Integrating Large Language Models and Machine Learning for Fake News Detection
2024 20th IEEE International Colloquium on Signal Processing & Its Applications (CSPA)(2024)
摘要
The increased fake news in today’s digital landscape necessitates effective detection methods to safeguard information integrity. While conventional machine learning, armed with features like text patterns, metadata, and user behavior, has made inroads in fake news detection, its heavy reliance on manually crafted features poses a challenge. This approach is time-consuming to capture the subtle complexities of fake news. Despite technological advancements, there remains a crucial research gap concerning utilizing emerging technologies like Large Language Models (LLMs), such as ChatGPT-3.5 and Bard, in fake news detection. This research addresses this gap by experimenting with and evaluating the potential of incorporating an LLM judgment using the ChatGPT-3.5 and conventional machine learning approaches in fake news detection. We tested various machine-learning models with the LLM. Out of these models, the hybrid XGBoost model emerges as a standout, boasting an accuracy of 96.39%, precision of 97.04%, recall of 98.17%, and an F1 score of 97.6%. This exceptional model performance could be attributed to the integration of ChatGPT-3.5’s judgments on the veracity of the news, demonstrating the importance of nuanced language patterns in distinguishing between authentic and fabricated news. These findings underline the potential of LLMs in revolutionizing fake news detection.
更多查看译文
关键词
Fake news detection,Large language model,Machine learning,Artificial intelligence,Deep learning
AI 理解论文
溯源树
样例
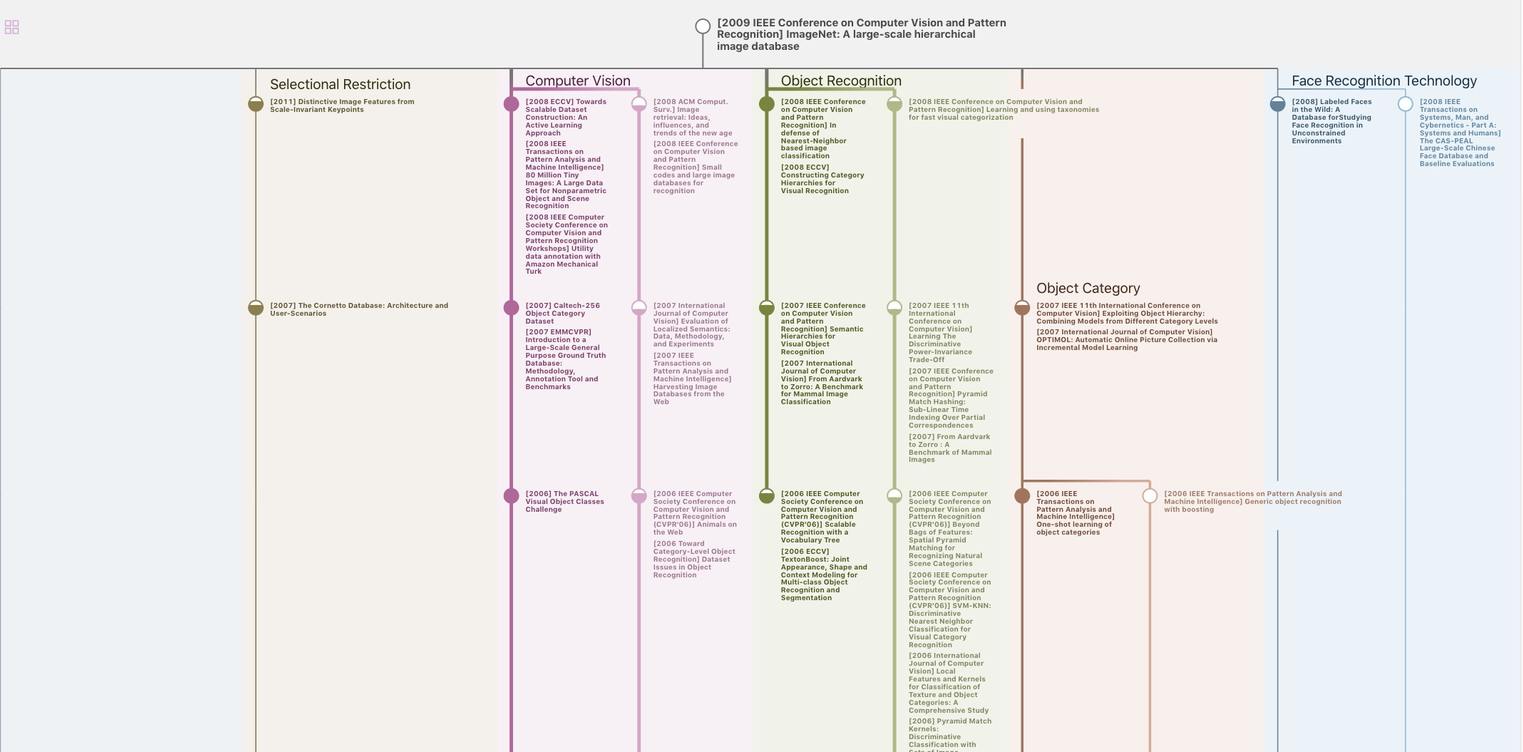
生成溯源树,研究论文发展脉络
Chat Paper
正在生成论文摘要