Causality Analysis and Prediction of Riverine Algal Blooms by Combining Empirical Dynamic Modeling and Machine Learning Techniques
WATER RESOURCES RESEARCH(2024)
Abstract
River algal blooms have become a global environmental problem due to their large impact range and environmental hazards. However, the complex mechanisms underlying these blooms make prediction and prevention challenging. Here, we employed empirical dynamic modeling (EDM) and machine learning to reveal the causes and predict diatom blooms from 2003 to 2017 in the Han River of China. The diatom cell density ranged from 0.1 to 5.1 x 10(7) cells L-1, whereas algal blooms often lasted for 10 days with density exceeding 10(7) cells L-1. The EDM results elucidated that, under consistent high nutrient concentrations, algal blooms were primarily regulated by eight environmental factors: water temperature in the Han River; water levels, flow velocities, and streamflow discharges in the Han River and the Yangtze River; and water level variation in the Han River. The poor performance (coefficient of determination R-2 < 0) of the multiple linear regression, EDM, and random forest models indicated the challenge of predicting algae density. Therefore, we used machine learning classification models to predict algal blooms occurrences. With the resampling techniques to account for imbalanced data, machine learning models achieved perfect classification prediction (Kappa value = 1) of the 13 algae-bloom events with a 10-day lead time during a 15-year period, providing an important reference for preemptive warning of riverine algal blooms. Plain Language Summary River algal blooms have become a global environmental problem due to their large impact range and environmental hazards. The Han River is one of the most frequent areas of river algal blooms in China, but there is no clear conclusion on the causes of algal blooms formation. In this study, we combined two methods to reveal the causes and predict the occurrence of algal blooms in the Han River. Our results show that the prediction of algal blooms in the Han River is feasible. These blooms are primarily affected by the water temperature and seven other hydrological variables in the Han and Yangtze River. This study provides a starting point for the preemptive warning of riverine algal blooms.
MoreTranslated text
Key words
river algal bloom,empirical dynamic modeling,convergent cross-mapping,machine learning,resampling technique
AI Read Science
Must-Reading Tree
Example
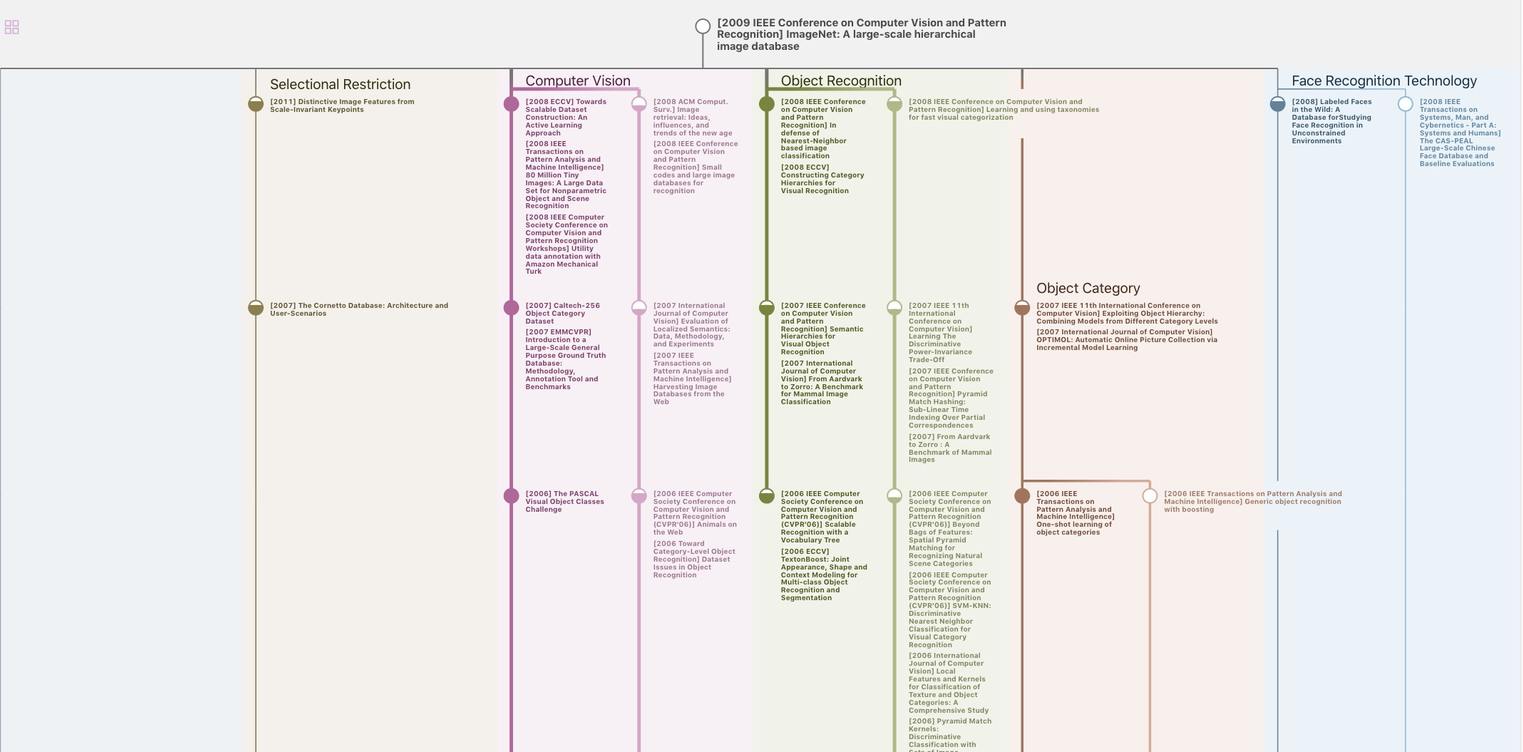
Generate MRT to find the research sequence of this paper
Chat Paper
Summary is being generated by the instructions you defined