Predictive insights into nonlinear nanofluid flow in rotating systems: a machine learning approach
Engineering with Computers(2024)
Abstract
This research seeks to explore the heat shift mechanisms in a rotating system that contains a hybrid nanofluid comprising of graphene oxide and copper particles mixed with pure water, using a novel methodology. The fluid flow in a rotating system is described by mathematical equations that involve nonlinear partial differential equations (PDEs). These equations are simplified by using similarity transformations, resulting in a system of ordinary differential equations. In general, it is not feasible to find a closed-form analytical solution for nonlinear ordinary differential equations (ODEs), which implies that determining an exact mathematical expression that characterizes the behavior of the solution to such ODEs is often challenging or impossible. To that end, we have utilized the controlled learning procedure of machine learning algorithms to predict the solutions for the nonlinear nanofluid problem flowing in the rotating system. The surrogated model are developed for different cases and scenarios, to review the might of differences in various physical parameters on the profiles of the fluid. Furthermore, the solutions are supported by performing an extensive statistical analysis based on different errors. It is concluded that machine learning-based method can potentially provide insights into the underlying physics of nonlinear flow problems, which can aid in the progress of more advanced and accurate models for prognosticating the behavior of nonlinear systems.
MoreTranslated text
Key words
Hybrid nanofluid,Rotating system,Schmidt number,Machine learning,Data mining,Supervised neural networks
AI Read Science
Must-Reading Tree
Example
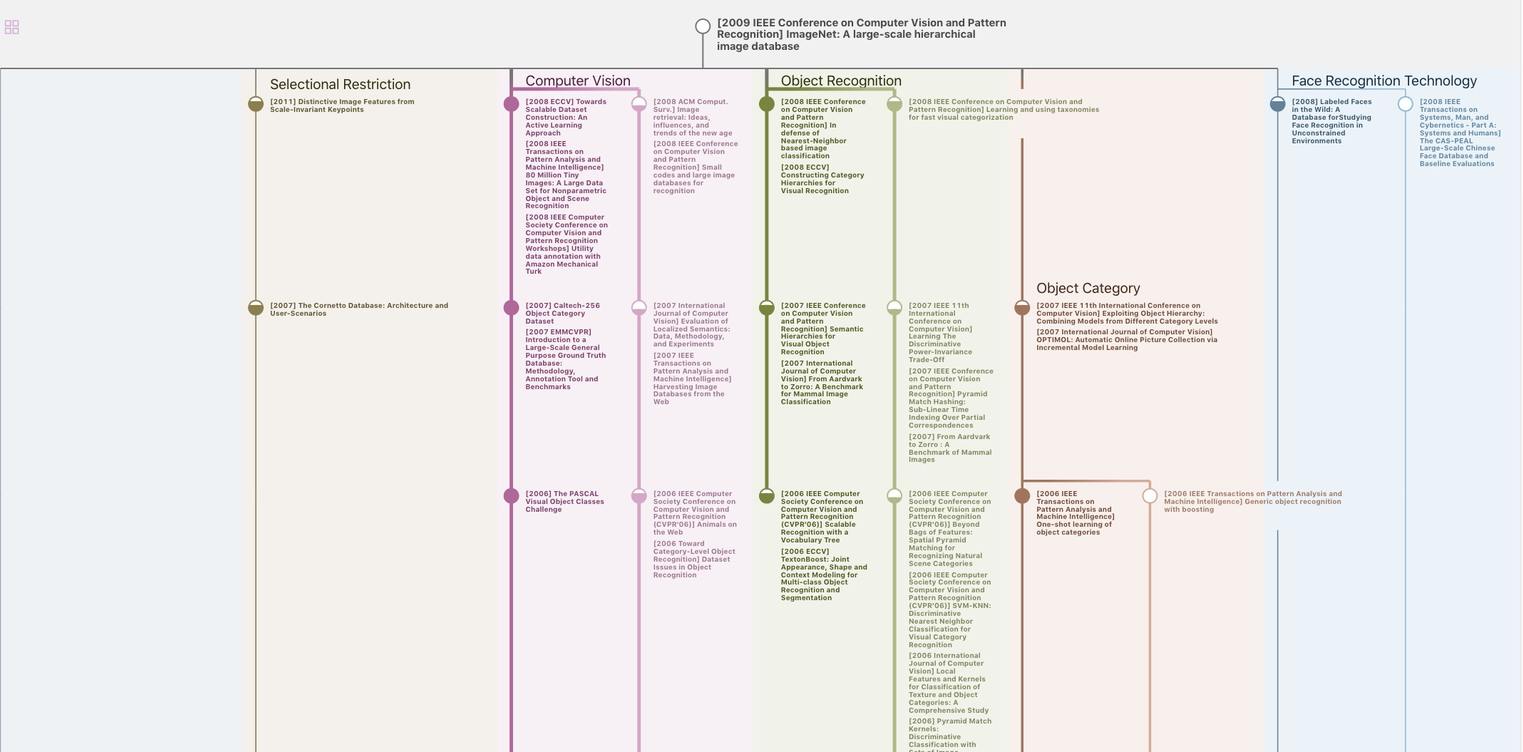
Generate MRT to find the research sequence of this paper
Chat Paper
Summary is being generated by the instructions you defined