Semantic and Spatial Adaptive Pixel-level Classifier for Semantic Segmentation
CoRR(2024)
摘要
Vanilla pixel-level classifiers for semantic segmentation are based on a
certain paradigm, involving the inner product of fixed prototypes obtained from
the training set and pixel features in the test image. This approach, however,
encounters significant limitations, i.e., feature deviation in the semantic
domain and information loss in the spatial domain. The former struggles with
large intra-class variance among pixel features from different images, while
the latter fails to utilize the structured information of semantic objects
effectively. This leads to blurred mask boundaries as well as a deficiency of
fine-grained recognition capability. In this paper, we propose a novel Semantic
and Spatial Adaptive (SSA) classifier to address the above challenges.
Specifically, we employ the coarse masks obtained from the fixed prototypes as
a guide to adjust the fixed prototype towards the center of the semantic and
spatial domains in the test image. The adapted prototypes in semantic and
spatial domains are then simultaneously considered to accomplish classification
decisions. In addition, we propose an online multi-domain distillation learning
strategy to improve the adaption process. Experimental results on three
publicly available benchmarks show that the proposed SSA significantly improves
the segmentation performance of the baseline models with only a minimal
increase in computational cost. Code is available at
https://github.com/xwmaxwma/SSA.
更多查看译文
AI 理解论文
溯源树
样例
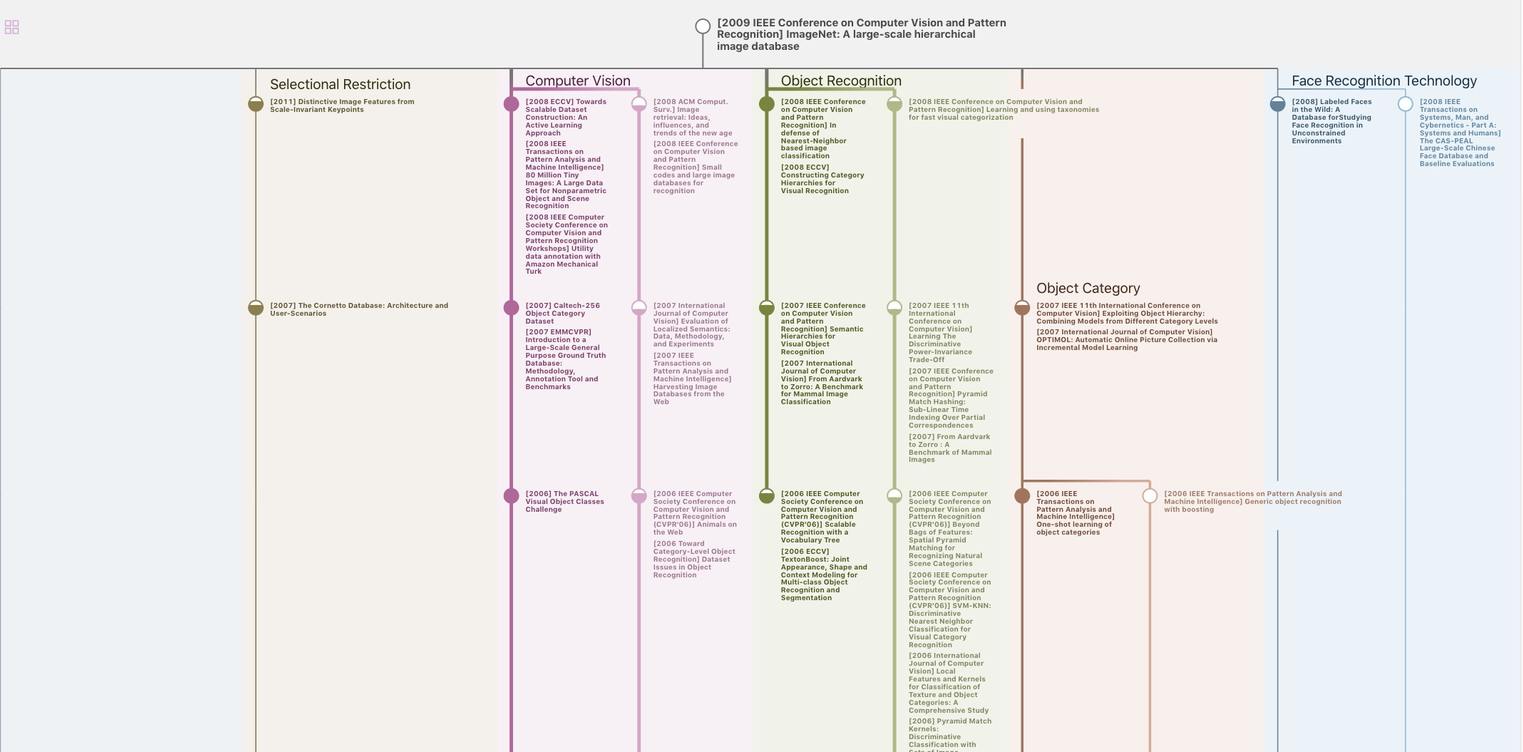
生成溯源树,研究论文发展脉络
Chat Paper
正在生成论文摘要