Compositional Design of Multicomponent Alloys using Reinforcement Learning
Acta Materialia(2024)
摘要
The design of alloys has typically involved adaptive experimental synthesis and characterization guided by machine learning models fitted to available data. A bottleneck for sequential design, be it for self-driven or manual synthesis, by Bayesian Global Optimization (BGO) for example, is that the search space becomes intractable as the number of alloy elements and its compositions exceed a threshold. Here we investigate how reinforcement learning (RL) performs in the compositional design of alloys within a closed loop with manual synthesis and characterization. We demonstrate this strategy by designing a phase change multicomponent alloy (Ti27.2Ni47Hf13.8Zr12) with the highest transformation enthalpy (ΔH) -37.1 J/g (-39.0 J/g with further calibration) within the TiNi-based family of alloys from a space of over 2 × 108 candidates, although the initial training is only on a compact dataset of 112 alloys. We show how the training efficiency is increased by employing acquisition functions containing uncertainties, such as expected improvement (EI), as the reward itself. Existing alloy data is often limited, however, if the agent is pretrained on experimental results prior to the training process, it can access regions of higher reward values more frequently. In addition, the experimental feedback enables the agent to gradually explore new regions with higher rewards, compositionally different from the initial dataset. Our approach directly applies to processing conditions where the actions would be performed in a given order. We also compare RL performance to BGO and the genetic algorithm on several test functions to gain insight on their relative strengths in materials design.
更多查看译文
关键词
Compositional design,Reinforcement learning,Multicomponent alloys,Transformational enthalpy,Phase change materials
AI 理解论文
溯源树
样例
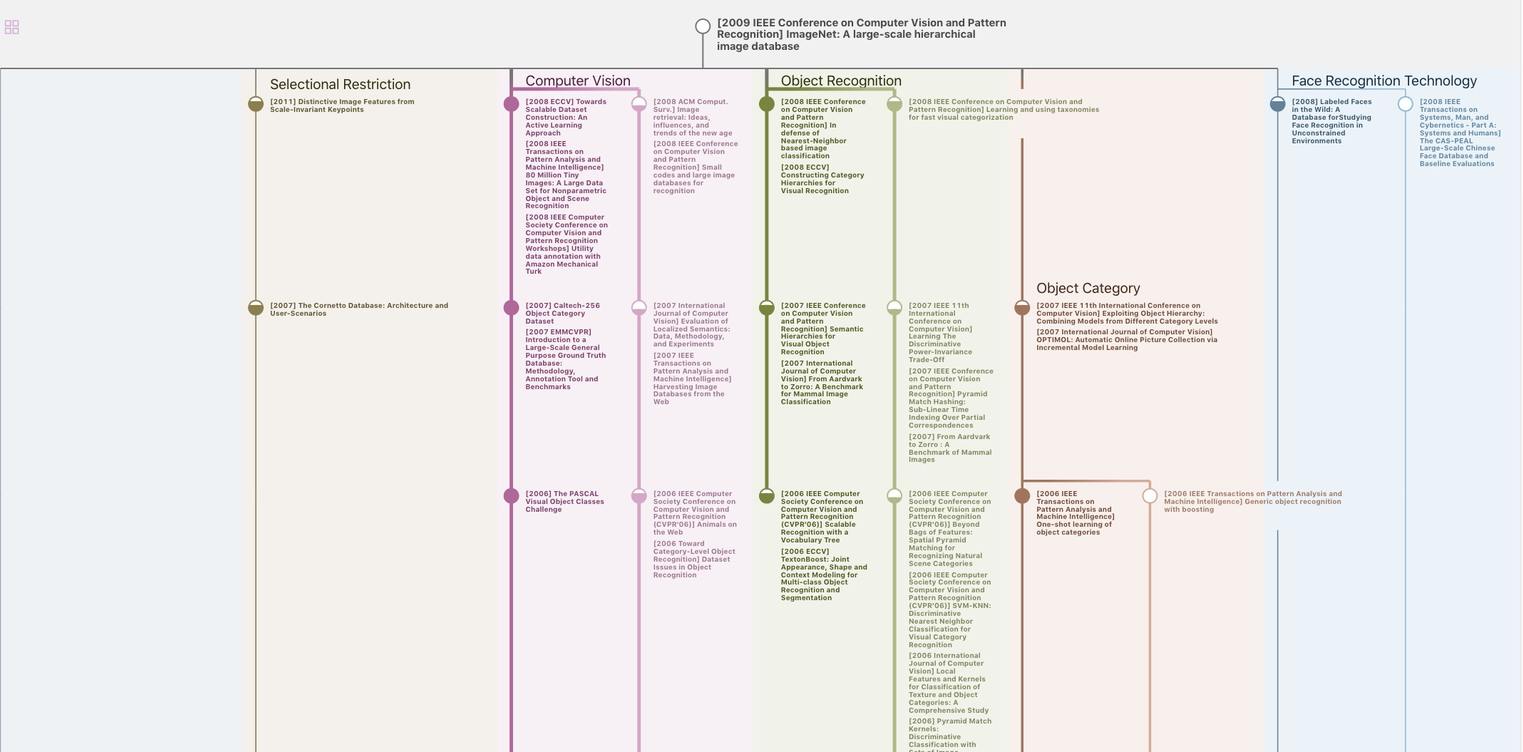
生成溯源树,研究论文发展脉络
Chat Paper
正在生成论文摘要