Prediction of carbapenem-resistant gram-negative bacterial bloodstream infection in intensive care unit based on machine learning
BMC Medical Informatics and Decision Making(2024)
Abstract
Predicting whether Carbapenem-Resistant Gram-Negative Bacterial (CRGNB) cause bloodstream infection when giving advice may guide the use of antibiotics because it takes 2–5 days conventionally to return the results from doctor's order. It is a regional multi-center retrospective study in which patients with suspected bloodstream infections were divided into a positive and negative culture group. According to the positive results, patients were divided into the CRGNB group and other groups. We used the machine learning algorithm to predict whether the blood culture was positive and whether the pathogen was CRGNB once giving the order of blood culture. There were 952 patients with positive blood cultures, 418 patients in the CRGNB group, 534 in the non-CRGNB group, and 1422 with negative blood cultures. Mechanical ventilation, invasive catheterization, and carbapenem use history were the main high-risk factors for CRGNB bloodstream infection. The random forest model has the best prediction ability, with AUROC being 0.86, followed by the XGBoost prediction model in bloodstream infection prediction. In the CRGNB prediction model analysis, the SVM and random forest model have higher area under the receiver operating characteristic curves, which are 0.88 and 0.87, respectively. The machine learning algorithm can accurately predict the occurrence of ICU-acquired bloodstream infection and identify whether CRGNB causes it once giving the order of blood culture.
MoreTranslated text
Key words
Carbapenem-Resistant gram-negative bacteria,Bloodstream infection,Machine learning,Intensive care unit,Prediction model
AI Read Science
Must-Reading Tree
Example
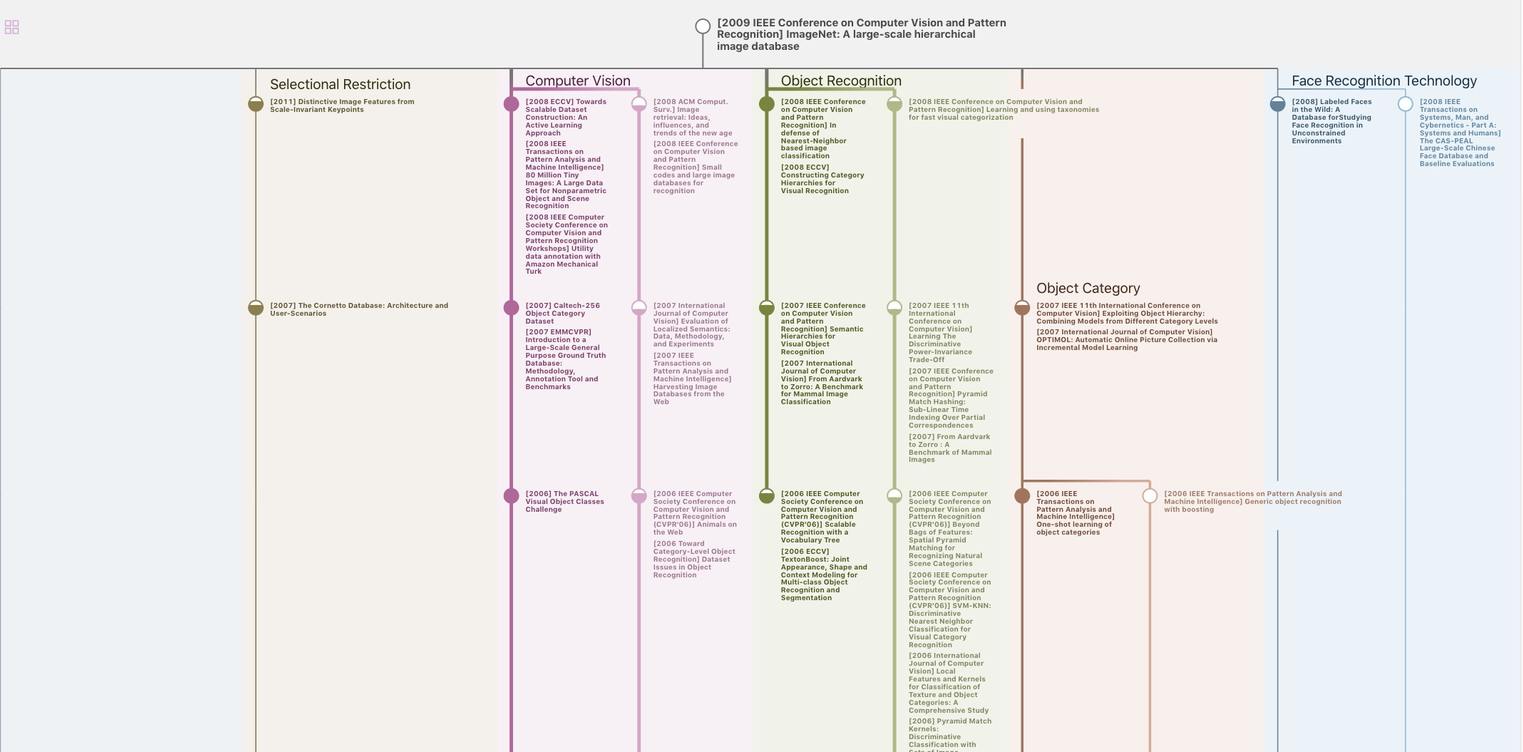
Generate MRT to find the research sequence of this paper
Chat Paper
Summary is being generated by the instructions you defined