A Case Study of Enhancing Sparse Retrieval using LLMs.
The Web Conference(2024)
摘要
While dense retrieval methods have made significant advancements, sparse retrieval techniques continue to offer advantages in terms of interpretability and generalizability. However, query-document term mismatch in sparse retrieval persists, rendering it infeasible for many practical applications. Recent research has shown that Large Language Models (LLMs) hold relevant information that can enhance sparse retrieval through the application of prompt engineering. In this paper, we build upon this concept to explore various strategies employing LLMs for information retrieval purposes. Specifically, we utilize LLMs to enhance sparse retrieval by query rewriting and query expansion. In query rewriting, the original query is refined by creating several new queries. For query expansion, LLMs are employed to generate extra terms, thereby enriching the original query. We conduct experiments on a range of well-known information retrieval datasets, including MSMARCO-passage, TREC2019, TREC2020, Natural Questions, SCIFACT. The experiments show that LLMs can be beneficial for sparse methods since the added information provided by the LLMs can help diminish the discrepancy between the term frequencies of the important terms in a query and the relevant document. In certain domains, we demonstrate that the effectiveness of LLMs is constrained, indicating that they may not consistently perform optimally, which will be explored in future research.
更多查看译文
AI 理解论文
溯源树
样例
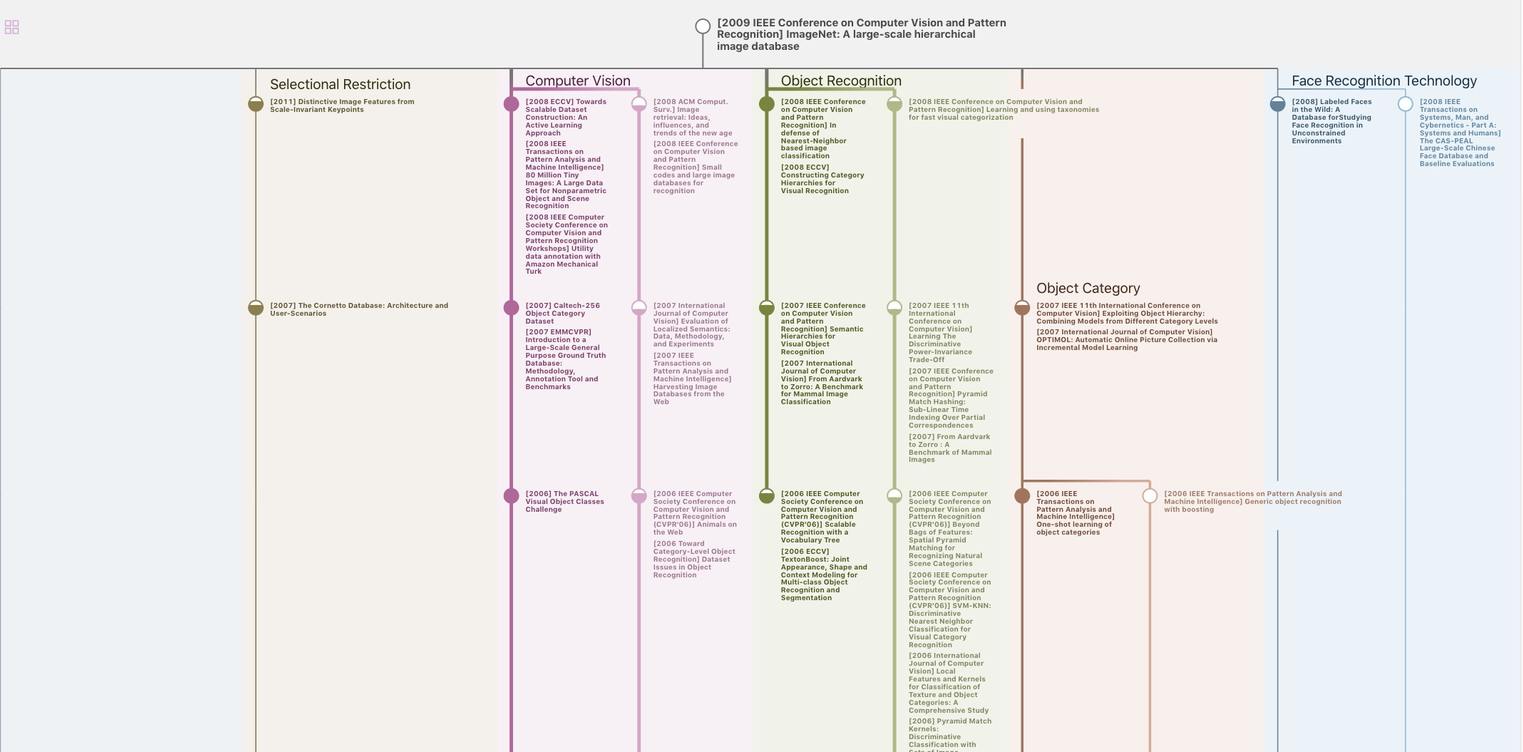
生成溯源树,研究论文发展脉络
Chat Paper
正在生成论文摘要