ImputeCC Enhances Integrative Hi-C-Based Metagenomic Binning Through Constrained Random-Walk-Based Imputation.
Annual International Conference on Research in Computational Molecular Biology(2024)
摘要
Metagenomic Hi-C (metaHi-C) enables the recognition of relationships between contigs in terms of their physical proximity within the same cell, facilitating the reconstruction of high-quality metagenome-assembled genomes (MAGs) from complex microbial communities. However, current Hi-C-based contig binning methods solely depend on Hi-C interactions between contigs to group them, ignoring invaluable biological information, including the presence of single-copy marker genes. Here, we introduce ImputeCC, an integrative contig binning tool tailored for metaHi-C datasets. ImputeCC integrates Hi-C interactions with the inherent discriminative power of single-copy marker genes, initially clustering them as preliminary bins, and develops a new constrained random walk with restart (CRWR) algorithm to improve Hi-C connectivity among these contigs. Extensive evaluations on mock and real metaHi-C datasets from diverse environments, including the human gut, wastewater, cow rumen, and sheep gut, demonstrate that ImputeCC consistently outperforms other Hi-C-based contig binning tools. ImputeCC’s genus-level analysis of the sheep gut microbiota further reveals its ability and potential to recover essential species from dominant genera such as Bacteroides , detect previously unrecognized genera, and shed light on the characteristics and functional roles of genera such as Alistipes within the sheep gut ecosystem. Availability: ImputeCC is implemented in Python and available at https://github.com/dyxstat/ImputeCC . The Supplementary Information is available at https://doi.org/10.5281/zenodo.10776604 .
更多查看译文
AI 理解论文
溯源树
样例
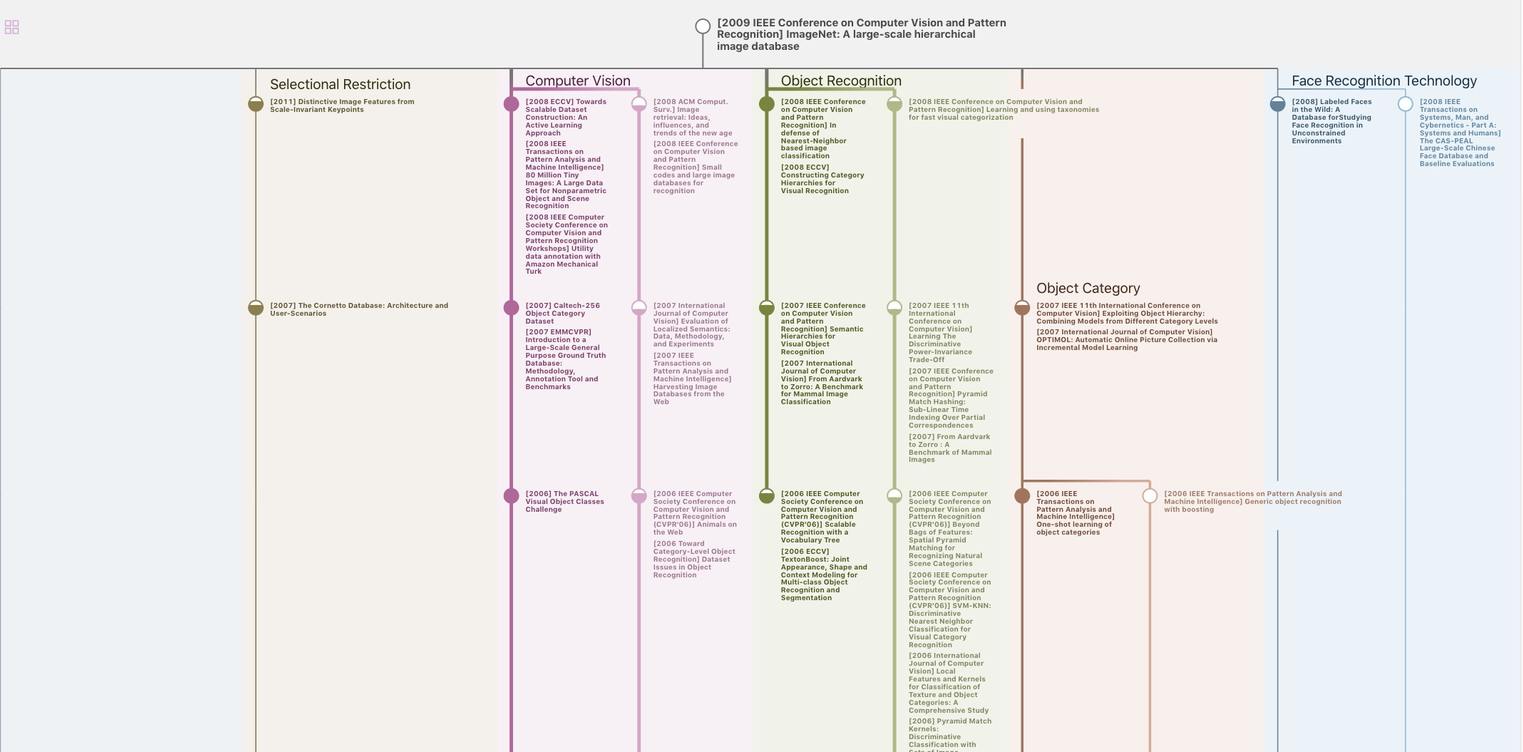
生成溯源树,研究论文发展脉络
Chat Paper
正在生成论文摘要