52.5 TOPS/W 1.7GHz Reconfigurable XGBoost Inference Accelerator Based on Modular-Unit-Tree with Dynamic Data and Compute Gating.
2024 IEEE Custom Integrated Circuits Conference (CICC)(2024)
Abstract
The XGBoost has emerged as a powerful AI algorithm achieving high accuracy winning multiple Kaggle competitions in many tasks including medical diagnosis, recommendation system, and autonomous driving [1]. It provides a great potential for edge devices due to a binary-tree-based simple computing kernel compared to deep learning [2]. Despite such a potential from the kernel-level simplicity, the efficient end-to-end realization is hindered by multiple design challenges due to 1) the highly irregular tree shape, 2) low hardware utilization, 3) delay from the sequential processing of each tree node, and 4) a large data movement to all nodes [3]–[5]. We propose low-power and high performance XGBoost accelerator by employing modular unit trees and reconfigurable interconnects along with a selective data movement and execution. The proposed accelerator achieves 52.5 TOPS/Wand 0.41
$\text{TOPS}/\text{mm}^{2}$
, which are the best among the reported CNN and tree-based classifiers [2]–[5].
MoreTranslated text
Key words
XGBoost,Input Features,Light Signal,Lookup Table,Load Data,Leaf Node,Recommender Systems,Multiple Trees,High Energy Cost,Tree Shape,Modular Units,Tree-based Classifiers,Tree Architecture,Kernel Computation,Output Gain,CNN Classifier
AI Read Science
Must-Reading Tree
Example
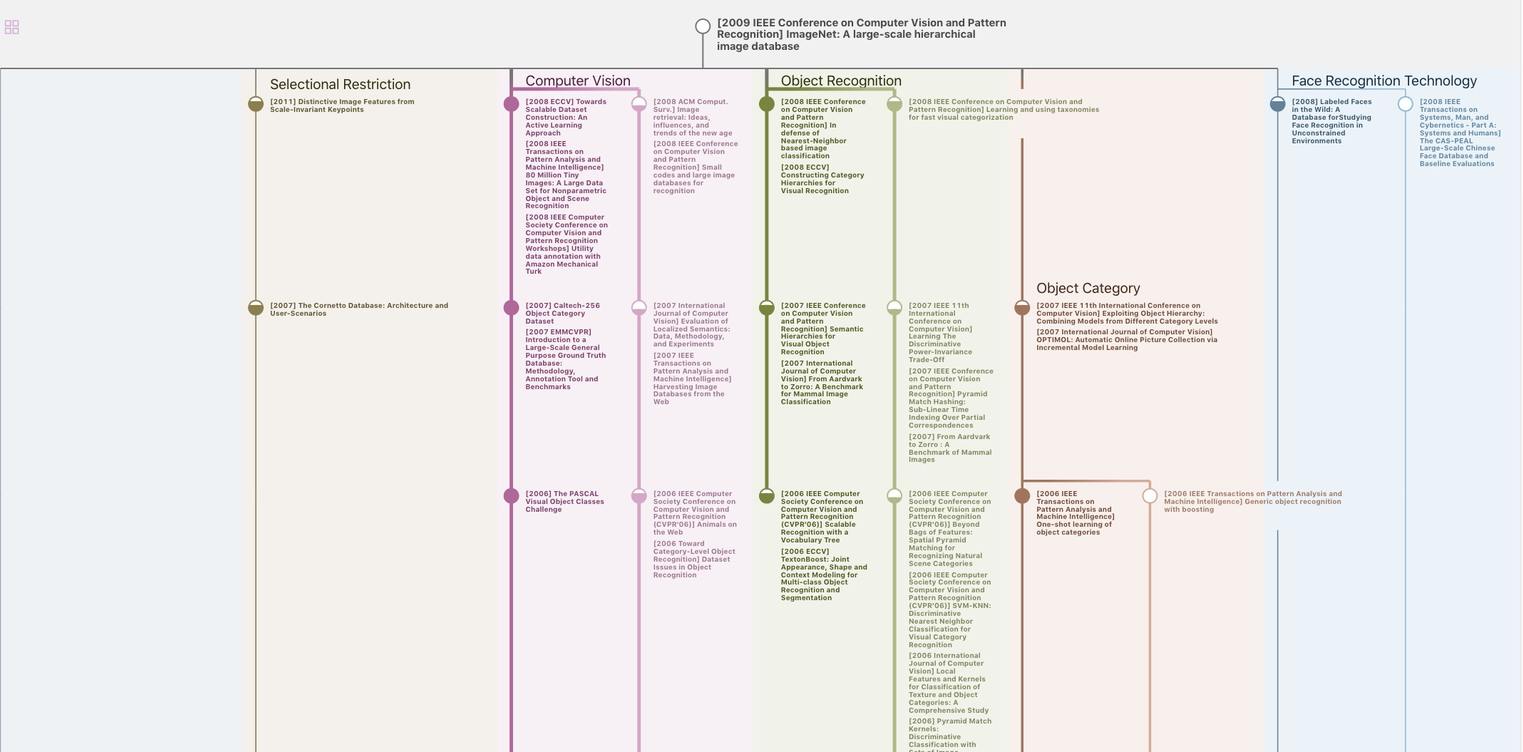
Generate MRT to find the research sequence of this paper
Chat Paper
Summary is being generated by the instructions you defined