Federated Learning CNN for Broccoli Leaf Disease Control: A Path to Sustainable Farming
2024 11th International Conference on Reliability, Infocom Technologies and Optimization (Trends and Future Directions) (ICRITO)(2024)
Abstract
A federated learning approach using convolutional neural networks (CNNs) for broccoli leaves' disease classification is described in our study. Here are a few highlights from the article. Using input from five varied clients, each representing a different cultivation scenario, we tackle the difficult task of classifying five separate classes of broccoli leaf diseases: healthy leaves, downy mildew, Alternaria leaf spot, black rot, and ring spot. On average, our federated learning CNN model reached an accuracy of 94.02%, recall of 94.54%, and F1-Score of 96.15%, proving its effectiveness. These results show that our federated learning model was able to provide an accurate classification of the five classes of broccoli leaf diseases. The model was able to identify standard features such as color, size, and shape to differentiate different courses. The weighted average, considering client performance and dataset sizes, equates to an F1-Score of 96.20%, a recall value of 94.53%, and precision at the said percentile was rounded up. In addition, considering all the clients and data sizes within dataset management, it does not miss out on accuracy, which stands at 94.03%, recall that is put up for standardization at 94.52%, and an F1-Score of 96%. These comprehensive results demonstrate that our federated learning model consistently and robustly classifies broccoli leaf diseases through diverse cultivation scenarios. This reinforces the ability of federated learning to overcome agricultural issues without compromising data confidentiality.
MoreTranslated text
Key words
Broccoli leaves,Leaf diseases,(CNN)_(FL),Disease,Augmentation image,data privacy,data security
AI Read Science
Must-Reading Tree
Example
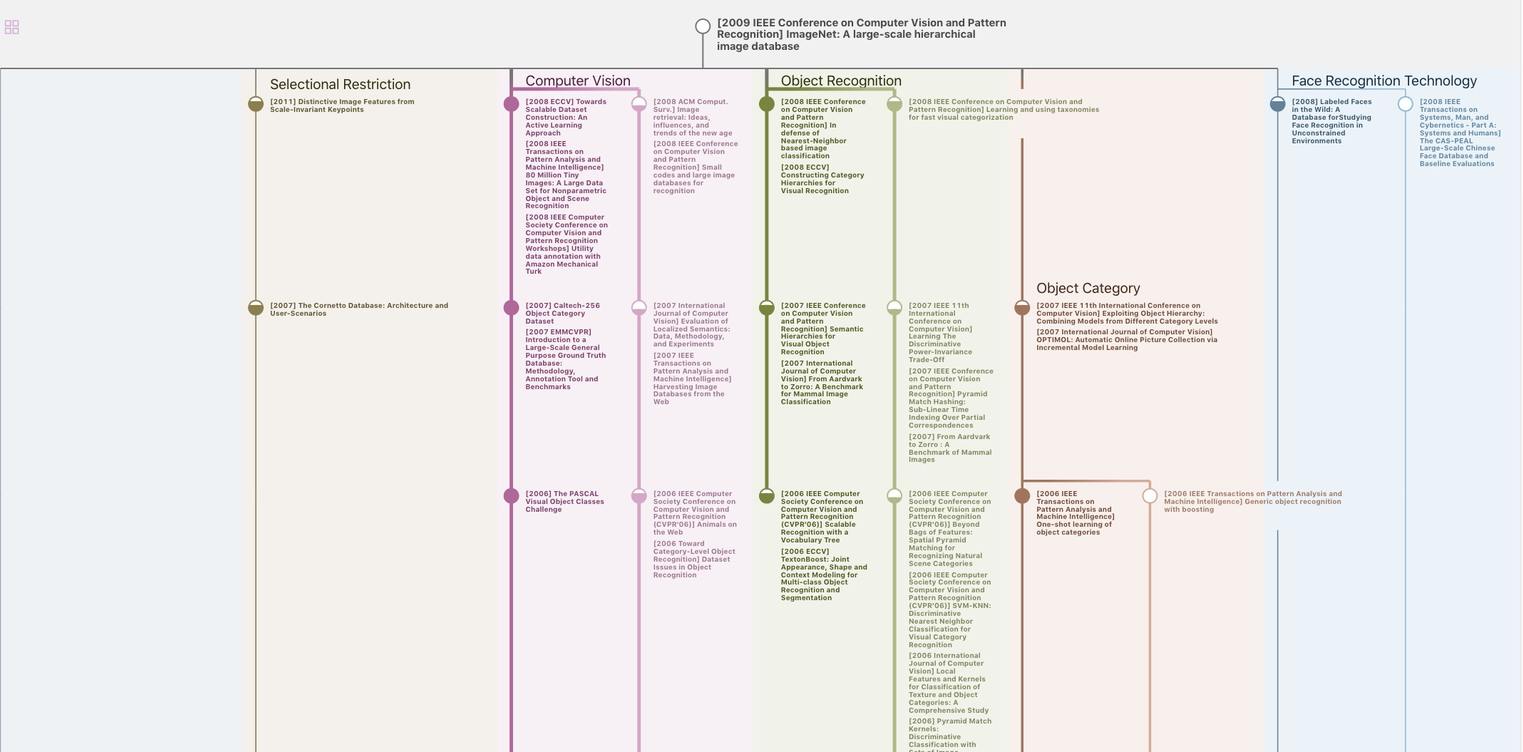
Generate MRT to find the research sequence of this paper
Chat Paper
Summary is being generated by the instructions you defined