Towards Subgraph Isomorphism Counting with Graph Kernels
CoRR(2024)
Abstract
Subgraph isomorphism counting is known as #P-complete and requires
exponential time to find the accurate solution. Utilizing representation
learning has been shown as a promising direction to represent substructures and
approximate the solution. Graph kernels that implicitly capture the
correlations among substructures in diverse graphs have exhibited great
discriminative power in graph classification, so we pioneeringly investigate
their potential in counting subgraph isomorphisms and further explore the
augmentation of kernel capability through various variants, including
polynomial and Gaussian kernels. Through comprehensive analysis, we enhance the
graph kernels by incorporating neighborhood information. Finally, we present
the results of extensive experiments to demonstrate the effectiveness of the
enhanced graph kernels and discuss promising directions for future research.
MoreTranslated text
AI Read Science
Must-Reading Tree
Example
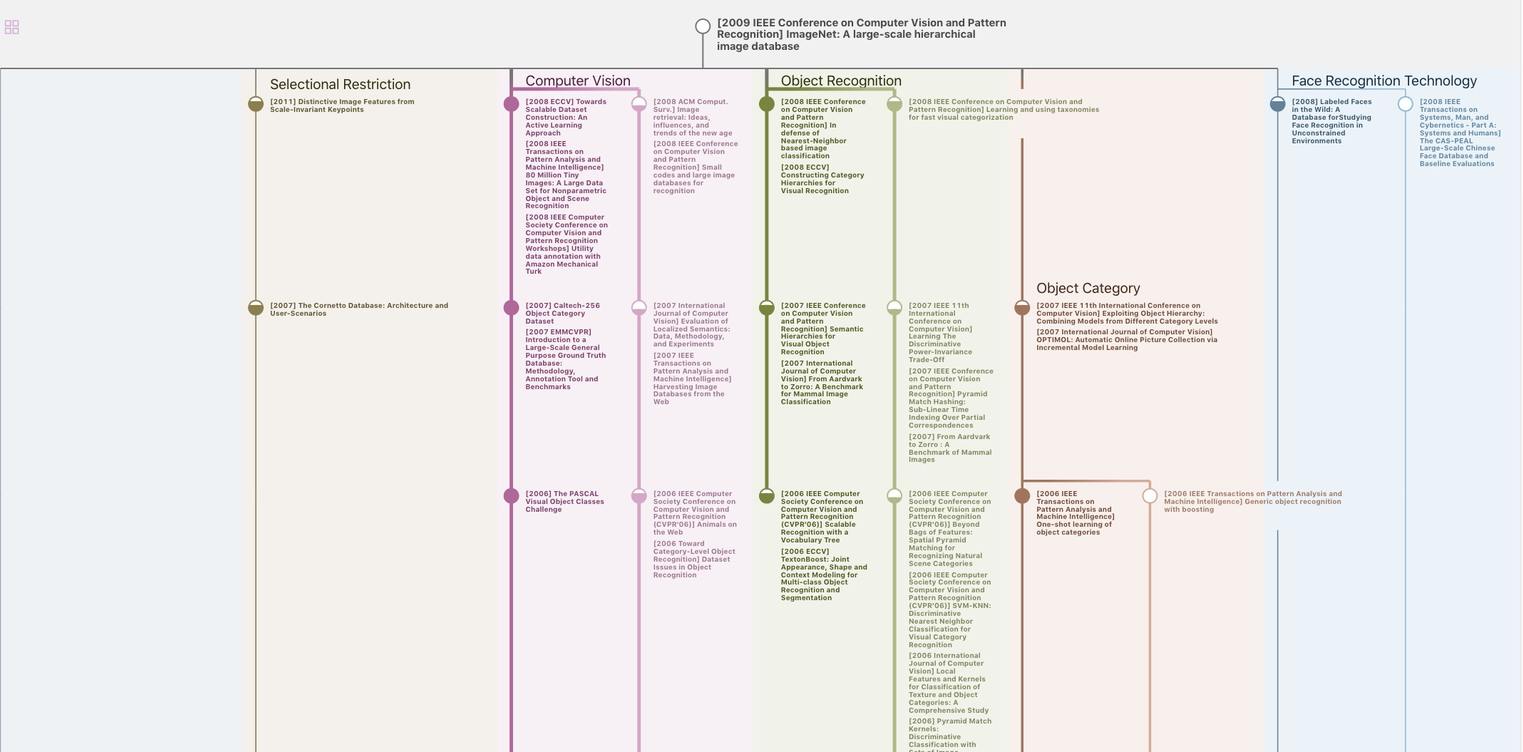
Generate MRT to find the research sequence of this paper
Chat Paper
Summary is being generated by the instructions you defined