Monitoring and prediction of the spongy moth (Lymantria dispar) outbreaks in Mountain’s landscape using a combination of Sentinel-2 images and nonlinear time series model
Forest Ecology and Management(2024)
Abstract
Lymantria dispar (Lepidoptera: Erebidae) is a significant defoliator both in its native and introduced regions. To better monitor and predict outbreaks for appropriate management of L. dispar, we estimated the defoliation distribution by L. dispar and performed nonlinear time-series forecasting of the vegetation anomaly dynamics. The Normalized Difference Vegetation Index (NDVI) obtained from Sentinel-2 was utilized to detect defoliation of the forests in the Altai Republic of Western Siberia. During the L. dispar outbreak in 2017, forest defoliation was captured by at two sites. The difference between NDVI in defoliated sites and seasonal NDVI was compared to characterize the NDVI dynamics of L. dispar defoliation. Results show that the NDVI of the two defoliated sites decreased by −0.101 and −0.087 during the season, respectively. The spatial distribution (100 m resolution) of the defoliation using NDVI showed a decreasing trend from 2017 to 2021. It showed heavy defoliation with a maximum of 10 % of the forest area from 2017 to 2018, in contrast to forest recovery in subsequent years. The defoliation was influenced by topographic factors, which occurred at relatively low elevations (916–1466 m) along roadways and open slopes. The NDVI model was built with dynamic mode decomposition (DMD) to represent the spatiotemporal dynamics of normal vegetation changes. The negative deviations between observed NDVI and the DMD-predicted NDVI then indicated anomalous vegetation changes such as defoliation by L. dispar. The study area's vegetation anomaly dynamics were forecasted using the local manifold distance-based regression (LMDr), a nonlinear forecasting technique that employs the state space reconstruction technique. The nonlinear time-series model demonstrated the best forecasting skill (ρ = 0.612) with the embedding (time-delay) dimension E = 11, suggesting the potential for anomaly detection and outbreak forecasting by the model. The study provided a good monitoring and forecasting framework for L. dispar defoliation in native ranges by combining a high-resolution imagery dataset with nonlinear anomaly detection.
MoreTranslated text
Key words
Forest pests,Defoliation models,Sentinel mission,Gypsy moth,NDVI temporal series
AI Read Science
Must-Reading Tree
Example
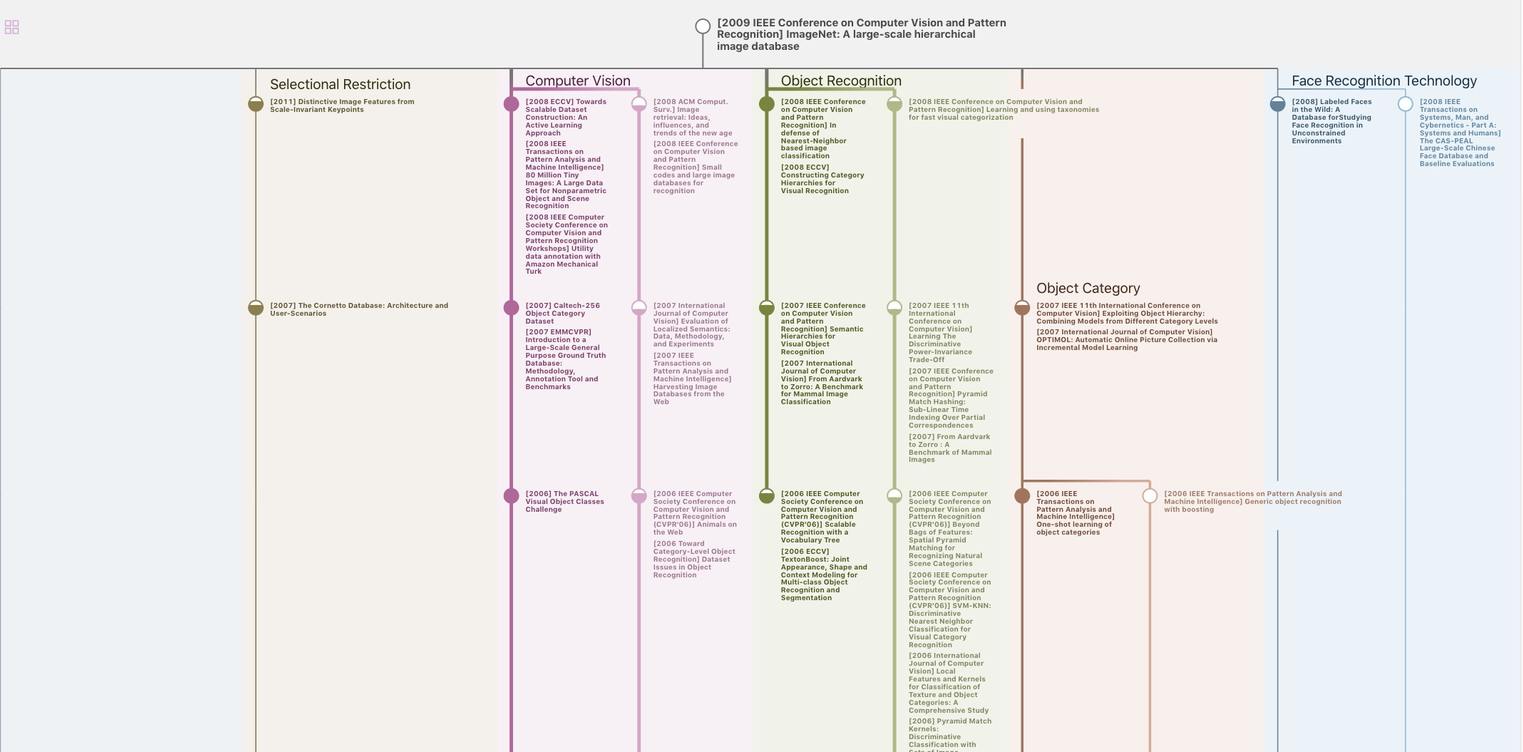
Generate MRT to find the research sequence of this paper
Chat Paper
Summary is being generated by the instructions you defined