Parameter-setting heuristic for the quantum alternating operator ansatz
PHYSICAL REVIEW RESEARCH(2024)
Abstract
The quantum alternating operator ansatz (QAOA) is a generalized approach for solving challenging optimization problems that builds on the alternating structure of the quantum approximate optimization algorithm. Finding high-quality parameters efficiently for QAOA remains a major challenge in practice. In this work, we introduce a classical strategy for parameter setting, suitable for cases in which the number of distinct cost values grows only polynomially with the problem size, such as is common for constraint-satisfaction problems. The crux of our strategy is that we replace the cost function expectation value step of QAOA with a classical model that can be efficiently evaluated classically and has parameters which play an analogous role to the QAOA parameters. This model is based on empirical observations that, in some QAOA states, variable configurations with the same cost have the same amplitudes from step to step. We define this class of states as homogeneous states. For problems with particular symmetries, QAOA states are guaranteed to be homogeneous. More generally, high overlaps between QAOA states and homogeneous states have been empirically observed in a number of settings. Building on this idea, we define a classical homogeneous proxy for QAOA in which this property holds exactly and which yields information describing both states and expectation values. We then classically determine high-quality parameters for this proxy and then use these parameters in QAOA, an approach we label the homogeneous heuristic for parameter setting. We numerically examine this heuristic for MaxCut on unweighted Erdos-Renyi random graphs. For up to three QAOA levels we demonstrate that the heuristic is easily able to find parameters that match approximation ratios corresponding to previously found globally optimized approaches. For levels up to 20 we obtain parameters using our heuristic with approximation ratios monotonically increasing with depth, while a strategy that uses parameter transfer instead fails to converge with comparable classical resources. These results suggest that our heuristic may find good parameters in regimes that are intractable with noisy intermediate-scale quantum devices. Finally, we outline how our heuristic may be applied to wider classes of problems.
MoreTranslated text
AI Read Science
Must-Reading Tree
Example
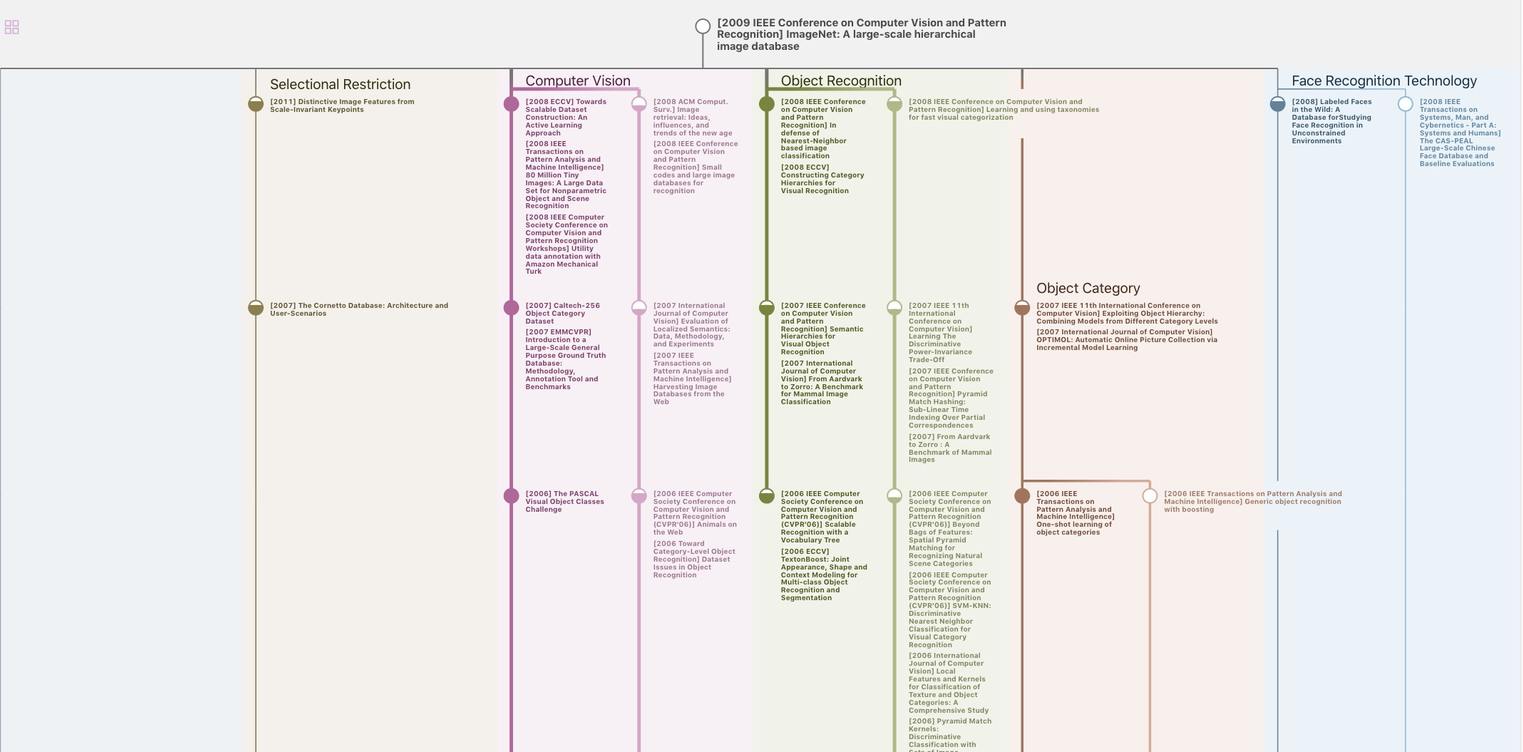
Generate MRT to find the research sequence of this paper
Chat Paper
Summary is being generated by the instructions you defined