Triplet Graph Attention Network for EEG-Based Cross-Subject Emotion Recognition
2023 7th Asian Conference on Artificial Intelligence Technology (ACAIT)(2023)
Abstract
As the correlation between emotion and brain activities has been gradually investigated, it is promising to objectively model the brain mechanism of human emotion with EEG in medical applications, such as the diagnosis of dysthymic disorder and brain disease. However, the huge individual variation of EEG may lead to the failure of recognition, and most existing studies cannot effectively exploit the complex topological information between channels. In this paper, a triplet graph attention network jointly trained from both local and global features is proposed to address the above problems. Specifically, we preliminarily explore topological connectivity by fusing both physical connections and functional connections between electrodes. To alleviate the effects of subject variation and capture the most essential emotional neural patterns, we design a mechanism that can dynamically select samples to form triplets to improve the class discriminability and weaken the subject discriminability in the high-order feature space. In addition, our model can obtain rich node features and effective graph features at the local and global levels, respectively, to capture emotion-related neural patterns. The experimental results on the SEED dataset show the effectiveness of our method in EEG-based cross-subject emotion recognition, and the visualization of the data distribution significantly illustrates that our model can enhance the discriminability of the emotion class.
MoreTranslated text
Key words
affective computing,emotion recognition,EEG,cross-subject,graph neural network,triplet network
AI Read Science
Must-Reading Tree
Example
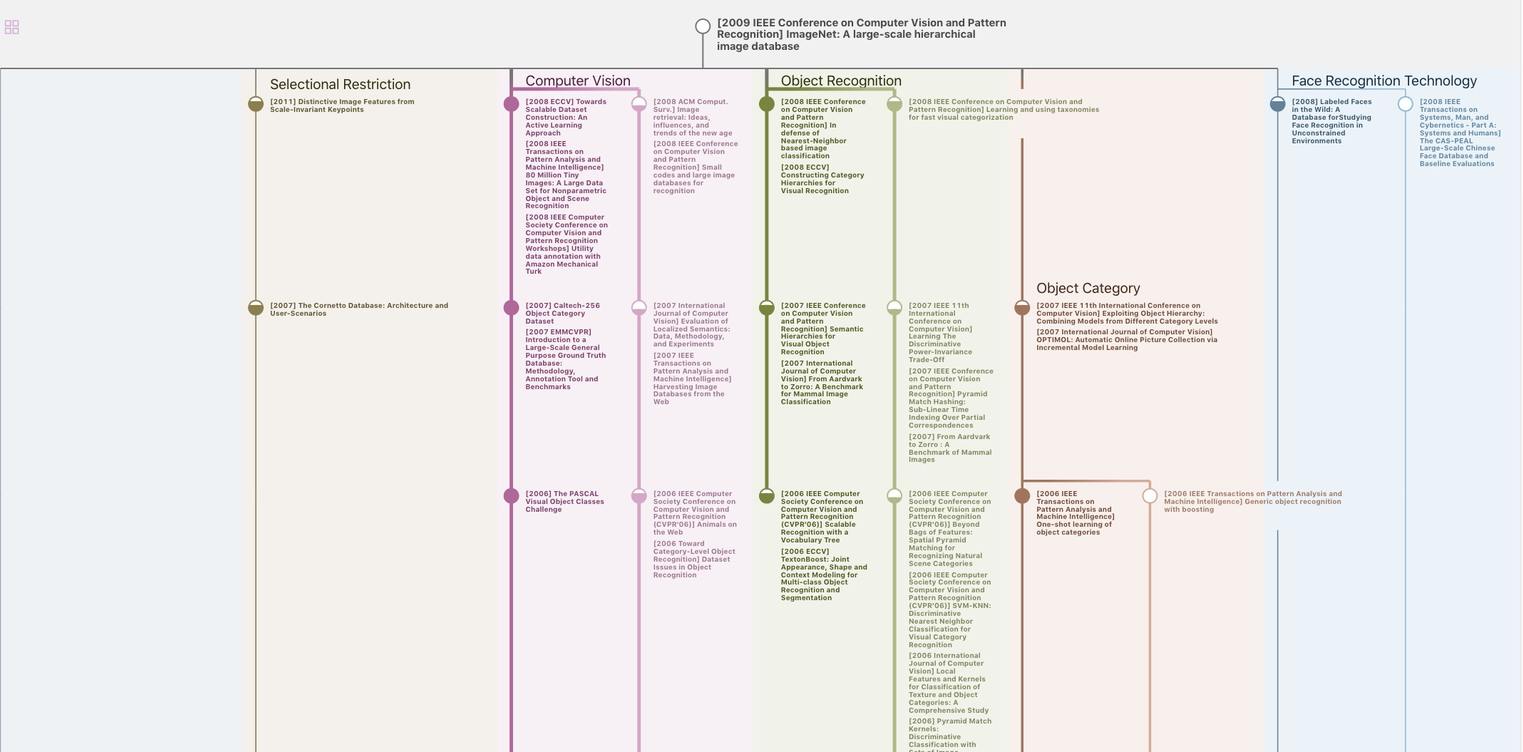
Generate MRT to find the research sequence of this paper
Chat Paper
Summary is being generated by the instructions you defined