Multi-Level Feature Representation Framework With Adaptive Margin Loss for Few-Shot Sonar Images Classification of AUVs.
IEEE Transactions on Intelligent Vehicles(2024)
Abstract
Autonomous underwater target detection is vital for enhancing the autonomy and intelligence of Autonomous Underwater Vehicles (AUVs). The main challenges in sonar image classification are insufficient underwater target samples, underwater target diversity, and the interference of noise. Most current underwater target classification methods rely on large-scale datasets and can only deal with specific categories or scenarios. To address this issue, this paper proposes a general framework (FS-UTnet) for underwater targets detection with few-shot samples, based on multi-scale local feature representation and adaptive margin. First, the multi-scale local feature representation is designed to preserve more discriminative information for each category. Then, the discriminative ability of reducing the intra-class distance while increasing inter-class distance is effectively enhanced by constructing adaptive margin loss. Real sea trial experiments were conducted to construct an underwater targets dataset based on side-scan sonar (FSL-SSS) for training and testing. Experimental results show that our proposed method outperforms several state-of-the-art techniques with very few labeled samples, achieving classification accuracies of 80.66% for the 5-way 5-shot scenario and 84.81% for the 5-way 10-shot scenario. This method exhibits remarkable capability in generalizing new detection tasks of AUV with few samples across different underwater conditions.
MoreTranslated text
Key words
Autonomous Underwater Vehicle,Underwater target detection,Side-scan sonar (SSS),few-shot learning
AI Read Science
Must-Reading Tree
Example
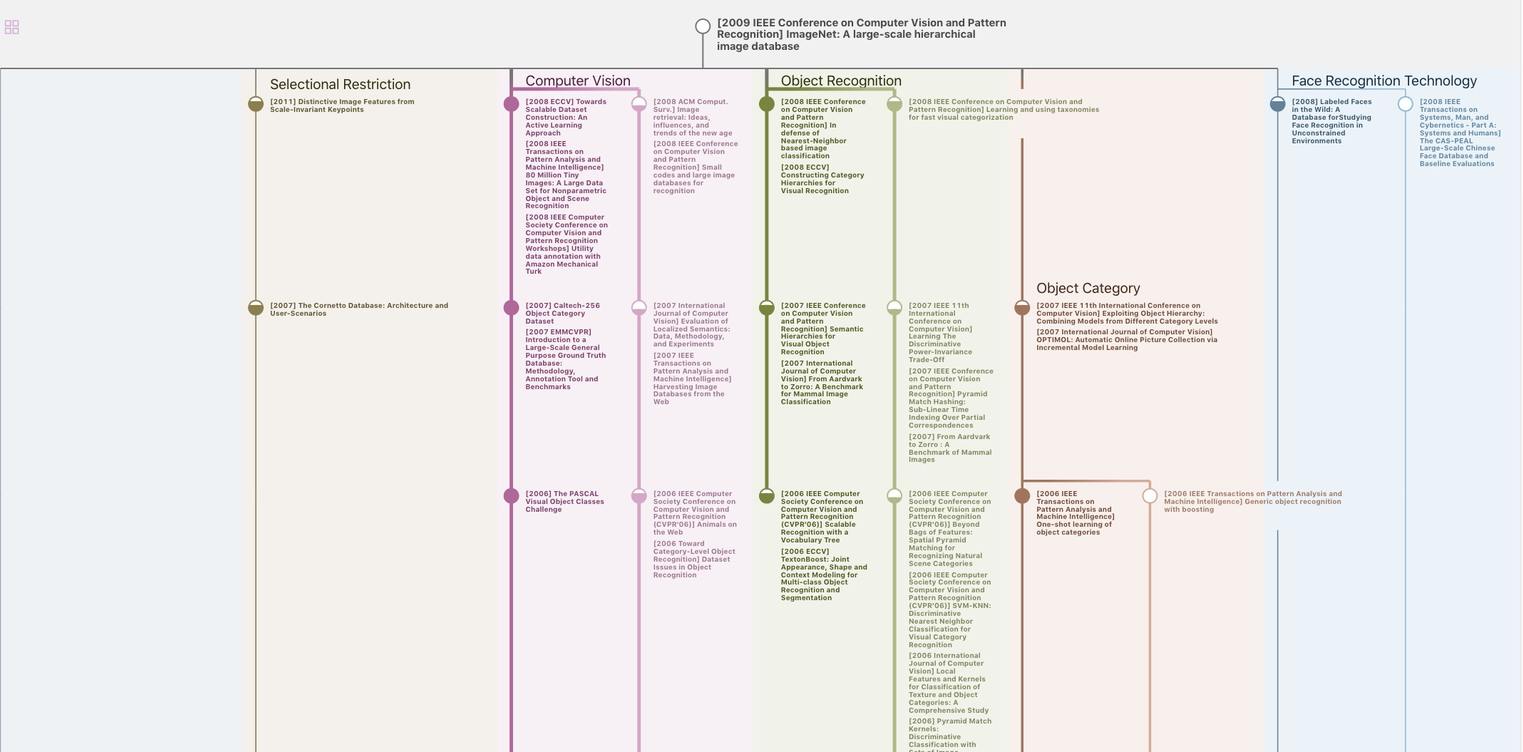
Generate MRT to find the research sequence of this paper
Chat Paper
Summary is being generated by the instructions you defined