Scalogram-Based Gait Abnormalities Classification Using Deep Convolutional Networks for Neurological and Non-Neurological Disorders
Journal of Medical and Biological Engineering(2024)
摘要
In the present day, there is a steep increase in cases of neurological and non-neurological diseases that may affect a person’s normal gait. This study proposes a methodology for classifying gait abnormalities using convolutional neural networks based on scalogram analysis of electromyography and foot insole data. This study utilizes scalograms for classifying electromyography and foot-insole data, offering robust handling of high-noise data with sudden transitions. The electromyography data is sourced from patients with hemiplegia, rheumatoid arthritis, prolapsed intervertebral disc, and osteoarthritis. Foot insole data from PhysioNet includes subjects with Parkinson’s disease, Huntington’s disease, and amyotrophic lateral sclerosis. Classification tasks are performed using convolutional neural network architecture, with performance assessed across three optimizers—adam, stochastic gradient descent, and rmsprop—and employing both max and average pooling layers for enhanced model optimization. The results indicate promising classification performance, with an accuracy of 96.75
更多查看译文
关键词
Scalogram,Foot insole,Electromyography,Deep learning,Gait analysis
AI 理解论文
溯源树
样例
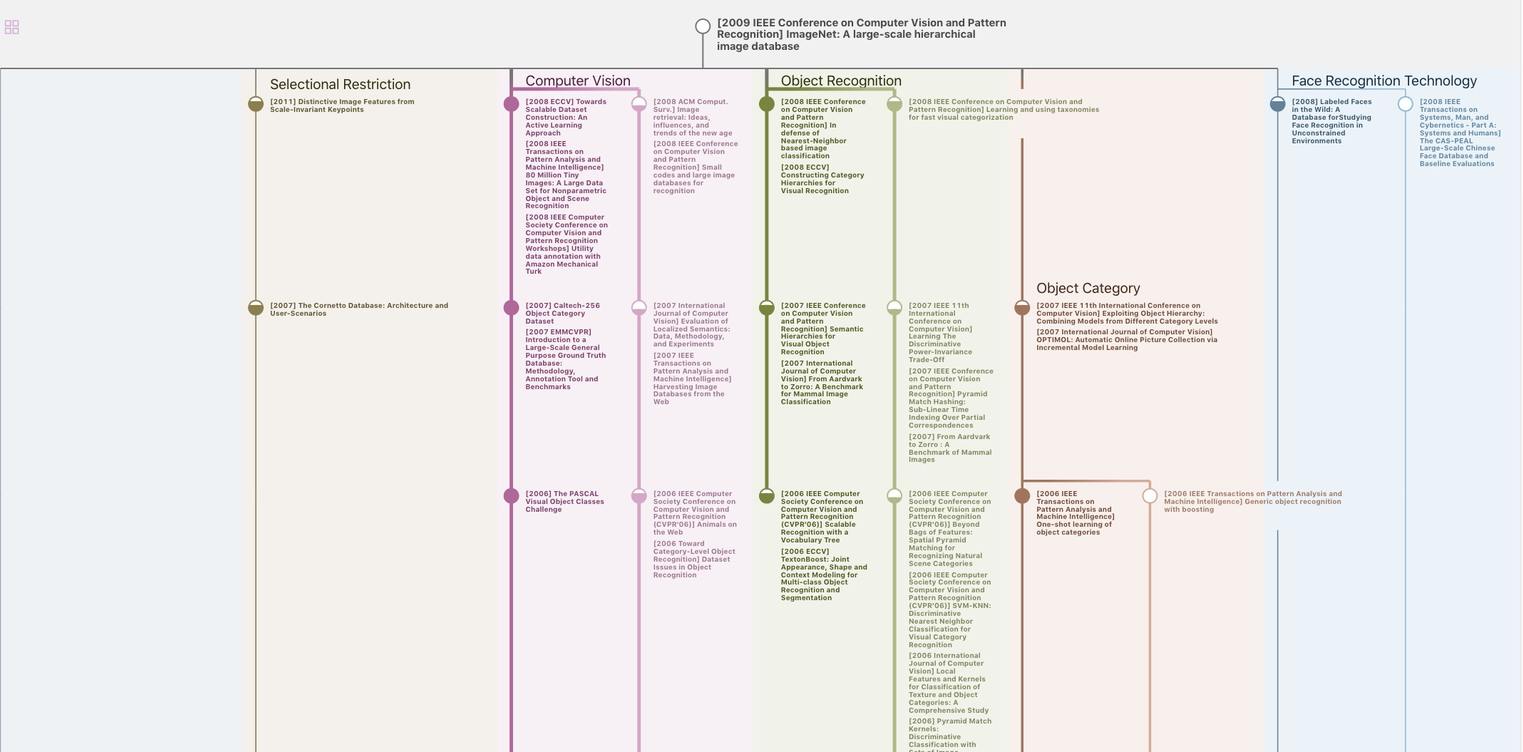
生成溯源树,研究论文发展脉络
Chat Paper
正在生成论文摘要