Unmasking Efficiency: Learning Salient Sparse Models in Non-IID Federated Learning
CoRR(2024)
摘要
In this work, we propose Salient Sparse Federated Learning (SSFL), a
streamlined approach for sparse federated learning with efficient
communication. SSFL identifies a sparse subnetwork prior to training,
leveraging parameter saliency scores computed separately on local client data
in non-IID scenarios, and then aggregated, to determine a global mask. Only the
sparse model weights are communicated each round between the clients and the
server. We validate SSFL's effectiveness using standard non-IID benchmarks,
noting marked improvements in the sparsity–accuracy trade-offs. Finally, we
deploy our method in a real-world federated learning framework and report
improvement in communication time.
更多查看译文
AI 理解论文
溯源树
样例
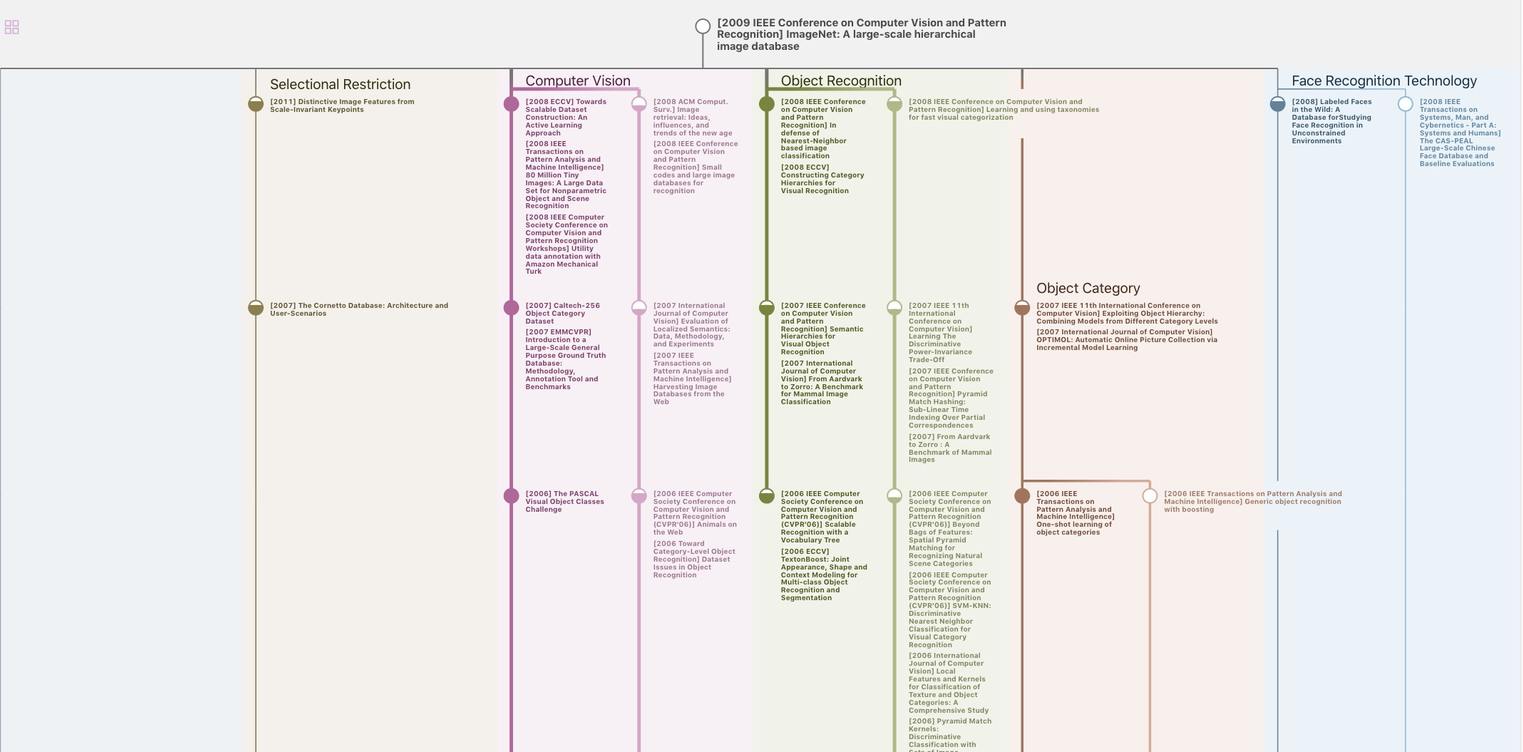
生成溯源树,研究论文发展脉络
Chat Paper
正在生成论文摘要