Develop Machine Learning-Based Model and Automated Process for Predicting Liquid Heat Capacity of Organics at Different Temperatures
FLUID PHASE EQUILIBRIA(2024)
关键词
Machine learning,Heat capacity,Liquid,Thermodynamic properties,Molecular descriptor
AI 理解论文
溯源树
样例
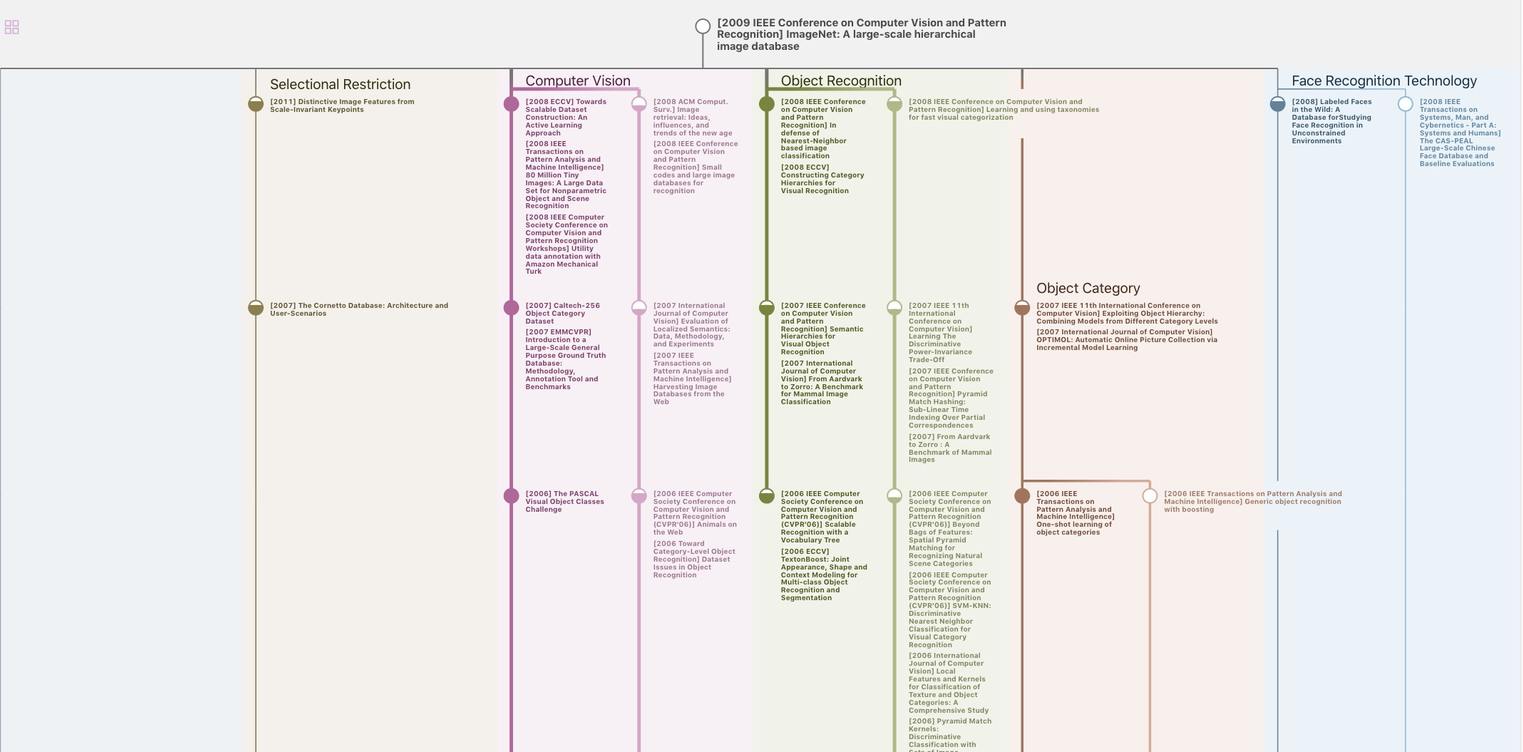
生成溯源树,研究论文发展脉络
Chat Paper
正在生成论文摘要