Computational Results Comparison of Discrete Time-Varying Nonlinear Optimization: A Recurrent Neural Network Method
2023 7th Asian Conference on Artificial Intelligence Technology (ACAIT)(2023)
Abstract
In this paper, we propose a new discrete-time neural network model to solve the discrete time-varying nonlinear optimization (DTNO) problems, and the truncation error of this model is raised to the fourth order for improving the calculative accuracy. Above all, the continuous time-varying nonlinear optimization (CTNO) problem is converted into the continuoustime Zhang neural network (ZNN) model through the ZNN design formula. Subsequently, a new discrete-time Zhang neural network (DTZNN) model is proposed rooted in the fundamental principles of general four-step discretization formula (FDF). Note that the proposed DTZNN model has a residual error with fourth-order pattern. During this process, in order to further investigate the impact of parameters for the truncation error, the optimal range of parameter is determined through comparative experiments. The final numerical experimental results indicate that the DTZNN model has the expected truncation error. When the values of parameter are confined within a specified range and remain relatively small, the calculative accuracy can further be improved.
MoreTranslated text
Key words
Zhang neural network (ZNN) model,discrete time-varing nonlinear optimization (DTNO),four-step discretization formula (FDF)
AI Read Science
Must-Reading Tree
Example
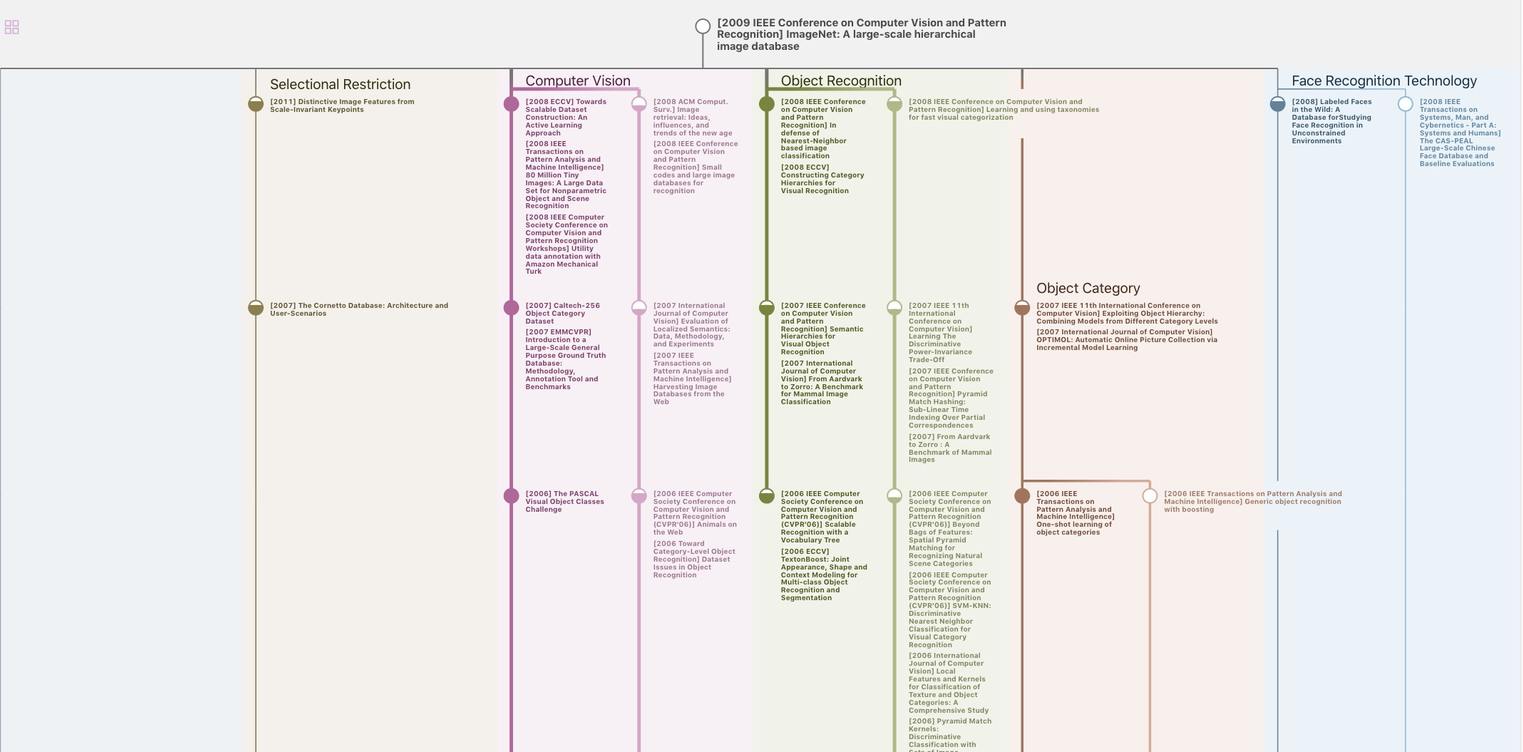
Generate MRT to find the research sequence of this paper
Chat Paper
Summary is being generated by the instructions you defined