Deep nested algorithm unrolling learning for inverse problems with its application in electrical capacitance tomography
Engineering Applications of Artificial Intelligence(2024)
Abstract
Electrical capacitance tomography stands out as a promising measurement modality but is limited by low-quality images. To alleviate this challenge, this study establishes a new bilevel optimization imaging model, composed of an upper level optimization problem and a lower level optimization problem, which achieves autonomous tuning of the regularization parameter. The new bilevel optimization imaging model is reduced into a single-level optimization problem by the algorithm unrolling technique, which is further solved by the particle swarm optimization algorithm. Based on the learned regularization parameters and the given capacitance data, a new lower level optimization problem that integrates the measurement physics, learned image prior and sparsity-induced prior is solved for reconstruction. A new physics-informed broad learning system is developed to predict image priors, and a novel optimization model is built for training by introducing measurement physics to prevent predictions from deviating from fundamental physical significance. A new optimizer that allows the training model to be solved in a more effective way is proposed. Comparisons with other popular reconstruction algorithms confirm that the new bilevel optimization imaging method achieves significant improvements in reconstruction accuracy and noise immunity. This study provides a promising roadmap to improve imaging quality by unlocking the synergistic potential between collected data and measurement physics.
MoreTranslated text
Key words
Imaging method,Learned image prior,Physics-informed broad learning system,Bilevel optimization,Algorithm unrolling learning,Electrical capacitance tomography
AI Read Science
Must-Reading Tree
Example
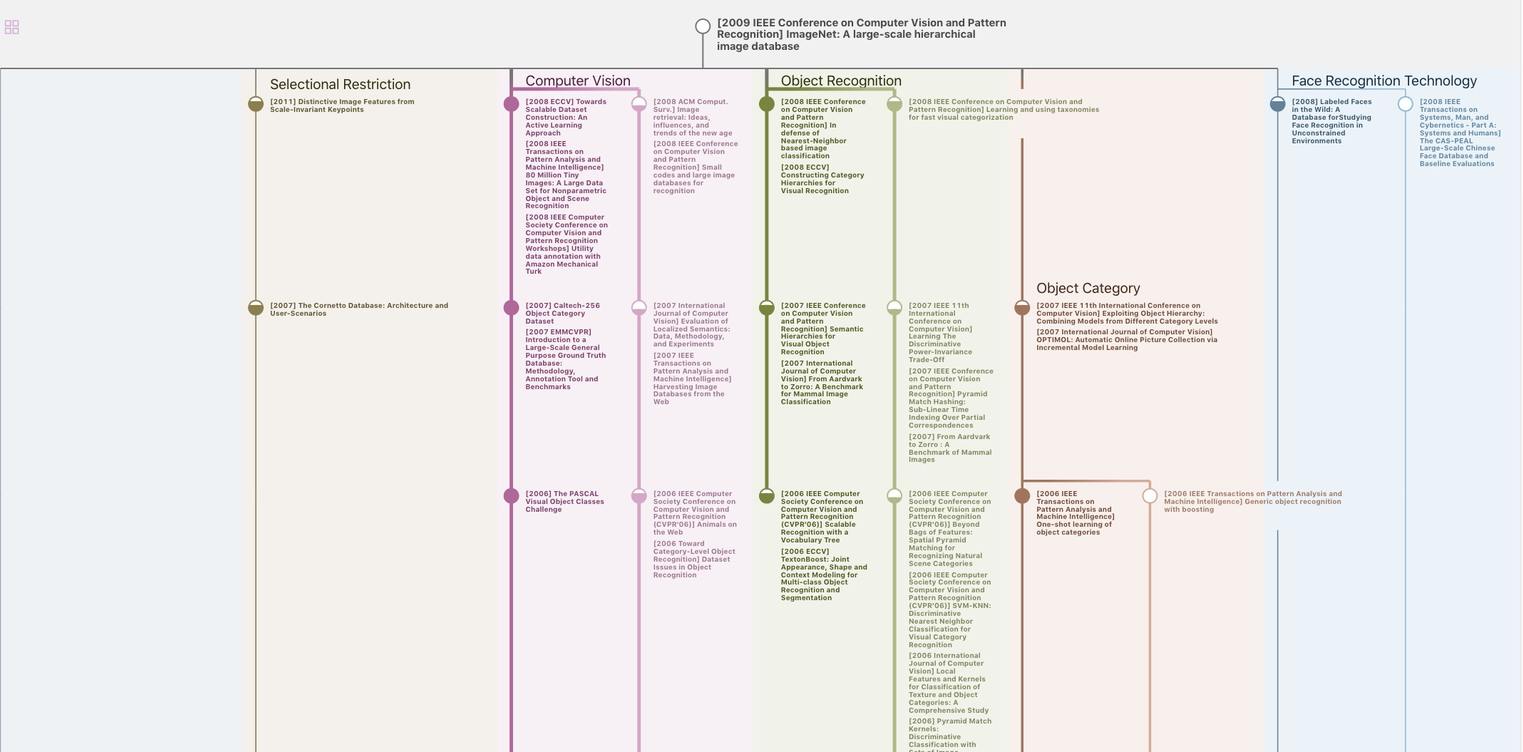
Generate MRT to find the research sequence of this paper
Chat Paper
Summary is being generated by the instructions you defined