Domino-Pro-Max: Towards Efficient Network Simplification and Reparameterization for Embedded Hardware Systems
IEEE Transactions on Computer-Aided Design of Integrated Circuits and Systems(2024)
摘要
The prohibitive complexity of convolutional neural networks (CNNs) has triggered an increasing demand for network simplification. To this end, one natural solution is to remove the redundant channels or layers to explore simplified network structures. However, the resulting simplified network structures often suffer from sub-optimal accuracy-efficiency trade-offs. To overcome such limitations, we, in this work, introduce a simple yet effective network simplification approach, namely Domino, which aims to comprehensively revisit the trade-off dilemma between accuracy and efficiency from a new perspective of linearity and non-linearity through linearity grafting. Furthermore, we also draw insights from Domino and introduce two enhanced variants, namely Domino-Pro and Domino-Pro-Max, to improve the attainable accuracy on target task without degrading the runtime efficiency on target hardware. Extensive experiments are conducted on two popular Nvidia Jetson embedded hardware systems (i.e., Xavier and Nano) and two representative deep convolutional networks (i.e., MobileNetV2 and ResNet50), which clearly demonstrate the superiority of Domino and its two enhanced variants over previous state-of-the-art methods.
更多查看译文
AI 理解论文
溯源树
样例
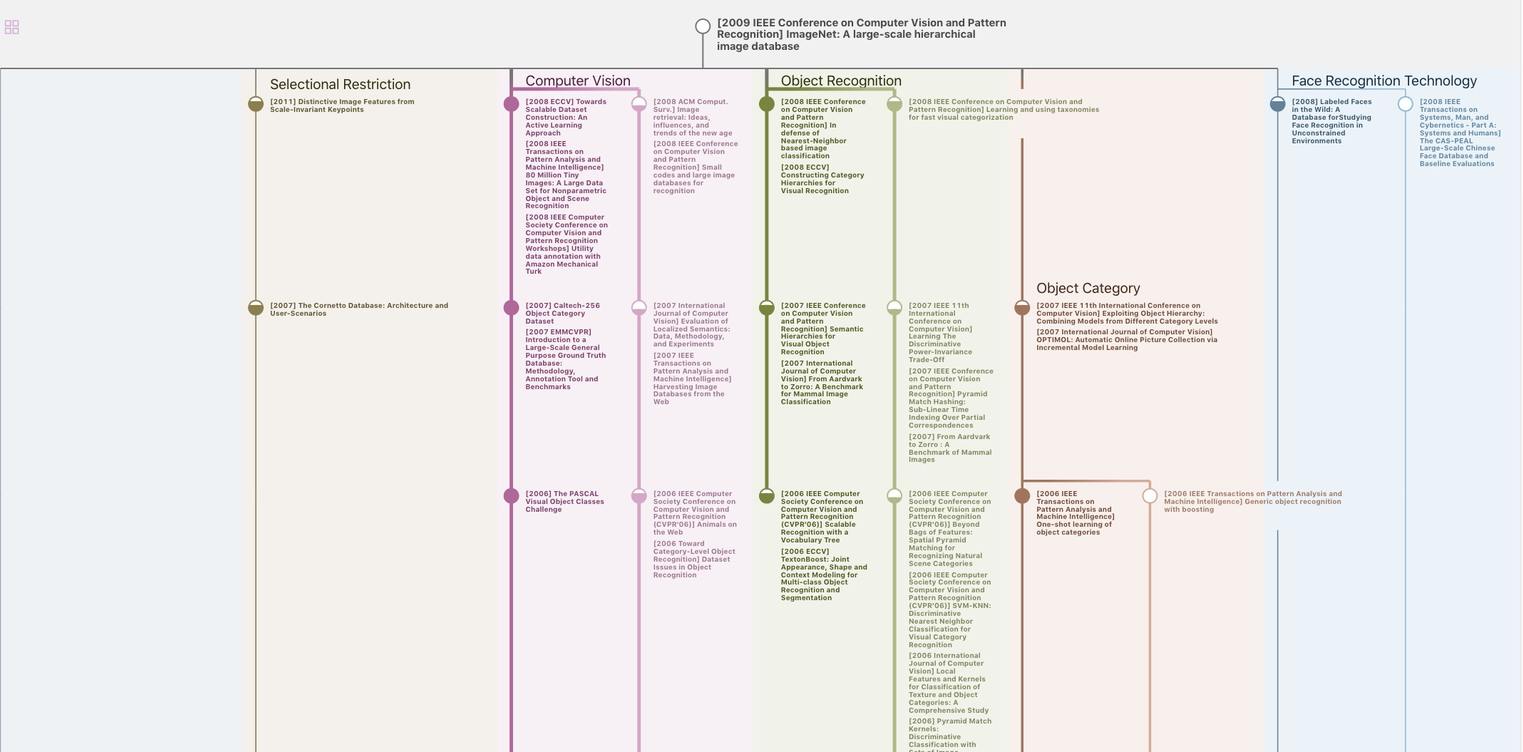
生成溯源树,研究论文发展脉络
Chat Paper
正在生成论文摘要