Leveraging Large Language Models for Automated Web-Form-Test Generation: An Empirical Study
CoRR(2024)
摘要
The testing of web forms is an essential activity for ensuring the quality of
web applications, which mainly involves evaluating the interactions between
users and forms. Automated test-case generation remains a challenge for
web-form testing: Due to the complex, multi-level structure of web pages, it
can be difficult to automatically capture their inherent contextual information
for inclusion in the tests. Large Language Models (LLMs) have great potential
for contextual text generation. OpenAI's GPT LLMs have been receiving a lot of
attention in software testing, however, they may fail to be applied in practice
because of information security concerns. To the best of our knowledge, no
comparative study examining different LLMs has yet been reported for
web-form-test generation. To address this gap in the literature, we conducted a
comprehensive empirical study investigating the effectiveness of 11 LLMs on 146
web forms from 30 open-source Java web applications. According to the
experimental results, different LLMs can achieve different testing
effectiveness. Notably, the GPT-4, GLM-4, and Baichuan2 LLMs can generate
better web-form tests than the others. Compared with GPT-4, other LLMs find it
difficult to generate appropriate tests for web forms, resulting in decreased
successfully-submitted rates (SSRs, measured by the proportions of the
LLMs-generated web-form tests that can be successfully inserted into the web
forms and submitted) ranging from 9.10
achieve higher SSRs than GPT-3.5, indicating a better ability to generate
appropriate tests for web forms. Our findings also show that, for all LLMs,
when the designed prompts include complete and clear contextual information
about the web forms, more effective web-form tests were generated. Finally, we
offer some insights for using LLMs to guide automated web-form testing.
更多查看译文
AI 理解论文
溯源树
样例
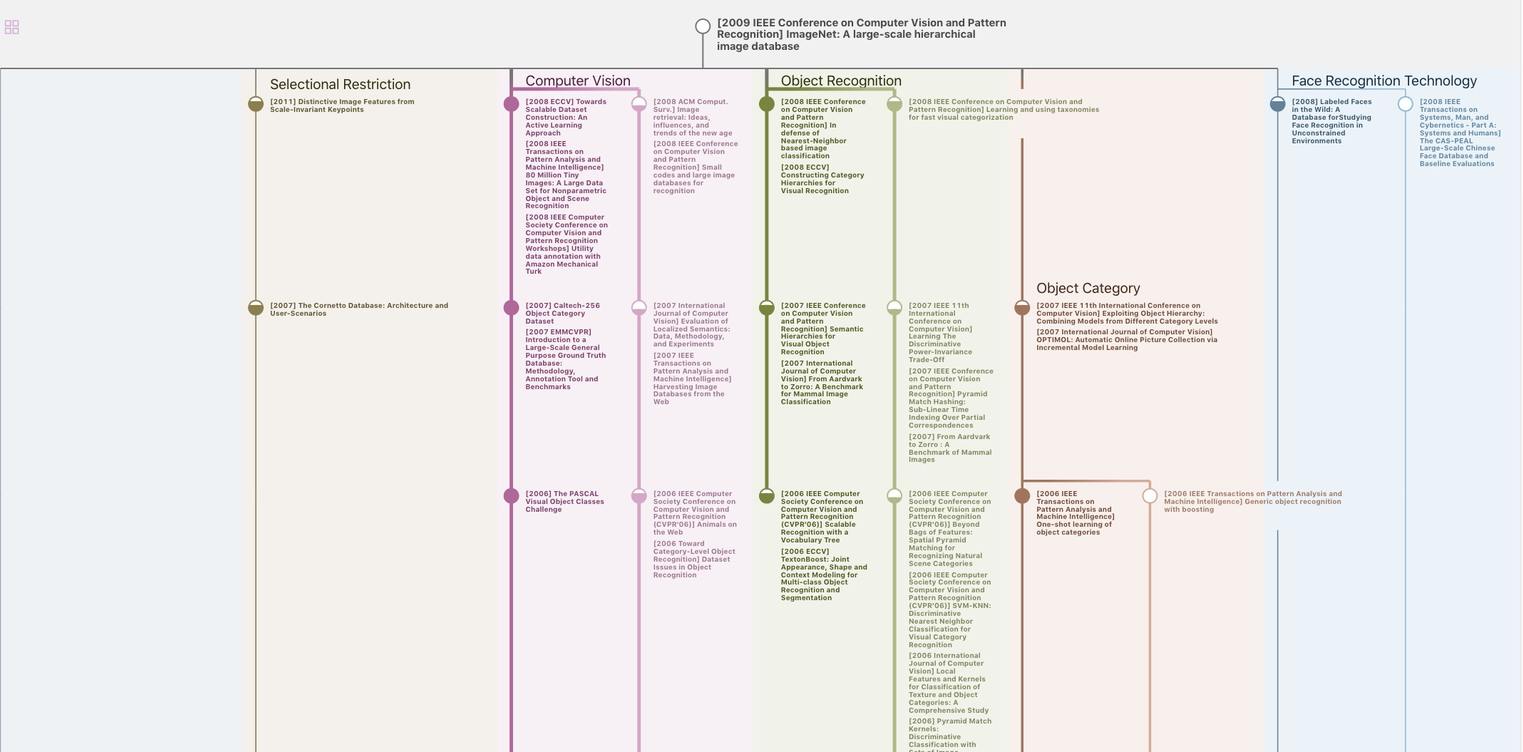
生成溯源树,研究论文发展脉络
Chat Paper
正在生成论文摘要