Novel Deep-Learning Method Based on LSA-Transformer for Fault Detection and Its Implementation in Penicillin Fermentation Process
MEASUREMENT(2024)
关键词
LSA-Transformer model,Fault detection,Penicillin fermentation process,Confusion matrix,Heatmap
AI 理解论文
溯源树
样例
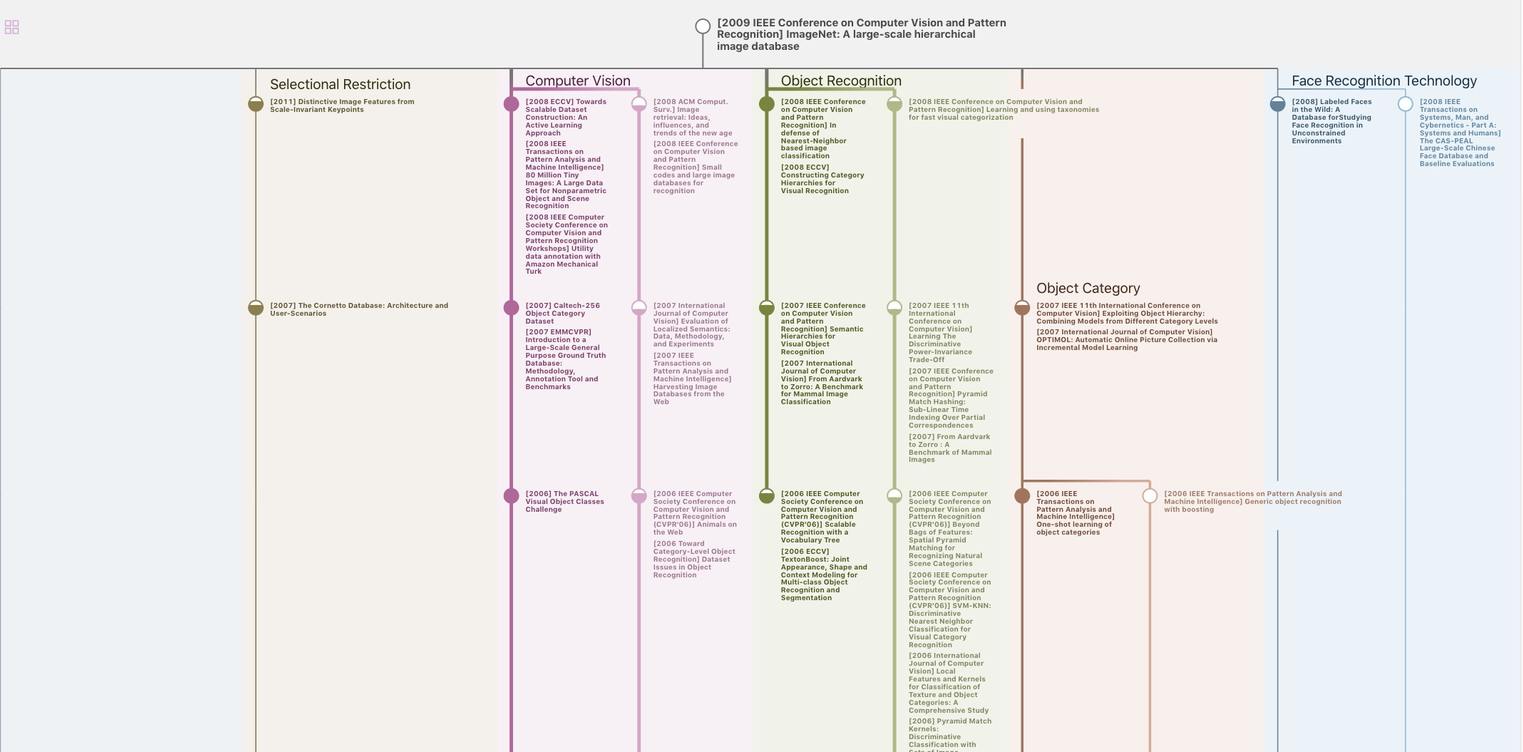
生成溯源树,研究论文发展脉络
Chat Paper
正在生成论文摘要