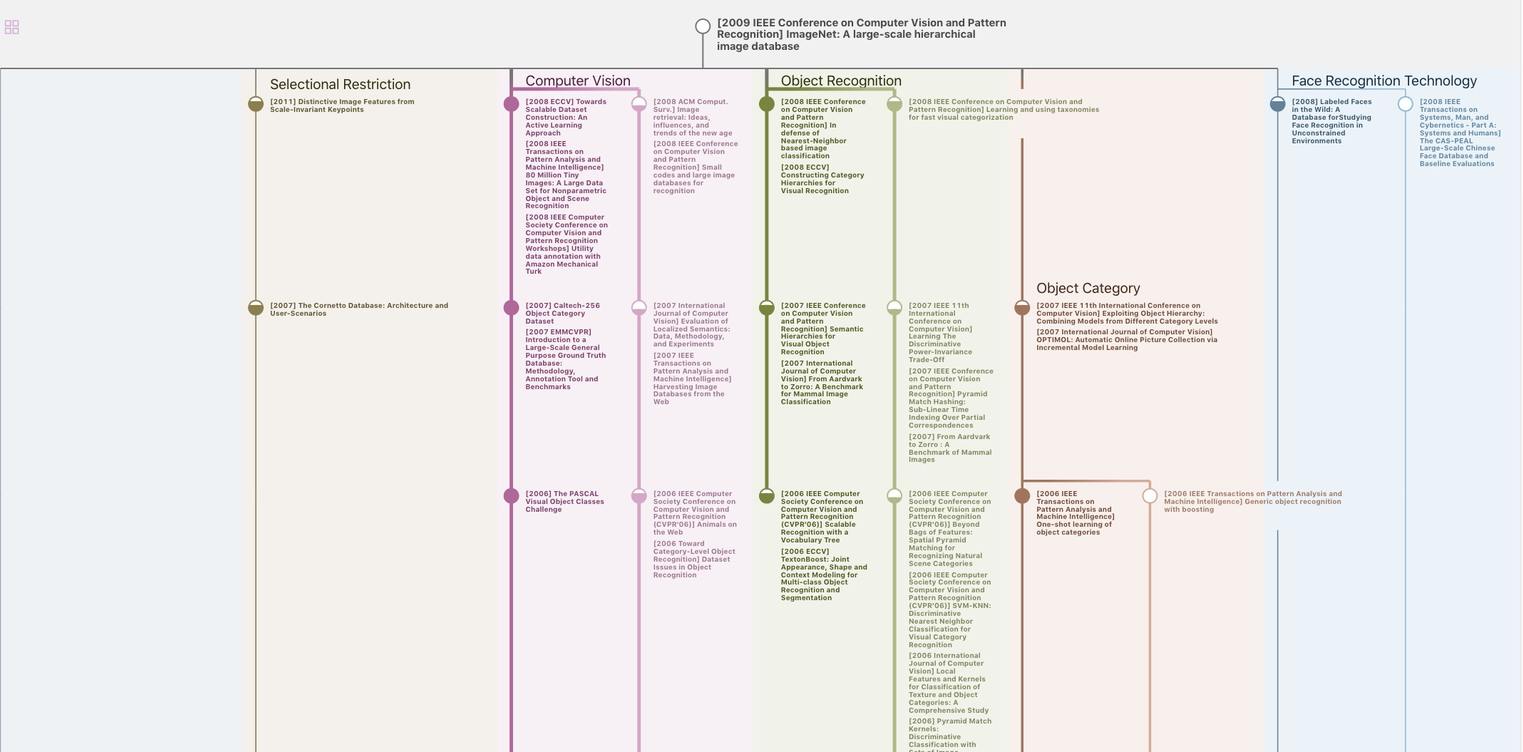
Generate MRT to find the research sequence of this paper
Chat Paper
Summary is being generated by the instructions you defined