Algorithms for predicting COVID outcome using ready-to-use laboratorial and clinical data.
Frontiers in public health(2024)
摘要
The pandemic caused by severe acute respiratory syndrome coronavirus 2 (SARS-CoV-2) is an emerging crisis affecting the public health system. The clinical features of COVID-19 can range from an asymptomatic state to acute respiratory syndrome and multiple organ dysfunction. Although some hematological and biochemical parameters are altered during moderate and severe COVID-19, there is still a lack of tools to combine these parameters to predict the clinical outcome of a patient with COVID-19. Thus, this study aimed at employing hematological and biochemical parameters of patients diagnosed with COVID-19 in order to build machine learning algorithms for predicting COVID mortality or survival. Patients included in the study had a diagnosis of SARS-CoV-2 infection confirmed by RT-PCR and biochemical and hematological measurements were performed in three different time points upon hospital admission. Among the parameters evaluated, the ones that stand out the most are the important features of the T1 time point (urea, lymphocytes, glucose, basophils and age), which could be possible biomarkers for the severity of COVID-19 patients. This study shows that urea is the parameter that best classifies patient severity and rises over time, making it a crucial analyte to be used in machine learning algorithms to predict patient outcome. In this study optimal and medically interpretable machine learning algorithms for outcome prediction are presented for each time point. It was found that urea is the most paramount variable for outcome prediction over all three time points. However, the order of importance of other variables changes for each time point, demonstrating the importance of a dynamic approach for an effective patient's outcome prediction. All in all, the use of machine learning algorithms can be a defining tool for laboratory monitoring and clinical outcome prediction, which may bring benefits to public health in future pandemics with newly emerging and reemerging SARS-CoV-2 variants of concern.
更多查看译文
关键词
SARS-CoV-2,COVID-19,hematological and biochemical parameters,predictive biomarkers,machine learning
AI 理解论文
溯源树
样例
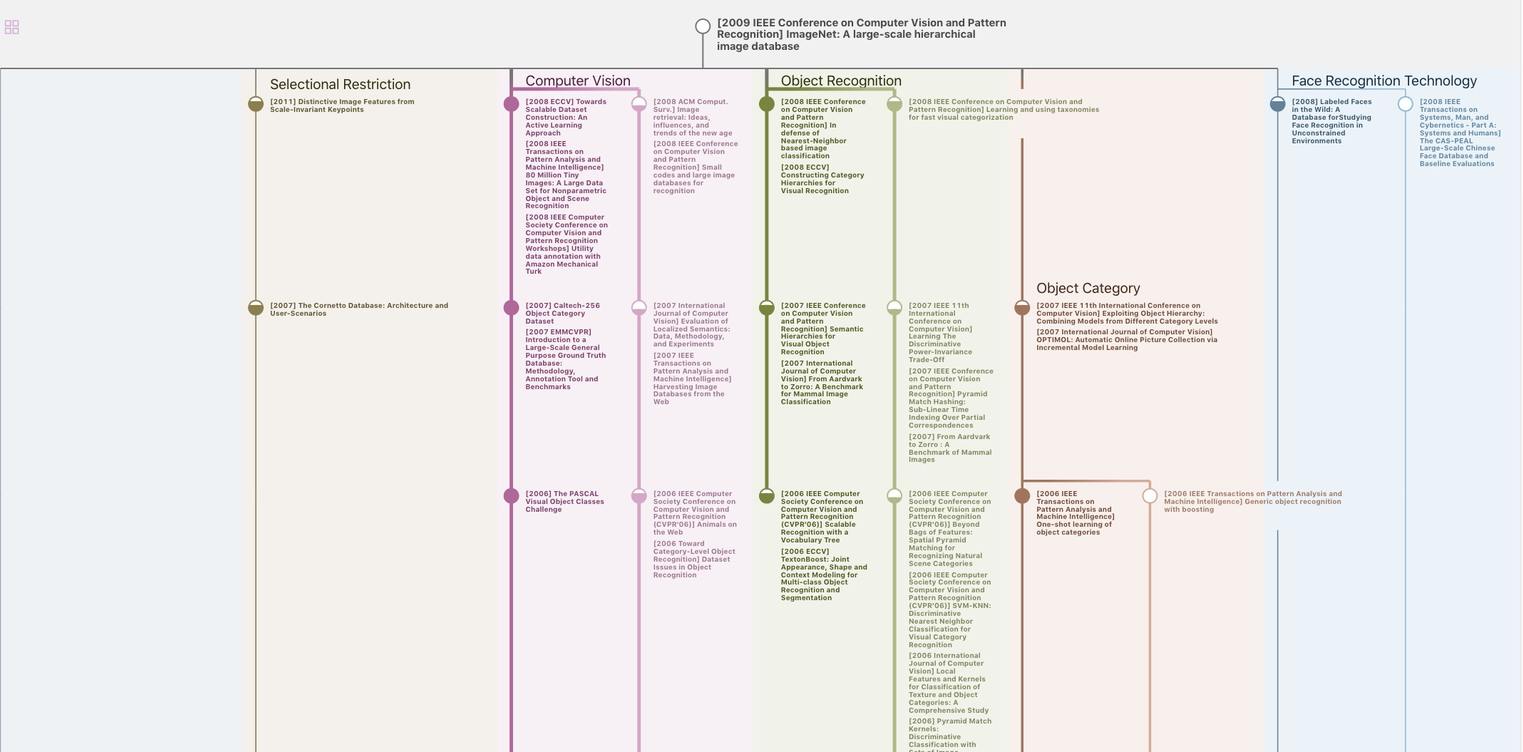
生成溯源树,研究论文发展脉络
Chat Paper
正在生成论文摘要