A Closed-Loop Brain-Machine Interface SoC Featuring a 0.2μJ/class Multiplexer Based Neural Network
2024 IEEE Custom Integrated Circuits Conference (CICC)(2024)
Abstract
Closed-loop bidirectional brain-machine interface (CL-BBMI) consisting of a neural signal recorder, a neural signal processing unit (NPU) and a modulation module plays an important role in the cutting-edge research in neuroscience [1]–[2]. In addition to traditional electrical-mode modulation, optogenetic stimulation shows great potential in BBMI in recent years [3]–[4]. Among the three modules, the NPU is a key component for the closed-loop, but very limited solutions have been reported in literature [4]. A neural network (NN) is typically a high-accuracy solution for NPU but suffers unaffordable power con-sumption especially in the implantable scenarios of BMI. Table lookup based multiplier-free NN is a feasible low-power approach. However, the current lookup table (LUT) based table lookup solution still requires a huge quantity of random-access memory (RAM) [5].
MoreTranslated text
Key words
Neural Network,Convolutional Layers,Power Consumption,Average Accuracy,Electromyography,Pulse Width,Processing Unit,Weight Vector,Batch Normalization,Lookup Table,Neural Signals,Quantization Error,Neuroscience Research,Top Left,Top Right,Non-rapid Eye Movement,Random Access Memory,Sleep Stages,Optogenetic Stimulation,Amount Of Memory
AI Read Science
Must-Reading Tree
Example
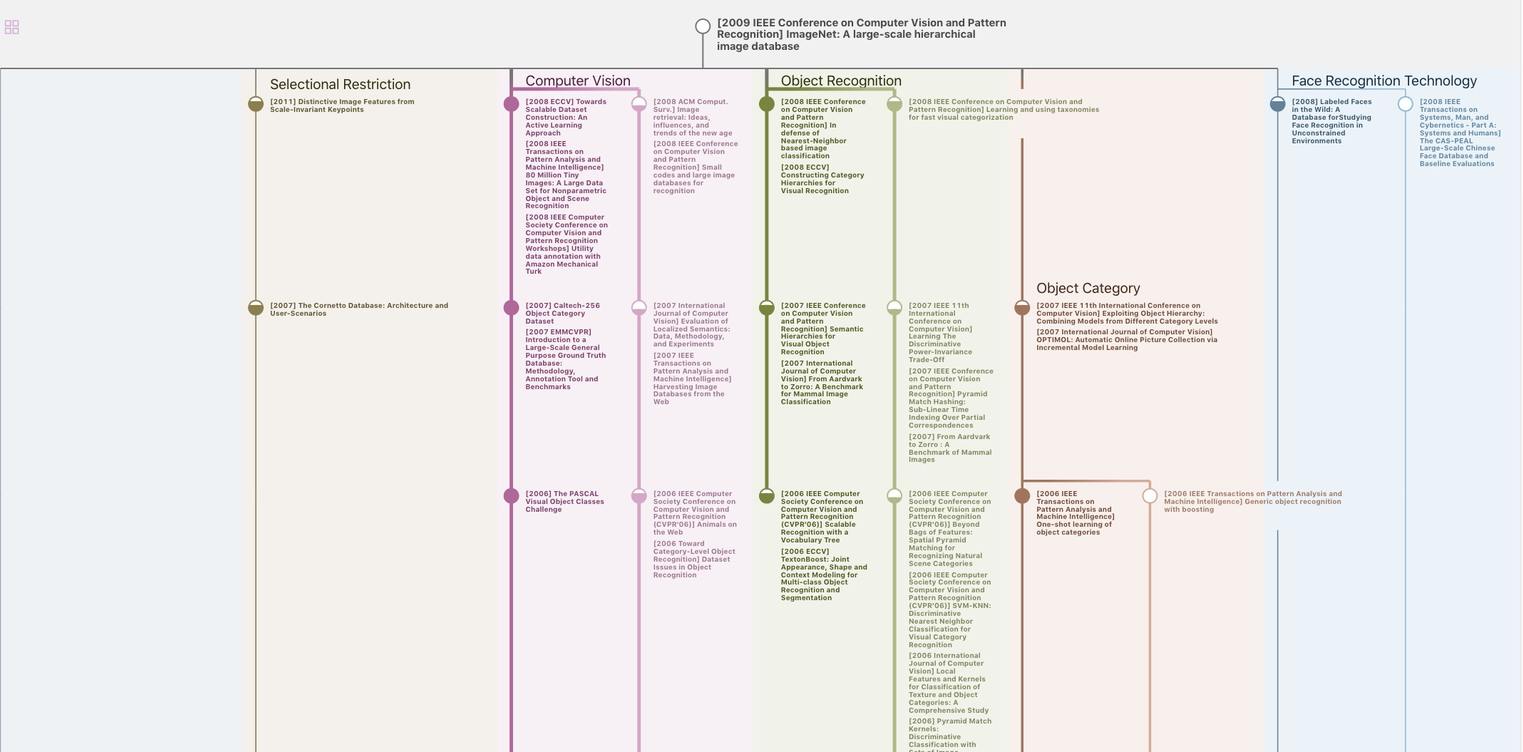
Generate MRT to find the research sequence of this paper
Chat Paper
Summary is being generated by the instructions you defined