Enhancing Reinforcement Learning in Sensor Fusion: A Comparative Analysis of Cubature and Sampling-based Integration Methods for Rover Search Planning
CoRR(2024)
Abstract
This study investigates the computational speed and accuracy of two numerical
integration methods, cubature and sampling-based, for integrating an integrand
over a 2D polygon. Using a group of rovers searching the Martian surface with a
limited sensor footprint as a test bed, the relative error and computational
time are compared as the area was subdivided to improve accuracy in the
sampling-based approach. The results show that the sampling-based approach
exhibits a 14.75% deviation in relative error compared to cubature when it
matches the computational performance at 100%. Furthermore, achieving a
relative error below 1% necessitates a 10000% increase in relative time
to calculate due to the 𝒪(N^2) complexity of the sampling-based
method. It is concluded that for enhancing reinforcement learning capabilities
and other high iteration algorithms, the cubature method is preferred over the
sampling-based method.
MoreTranslated text
AI Read Science
Must-Reading Tree
Example
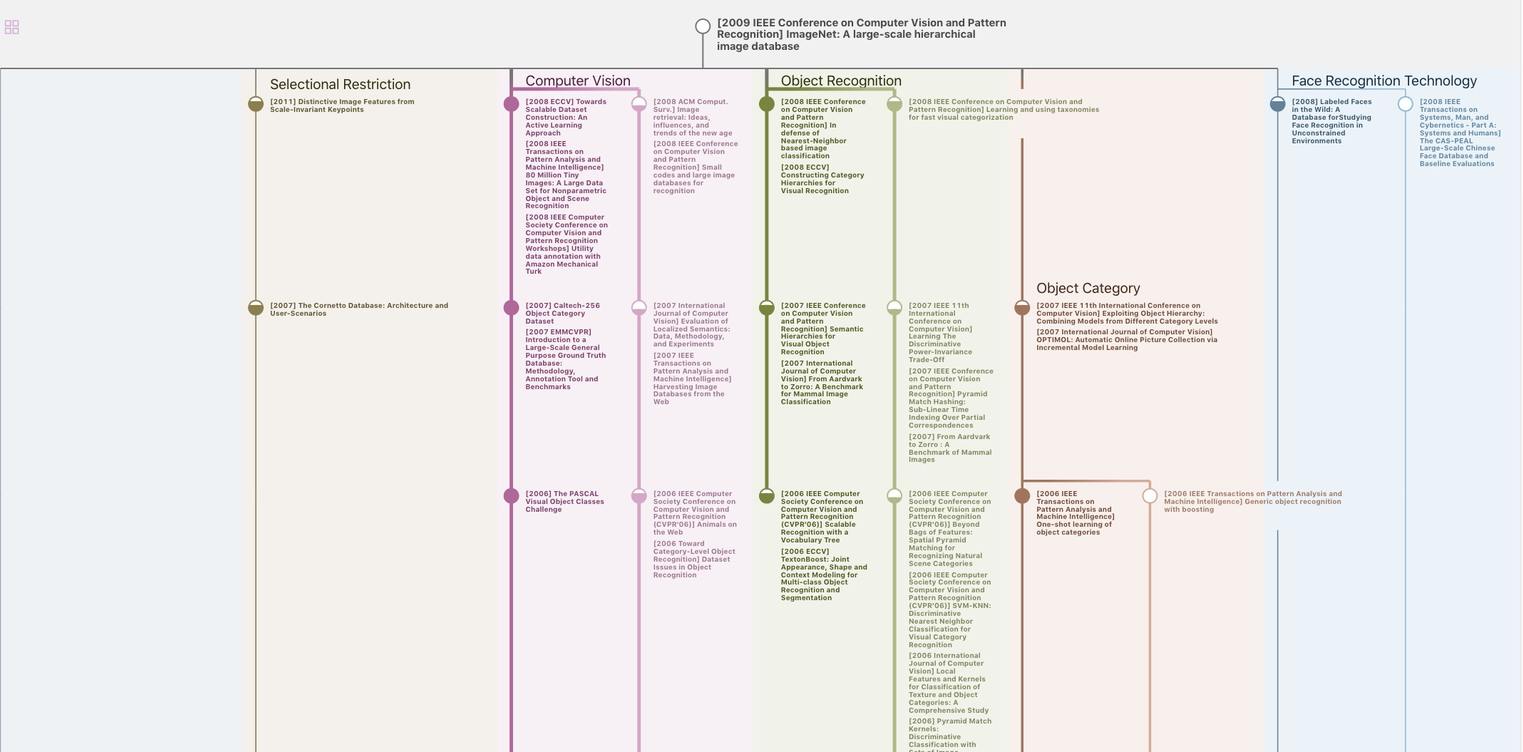
Generate MRT to find the research sequence of this paper
Chat Paper
Summary is being generated by the instructions you defined