Softness Prediction with a Soft Biomimetic Optical Tactile Sensor.
International Conference on Soft Robotics(2024)
Abstract
With the growing interest in vision-based tactile sensor technology for applications such as fruit harvesting based on ripeness, accurate object softness recognition has become increasingly important. In our study, we examined the capability of soft biomimetic optical tactile sensor, a TacTip with a flat sensing surface, for this task. By systematically pressing the TacTip against hardness-controlled silicone samples, we linked sequential TacTip tactile images of patterns of markers with the known Shore 00 hardness values of the samples. Trained on 1323 data points, the multichannel 2D CNN showed good accuracy across the entire Shore 00 hardness range. Yet, its performance diminished for hardness values above 70 during online tests. We interpret these differences in performance as due to the relative softness differential between the sensor's skin and the silicone samples.
MoreTranslated text
Key words
Tactile Sensor,Soft Sensor,Differences In Performance,Online Assessment,Elastography,Silicon Sample,Neural Network,Training Data,Young’s Modulus,Predictive Performance,R2 Values,Deep Neural Network,Mean Absolute Error,Long Short-term Memory,Robotic Arm,Total Image,Soft Robots,Mean Absolute Error Values,Temporal Range,Hardness Of Samples,Structural Similarity Index Measure,Hardness Levels,Average Mean Absolute Error,Hard Scale,Penetrometer,Simple Convolutional Neural Network,Convolutional Layers,Neural Network Training,Temporal Localization,Shore Hardness
AI Read Science
Must-Reading Tree
Example
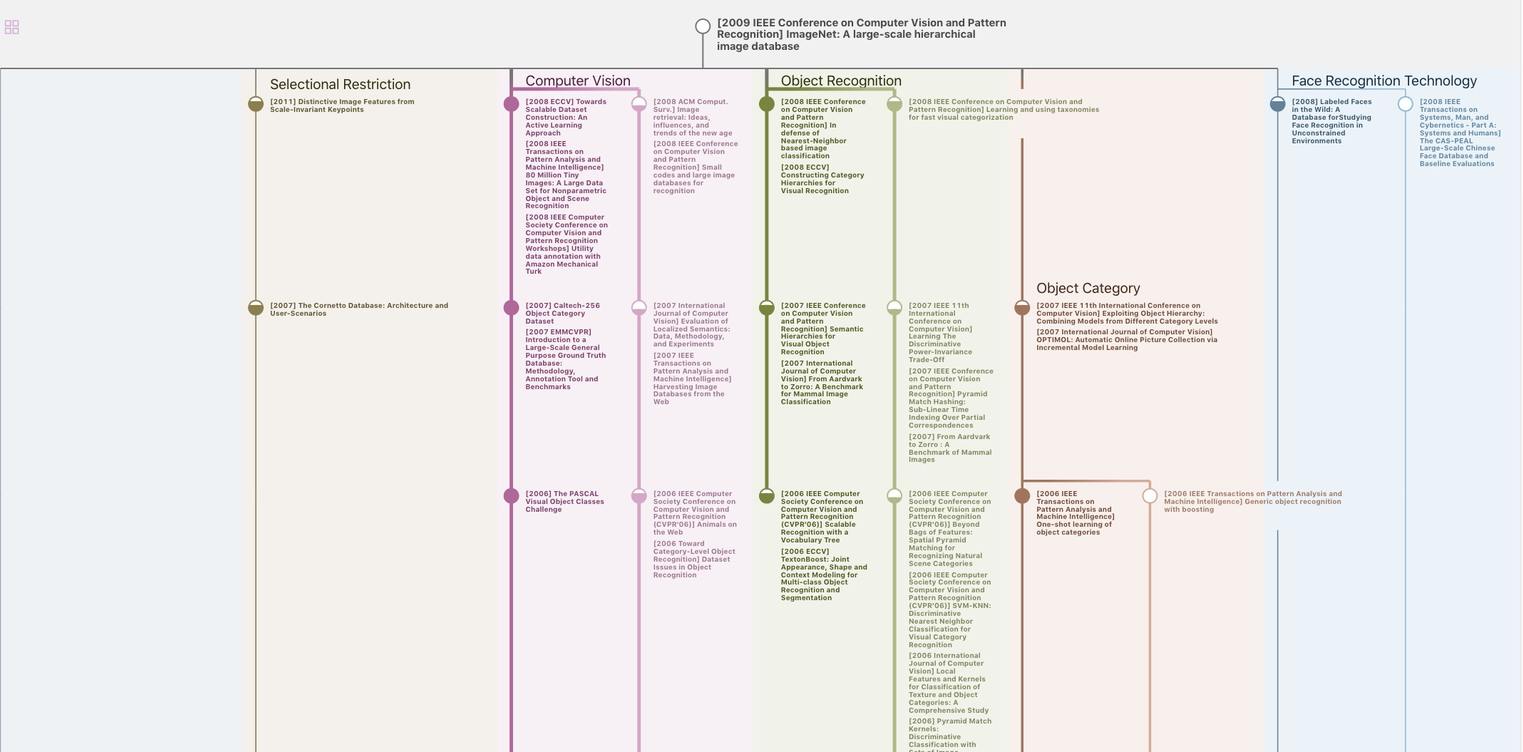
Generate MRT to find the research sequence of this paper
Chat Paper
Summary is being generated by the instructions you defined