Understanding Choice Independence and Error Types in Human-AI Collaboration.
ACM Conference on Human Factors in Computing Systems(2024)
摘要
The ability to make appropriate delegation decisions is an important prerequisite of effective human-AI collaboration. Recent work, however, has shown that people struggle to evaluate AI systems in the presence of forecasting errors, falling well short of relying on AI systems appropriately. We use a pre-registered crowdsourcing study (N = 611) to extend this literature by two underexplored crucial features of human AI decision-making: choice independence and error type. Subjects in our study repeatedly complete two prediction tasks and choose which predictions they want to delegate to an AI system. For one task, subjects receive a decision heuristic that allows them to make informed and relatively accurate predictions. The second task is substantially harder to solve, and subjects must come up with their own decision rule. We systematically vary the AI system’s performance such that it either provides the best possible prediction for both tasks or only for one of the two. Our results demonstrate that people systematically violate choice independence by taking the AI’s performance in an unrelated second task into account. Humans who delegate predictions to a superior AI in their own expertise domain significantly reduce appropriate reliance when the model makes systematic errors in a complementary expertise domain. In contrast, humans who delegate predictions to a superior AI in a complementary expertise domain significantly increase appropriate reliance when the model systematically errs in the human expertise domain. Furthermore, we show that humans differentiate between error types and that this effect is conditional on the considered expertise domain. This is the first empirical exploration of choice independence and error types in the context of human-AI collaboration. Our results have broad and important implications for the future design, deployment, and appropriate application of AI systems.
更多查看译文
AI 理解论文
溯源树
样例
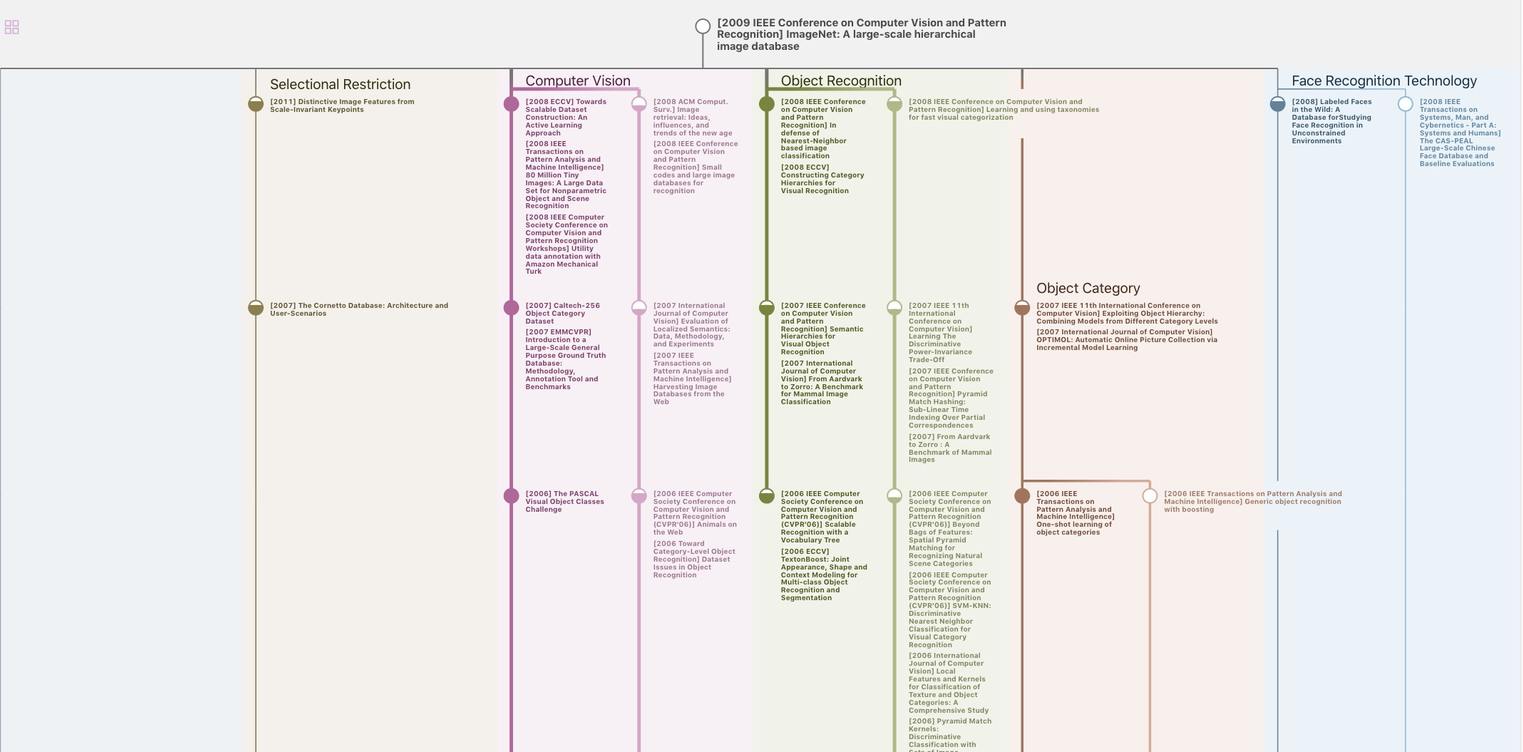
生成溯源树,研究论文发展脉络
Chat Paper
正在生成论文摘要