Multi-sensor fusion and machine learning-driven sequence-to-sequence translation for interpretable process signature prediction in machining
Journal of Manufacturing Systems(2024)
Abstract
During machining, kinetic energy is imparted to a workpiece to remove material. The integrity of the machined surface, which depends on the energy transfer, affects the quality and performance of the product, therefore needs to be quantified. Prior studies have indicated the potential of using machining power, or the power consumption at the tool-chip interface, as a process signature for predicting machined surface integrity. However, direct measurement of machining power is constrained by the availability of special equipment and the associated cost. To address this gap, this paper presents a machine learning-based method for machining power prediction through multi-sensor fusion and sequence-to-sequence translation from acoustic and vibration signals, which represent portions of the in-situ kinetic energy dissipation, to the machining power signal as a process signature. Specifically, a neural network architecture is developed to separately translate the acoustic and vibration signals to corresponding machining power signals. The two predicted power signals are subsequently fused to arrive at a unified power signal prediction. To check for spurious decision logic, the sensor fusion model is interpreted using integrated gradients to reveal temporal regions of the input data which have the most influence on the machining power prediction accuracy of the fusion model. Systematic cutting experiments performed on a lathe using 1018 steel have shown that the developed sensor fusion method for process signature prediction can successfully map machine acoustics to power consumption with 5.6% error, tool vibration to power consumption with 8.2% error, and acoustics and vibration, jointly, to power with 2.5% error. Model parameter interpretation reveals that the vibration signal is more influential on the machining power prediction result than the acoustic signal, but that overall model accuracy is diminished if only the vibration signal is used.
MoreTranslated text
Key words
Machining,Sensor fusion,Process signature,Interpretable machine learning,Acoustic signals
AI Read Science
Must-Reading Tree
Example
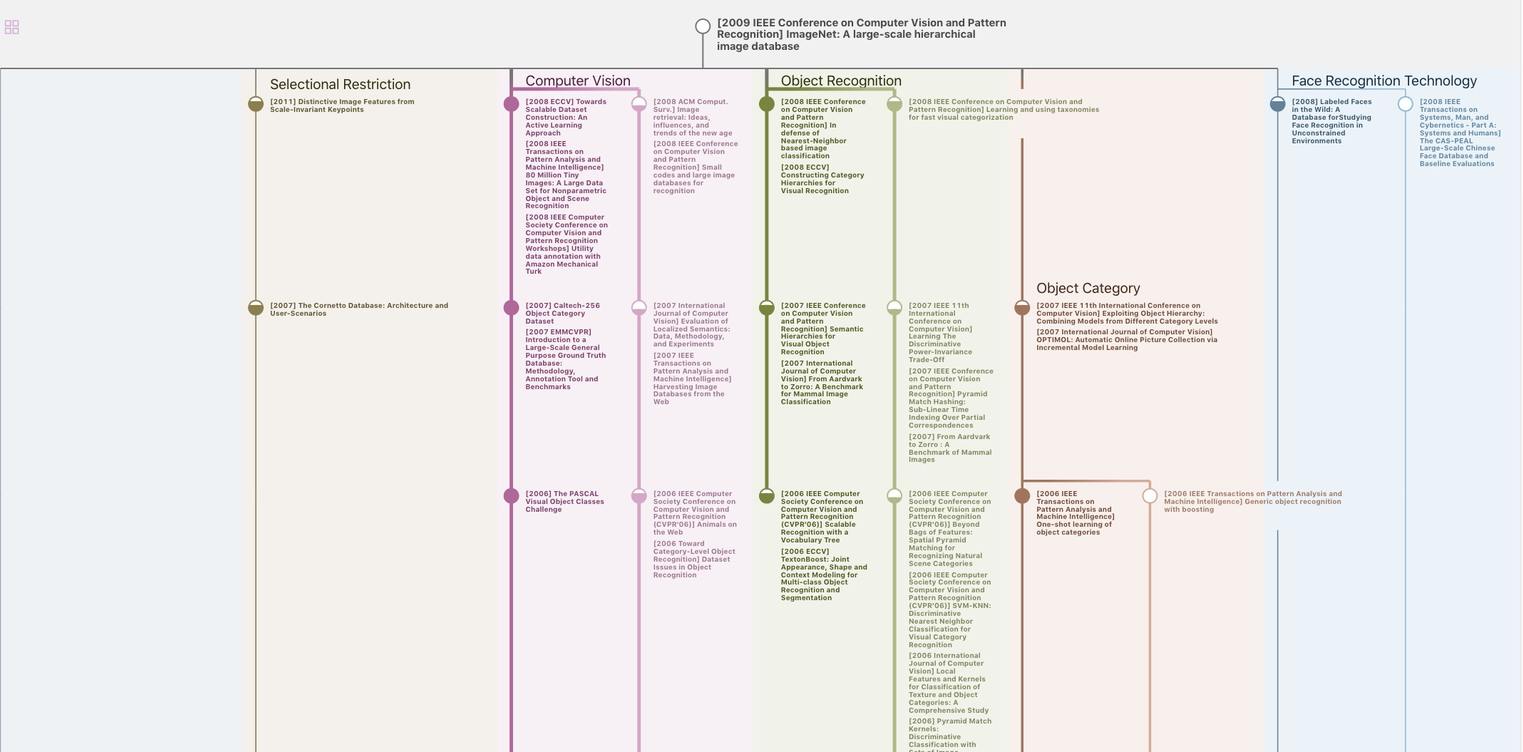
Generate MRT to find the research sequence of this paper
Chat Paper
Summary is being generated by the instructions you defined