Enhancing security in brain computer interface applications with deep learning: Wavelet transformed electroencephalogram-based user identification
Expert Systems with Applications(2024)
摘要
Electroencephalogram (EEG) signals have gained widespread use in medical applications, and the utilization of EEG signals as a biometric feature for user identification systems in brain-computer interface systems has recently garnered significant attention. This paper presents a deep learning framework that uses a deep residual neural network (ResNet) for the identification of distinct individuals based on their EEG signals. The proposed framework utilizes continuous wavelet transform to convert one-dimensional EEG signals into two-dimensional spatial images. By incorporating both the frequency and time characteristics of the EEG signals, this model effectively considers and analyses the intricate details present in the data, leading to improved performance. The ResNet model considers the interdependencies between different time instances of the EEG signals. These unique features, collectively, provide sufficient discriminative information to accurately identify individuals. The proposed method achieved an exceptional classification accuracy of 99.73% and an equal error rate of 0.0041 for 64 channels within 109 individuals. The results illustrate the superiority of the proposed method over existing approaches.
更多查看译文
关键词
Brain computer interface (BCI),Electroencephalogram (EEG),Biometric,User identification,Residual neural network (ResNet),Wavelet transform
AI 理解论文
溯源树
样例
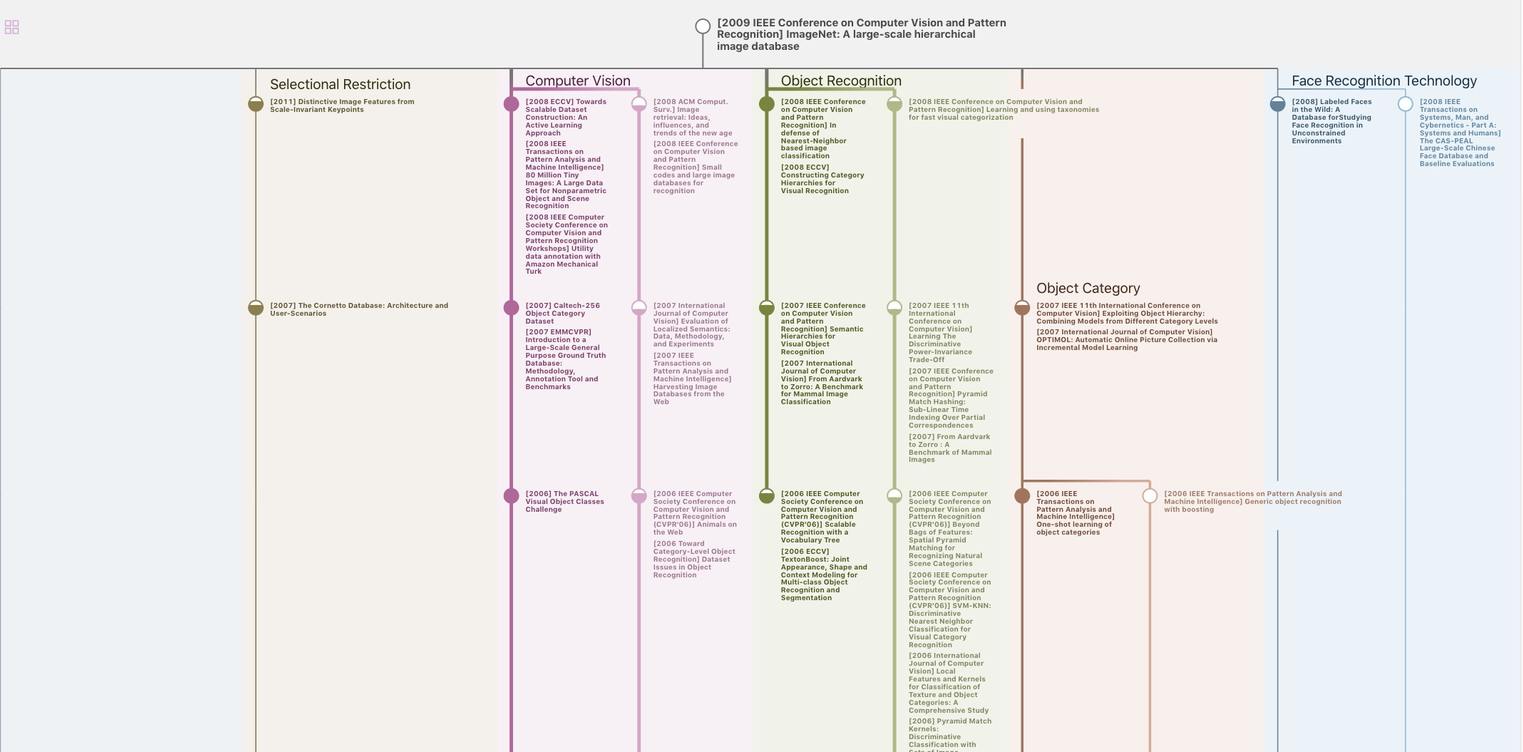
生成溯源树,研究论文发展脉络
Chat Paper
正在生成论文摘要