Deep learning based automatic seizure prediction with EEG time-frequency representation
Biomedical Signal Processing and Control(2024)
摘要
Automatic seizure prediction is crucial for developing a new therapy for patients suffering from medically intractable epilepsy, possessing important clinical application value. In order to solve the problems of overfitting due to the complexity of deep learning based seizure prediction networks and the susceptibility of EEG features to noise contamination, an automatic seizure prediction model based on Stockwell Transform (S-transform) and Multi-Channel Vision Transformer (MViT) is proposed in this work. The time-frequency representation of multi-channel electroencephalography (EEG) signals is obtained by using S-Transform. These time-frequency spectrograms are then compressed and sent into the MViT model for further spatial feature extraction and identification of preictal EEG state. The designed MViT model is lightweight, ensuring efficient feature discriminating ability even with a minimal number of network layers. In view of the persistence of EEG activities, K-of-N strategy is employed to further augment the predictive performance. Experiments on the CHB-MIT database and the SH-SDU clinical database yield segment-based accuracy of 97.57 % and 95.88 % respectively, demonstrating the superiority and potential of the proposed method in seizure prediction.
更多查看译文
关键词
Electroencephalography (EEG),Seizure prediction,Vision transformer,Stockwell transform
AI 理解论文
溯源树
样例
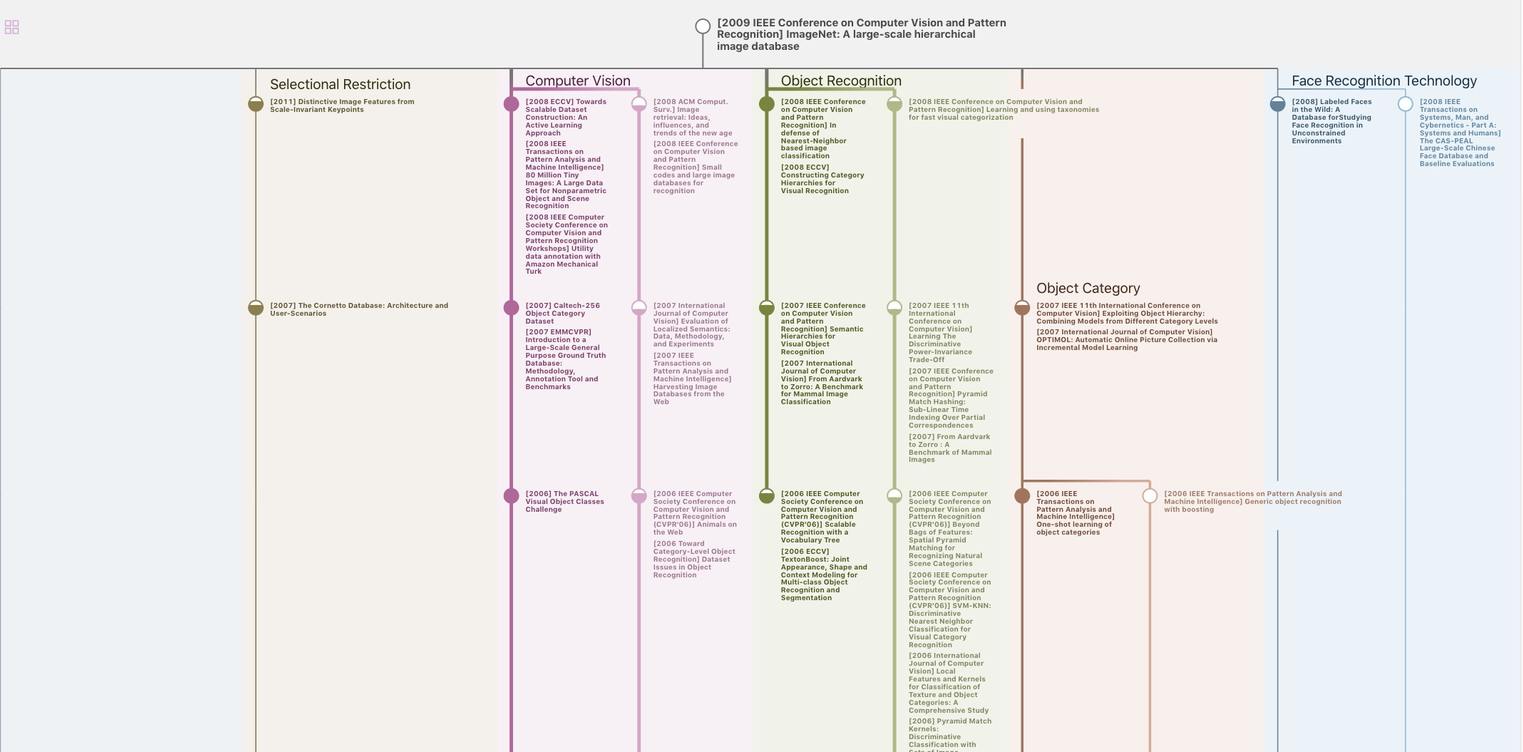
生成溯源树,研究论文发展脉络
Chat Paper
正在生成论文摘要