GETRF: A General Framework for Encrypted Traffic Identification With Robust Representation Based on Datagram Structure
IEEE Transactions on Cognitive Communications and Networking(2024)
Abstract
Identifying encrypted traffic is essential for network management, but it becomes increasingly challenging as more and more users adopt encryption protocols and traffic defense mechanisms for data privacy. Most existing methods heavily rely on deep features and labeled samples, overlooking the opportunity to capture pattern information of datagram structure. As a consequence, these methods are unable to extract rule-based knowledge from unlabeled data, limiting their ability to identify unseen or feature-perturbed traffic. In this paper, we propose a new framework for encrypted traffic identification named Generic Encrypted Traffic Representation Framework (GETRF), which includes three pre-training tasks designed based on the datagram structure. By leveraging the advantages of these tasks, GETRF can utilize unlabeled traffic to form generic and robust representations, capturing the characteristics of datagrams at the byte, packet, and flow levels. After fine-tuning, these representations can be applied to identification tasks across multiple scenarios. The results demonstrate that GETRF achieves state-of-the-art performance in multi-scenario and fine-grained identifications, and effectively handles feature-perturbed traffic.
MoreTranslated text
Key words
Encrypted traffic identification,Pre-training,Generic traffic representation,Cyberspace management,Datagram structure,Privacy-enhancing technology
AI Read Science
Must-Reading Tree
Example
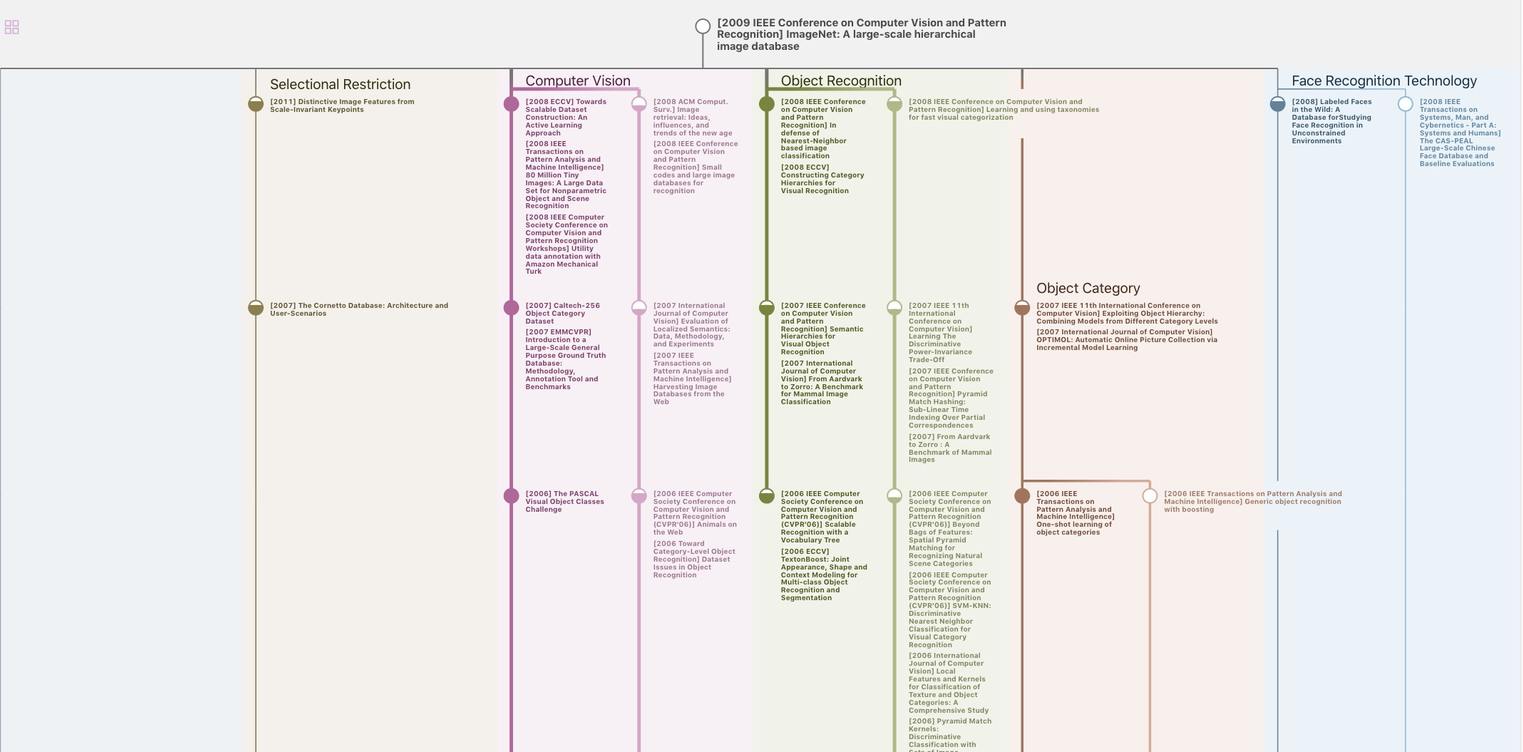
Generate MRT to find the research sequence of this paper
Chat Paper
Summary is being generated by the instructions you defined