IMPRL-Net: interpretable multi-view proximity representation learning network
Neural Computing and Applications(2024)
摘要
Due to the heterogeneity gap in multi-view data, researchers have been attempting to apply these data to learn a co-latent representation to bridge this gap. However, multi-view representation learning still confronts two challenges: (1) it is hard to simultaneously consider the performance of downstream tasks and the interpretability and transparency of the network; (2) it fails to learn representations that accurately describe the class boundaries of downstream tasks. To overcome these limitations, we propose an interpretable representation learning framework, named interpretable multi-view proximity representation learning network. On the one hand, the proposed network is customized by an explicitly designed optimization objective that enables it to learn semantic co-latent representations while maintaining the interpretability and transparency of the network from the design level. On the other hand, the designed multi-view proximity representation learning objective function encourages its learned co-latent representations to form intuitive class boundaries by increasing the inter-class distance and decreasing the intra-class distance. Driven by a flexible downstream task loss, the learned co-latent representation can adapt to various multi-view scenarios and has been shown to be effective in experiments. As a result, this work provides a feasible solution to a generalized multi-view representation learning framework and is expected to accelerate the research and exploration in this field.
更多查看译文
关键词
Multi-view learning,Deep learning,Representation learning,Proximity learning
AI 理解论文
溯源树
样例
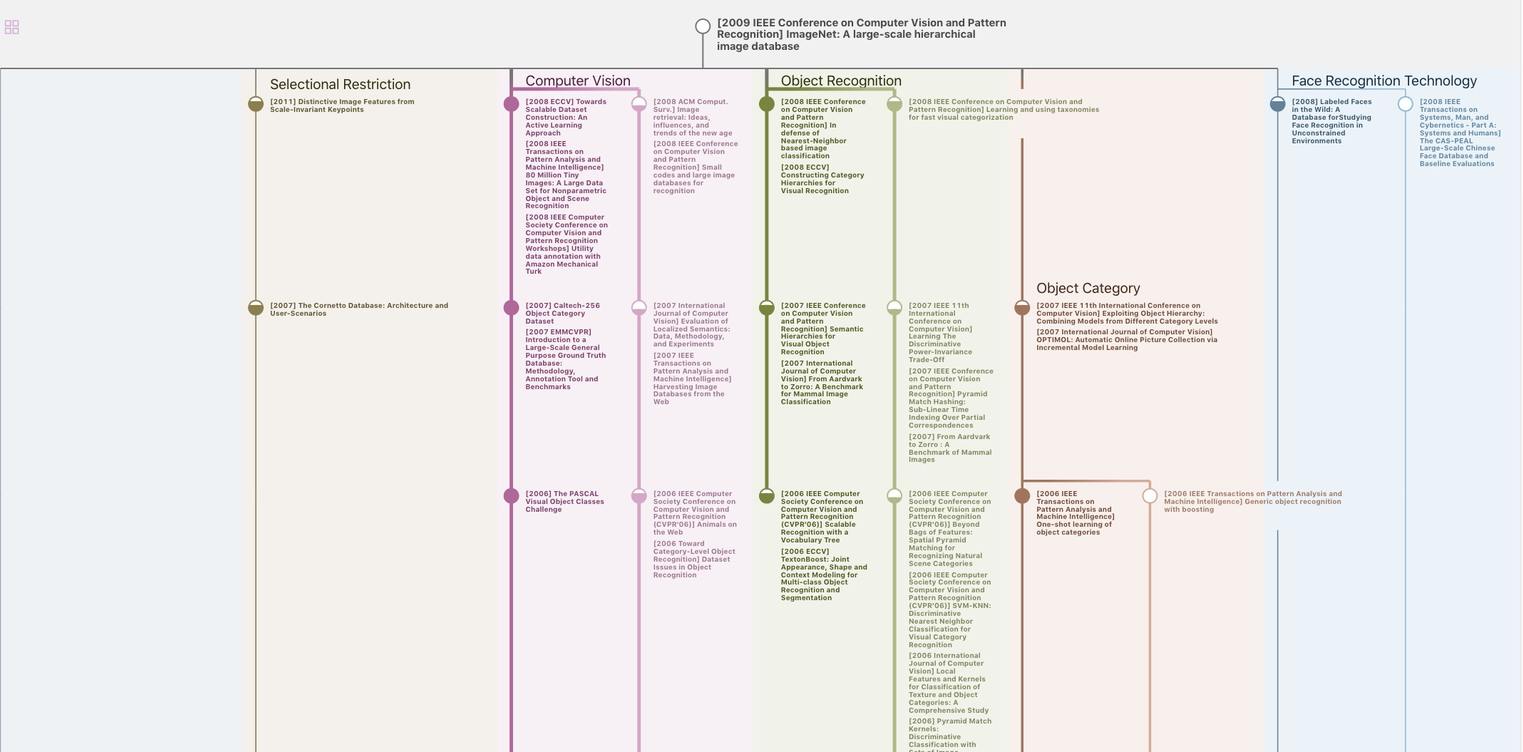
生成溯源树,研究论文发展脉络
Chat Paper
正在生成论文摘要