An underwater target recognition algorithm incorporating improved attention mechanism and downsampling
The Visual Computer(2024)
Abstract
To address the issue of low accuracy in recognizing underwater targets due to dense and blurred targets in underwater target detection, we propose a joint improved attention mechanism and downsampling network for underwater target detection. Firstly, to address the issue of dense targets, we introduce an improved channel attention module. This module enhances attention to spatial dimension information, highlights the saliency of feature maps of different channels and improves the detection ability of dense targets. Secondly, to address the issue of blurred underwater targets, we introduce a down-sampling module that combines same-layer connections and cross-layer skipping. This module reduces information loss caused by convolutional down-sampling and integrates features from different layers more fully. By improving the feature expression of the underwater image, the network’s detection accuracy for underwater blurred targets is further enhanced. Finally, the study introduces the focus loss function to address the imbalance of positive and negative samples. This function dynamically reduces the weight of easy-to-distinguish samples during training and prioritizes difficult-to-distinguish samples. Experimental results demonstrate a 2.71 https://figshare.com/articles/dataset/improved-yolov5/25375129 . Dataset: https://figshare.com/articles/dataset/DUO_zip/25370527 .
MoreTranslated text
Key words
Underwater target detection,Channel attention,Feature fusion,Focal loss function
AI Read Science
Must-Reading Tree
Example
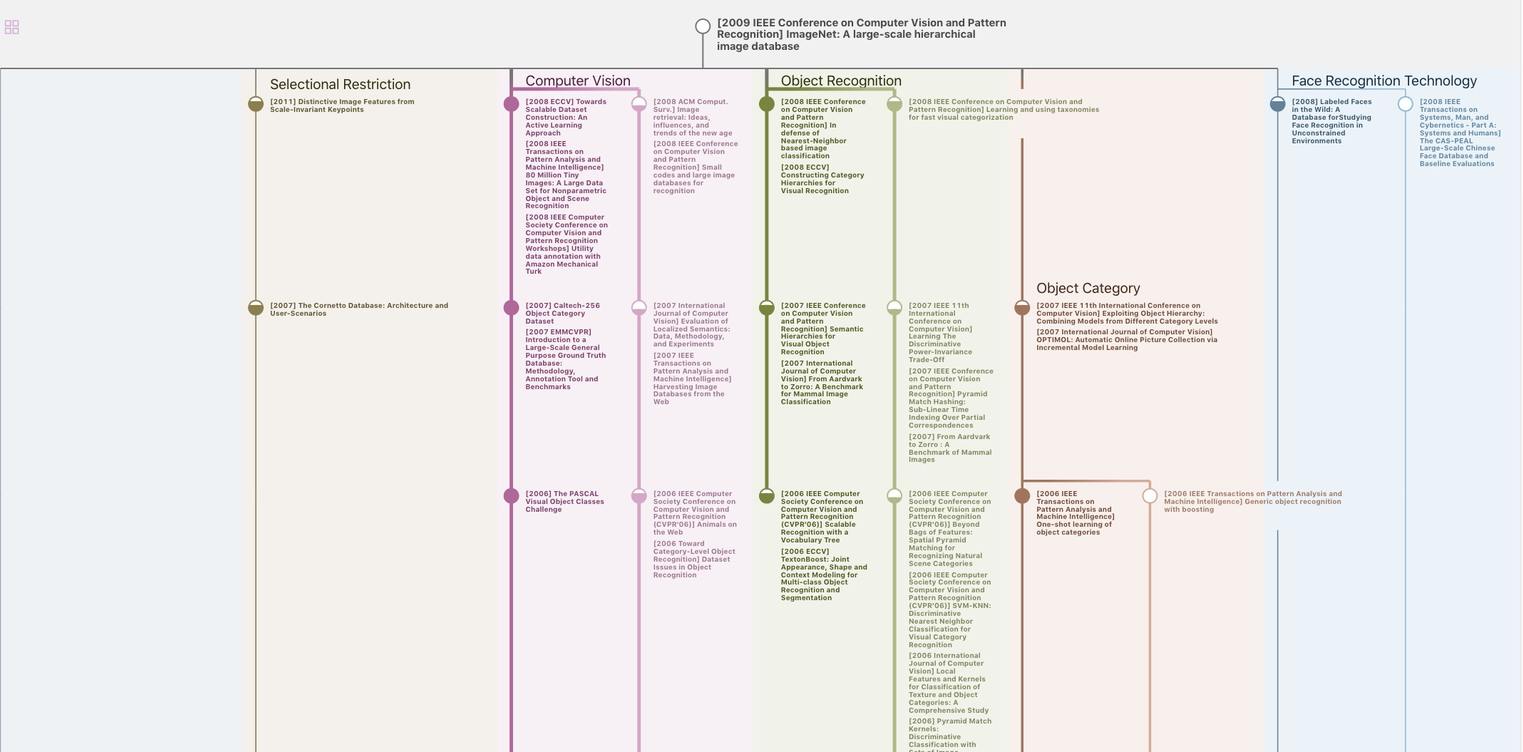
Generate MRT to find the research sequence of this paper
Chat Paper
Summary is being generated by the instructions you defined