ERAGent: Enhancing Retrieval-Augmented Language Models with Improved Accuracy, Efficiency, and Personalization
CoRR(2024)
Abstract
Retrieval-augmented generation (RAG) for language models significantly
improves language understanding systems. The basic retrieval-then-read pipeline
of response generation has evolved into a more extended process due to the
integration of various components, sometimes even forming loop structures.
Despite its advancements in improving response accuracy, challenges like poor
retrieval quality for complex questions that require the search of multifaceted
semantic information, inefficiencies in knowledge re-retrieval during long-term
serving, and lack of personalized responses persist. Motivated by transcending
these limitations, we introduce ERAGent, a cutting-edge framework that embodies
an advancement in the RAG area. Our contribution is the introduction of the
synergistically operated module: Enhanced Question Rewriter and Knowledge
Filter, for better retrieval quality. Retrieval Trigger is incorporated to
curtail extraneous external knowledge retrieval without sacrificing response
quality. ERAGent also personalizes responses by incorporating a learned user
profile. The efficiency and personalization characteristics of ERAGent are
supported by the Experiential Learner module which makes the AI assistant being
capable of expanding its knowledge and modeling user profile incrementally.
Rigorous evaluations across six datasets and three question-answering tasks
prove ERAGent's superior accuracy, efficiency, and personalization, emphasizing
its potential to advance the RAG field and its applicability in practical
systems.
MoreTranslated text
AI Read Science
Must-Reading Tree
Example
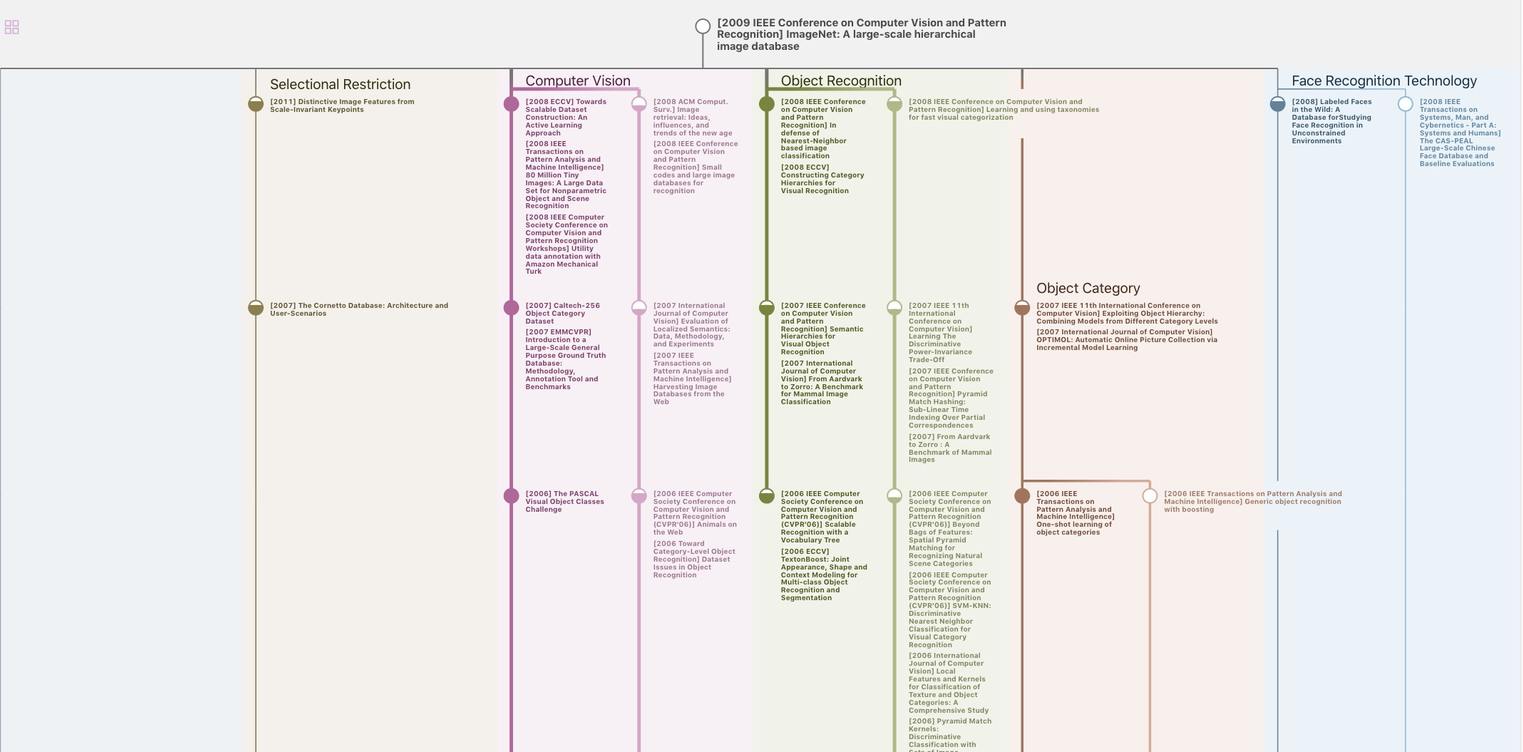
Generate MRT to find the research sequence of this paper
Chat Paper
Summary is being generated by the instructions you defined