Commensal or pathogen: computationally vectorizing microbial genomes for de novo risk assessment and virulence feature discovery in Klebsiella pneumoniae
crossref(2024)
摘要
Bacterial pathogenicity has traditionally focused on gene-level content with experimentally-confirmed functional properties. Hence, significant inferences are made based on similarity to known pathotypes and DNA-based genomic subtyping for risk. Herein, we achieved de novo prediction of human virulence in Klebsiella pneumoniae by expanding known virulence genes with spatially proximal gene discoveries linked by functional domain architectures across all prokaryotes. This approach identified gene ontology functions not typically associated with virulence sensu stricto . By leveraging machine learning models with these expanded discoveries, public genomes were assessed for virulence prediction using categorizations derived from isolation sources captured in available metadata. Performance for de novo strain-level virulence prediction achieved 0.81 F1-Score. Virulence predictions using expanded “discovered” functional genetic content were superior to that restricted to extant virulence database content. Additionally, this approach highlighted the incongruence in relying on traditional phylogenetic subtyping for categorical inferences. Our approach represents an improved deconstruction of genome-scale datasets for functional predictions and risk assessment intended to advance public health surveillance of emerging pathogens.
### Competing Interest Statement
The authors have declared no competing interest.
更多查看译文
AI 理解论文
溯源树
样例
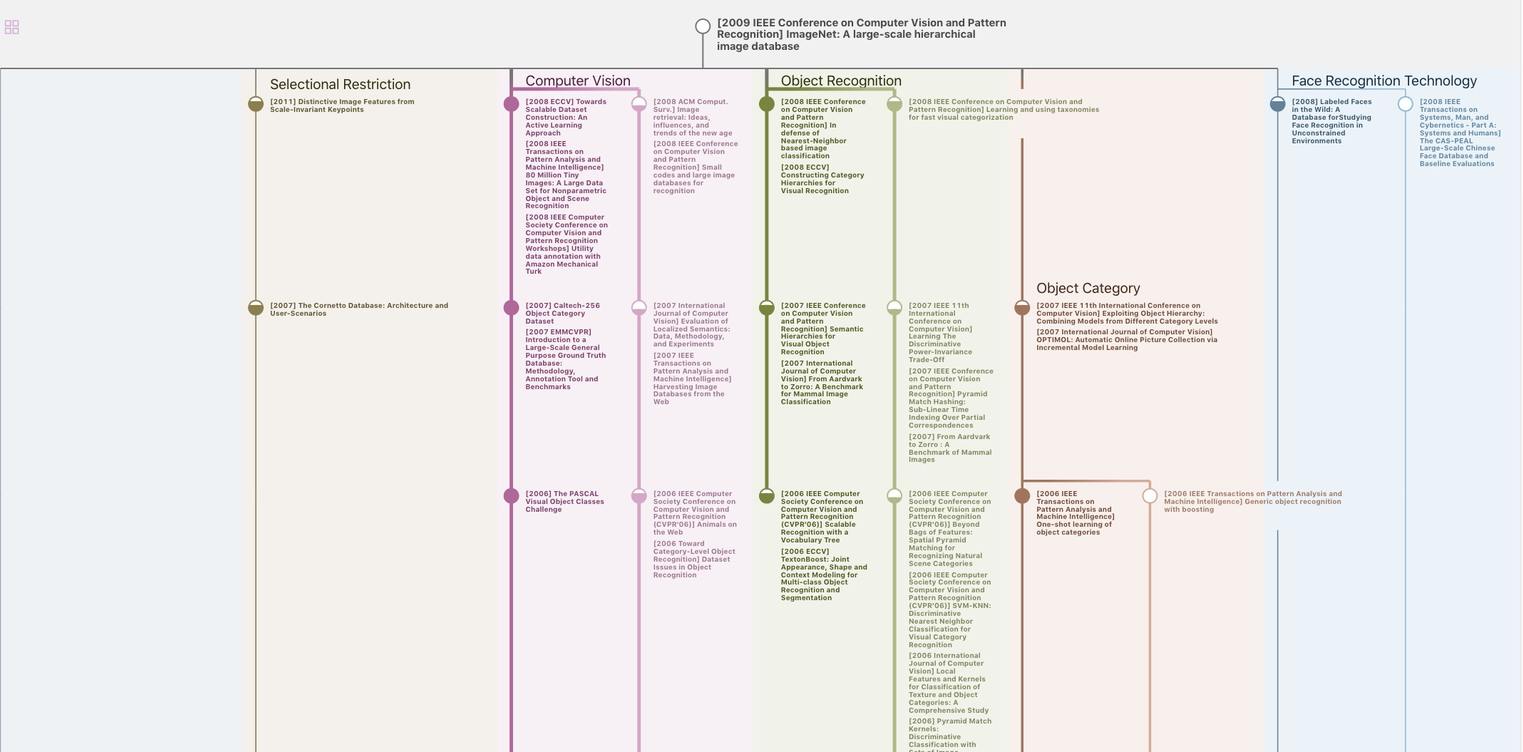
生成溯源树,研究论文发展脉络
Chat Paper
正在生成论文摘要